A Triplet Semi-supervised Deep Network for Fusion Classification of Hyperspectral and LiDAR Data
IEEE Transactions on Geoscience and Remote Sensing(2022)
摘要
Data fusion of hyperspectral and light detection and ranging (LiDAR) is conducive to obtain more comprehensive surface information and thereby achieve better classification result in Earth monitoring systems. However, lack of labeled samples usually limits the performance of supervised classifiers, and the heterogeneity of multisource data also brings great challenges to data fusion. Aiming to address these issues, we propose a triplet semisupervised deep network (TSDN) for fusion classification of hyperspectral and LiDAR. Specifically, we utilize three basic pathways to extract deep learning features: 1-D convolutional neural network (CNN) for spectral features in hyperspectral, 2-D CNN for spatial features in hyperspectral, and Cascade Net for elevation features in LiDAR data. Furthermore, a novel label calibration module (LCM) is proposed to generate effective pseudo labels with high confidence based on the superpixel segmentation by comparing the multiview classification results for assisting semisupervised model training. In addition, we design a novel 3D-Cross Attention Block to enhance the complementary spatial features of multisource data. Experiments on three public HSI-LiDAR benchmarks, Houston, Trento, and MUUFL Gulfport, have demonstrated the effectiveness and superiority of our proposed method.
更多查看译文
关键词
Classification,data fusion,deep learning,hyperspectral image,semisupervised
AI 理解论文
溯源树
样例
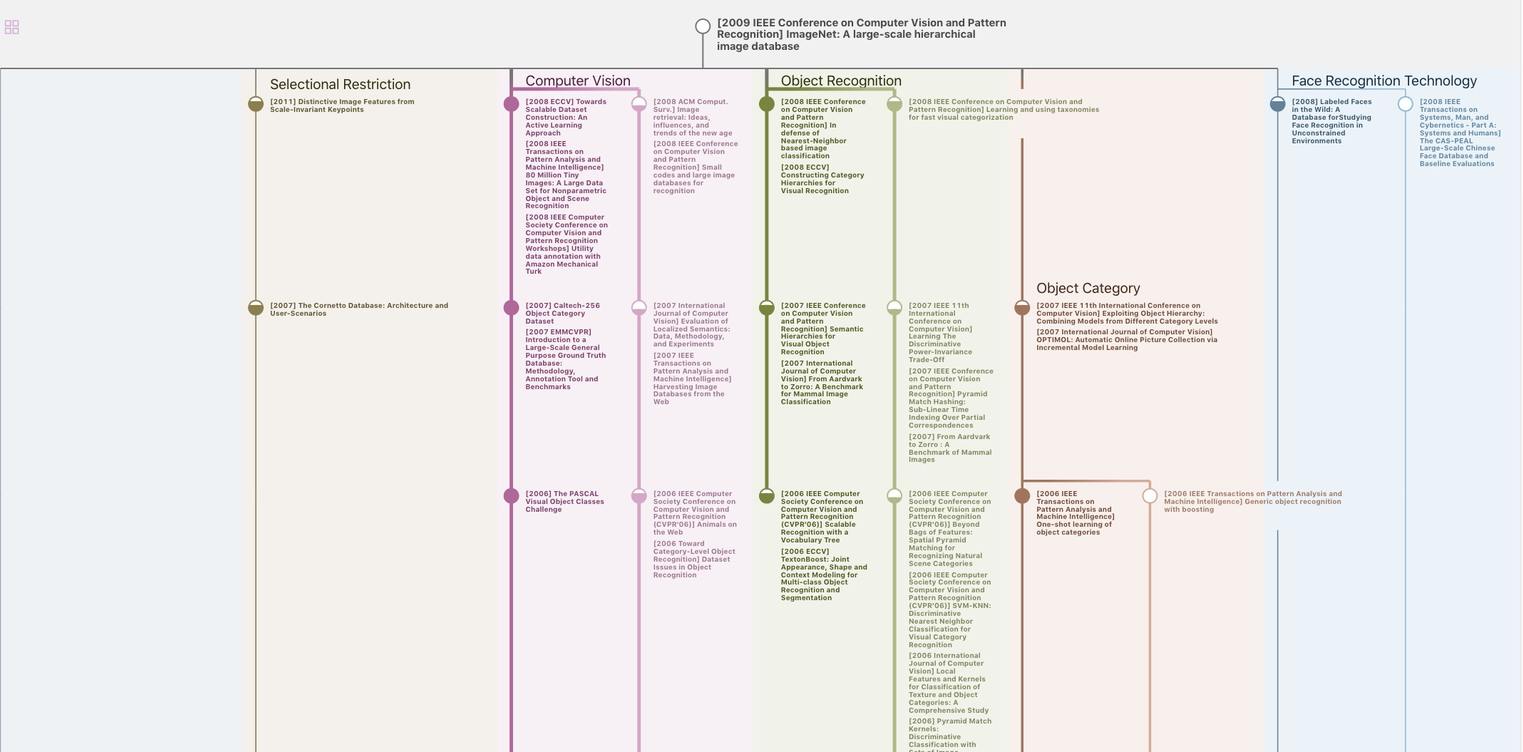
生成溯源树,研究论文发展脉络
Chat Paper
正在生成论文摘要