Use of modeling to map potentially toxic elements and assess the risk to human health in soils affected by mining activity
CATENA(2023)
摘要
Determining the levels and spatial distribution of potentially toxic elements (PTEs) is essential in designing mitigating strategies to remediate contaminated areas. Efficient prediction of PTEs is difficult due to high spatial variability and multiple sources of contamination. The use of machine learning algorithms is an alternative for the improvement of the predictions. Objective of the study was to model the spatial distribution of PTEs in soils surrounding a tailings pile and to assess potential risks to human health. A total set of 116 soil samples was collected to a depth of 0-10 cm. The covariate database was composed of morphometric variables derived from a DEM and the creation of a tailings pile location covariate. The models tested were Cubist (CUB), Random Forest (RF), Support Vector Machine (SVM), and Linear Model (Lm). Based on the best predictive model, maps showing the spatial distribution, pollution and risk to human health were created. The SVM model had the best perfor-mance for predicting spatially the soil contents of Pb and Zn, with lower values of mean absolute error (MAE -744.71; 306.36) and higher values of R2 (0.56; 0.52). It was possible to verify that the mining tailings pile is the main source of Pb and Zn contamination in the study area. The enrichment factor (EF) and the geoaccumulation index (Igeo) of Pb and Zn show that the soils close to the tailings piles are extremely contaminated (Igeo > 5 and EF > 40), and pose risks to human health (Total carcinogenic risk > 1 x 10-6), especially for children. Therefore, new studies must be carried out with the aim of monitoring and rehabilitating the contaminated areas.
更多查看译文
关键词
Chemical analysis,Lead ores,Pollution,Soils
AI 理解论文
溯源树
样例
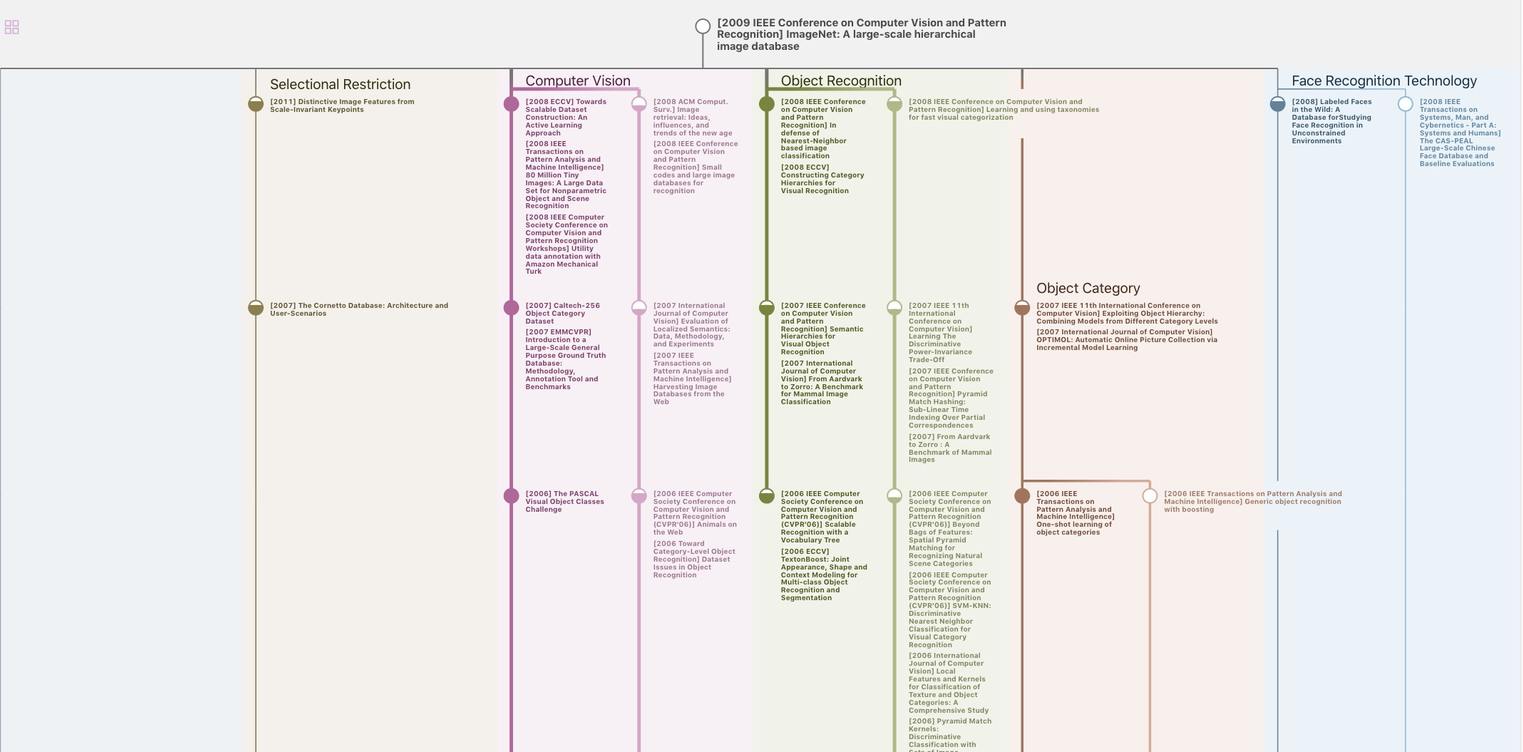
生成溯源树,研究论文发展脉络
Chat Paper
正在生成论文摘要