Improving indoor air flow and temperature prediction with local measurements based on CFD-EnKF data assimilation
Building and Environment(2022)
摘要
Mastering detailed multiphysics distribution information is an important prerequisite for creating suitable building environments. This study considered the simulation errors caused by the uncertainty of boundary conditions in computational fluid dynamics (CFD) and aimed to correct simulations with limited observational data. Based on the Ensemble Kalman Filter (EnKF), which is a sequential data assimilation algorithm, a technical framework for accurate indoor airflow and temperature field simulations was established. We evaluated the performance of this method through reduced-scale model experiments and verifying that simulation errors were significantly reduced, and that this approach is applicable to both mechanical ventilation and natural convection conditions. On this basis, further research explored the impact of the measuring point arrangement scheme and ensemble size on assimilation performance, and the optimal setting principles of the above parameters are presented in this paper. The proposed method can effectively weaken the negative impact caused by the uncertainty of boundary conditions in the CFD simulation, thereby improving the prediction accuracy and reliability and providing a positive impetus for realizing a global monitoring of the physical field of a building space.
更多查看译文
关键词
Data assimilation,Ensemble kalman filter,Indoor environment,CFD simulation,Boundary condition,Measurement
AI 理解论文
溯源树
样例
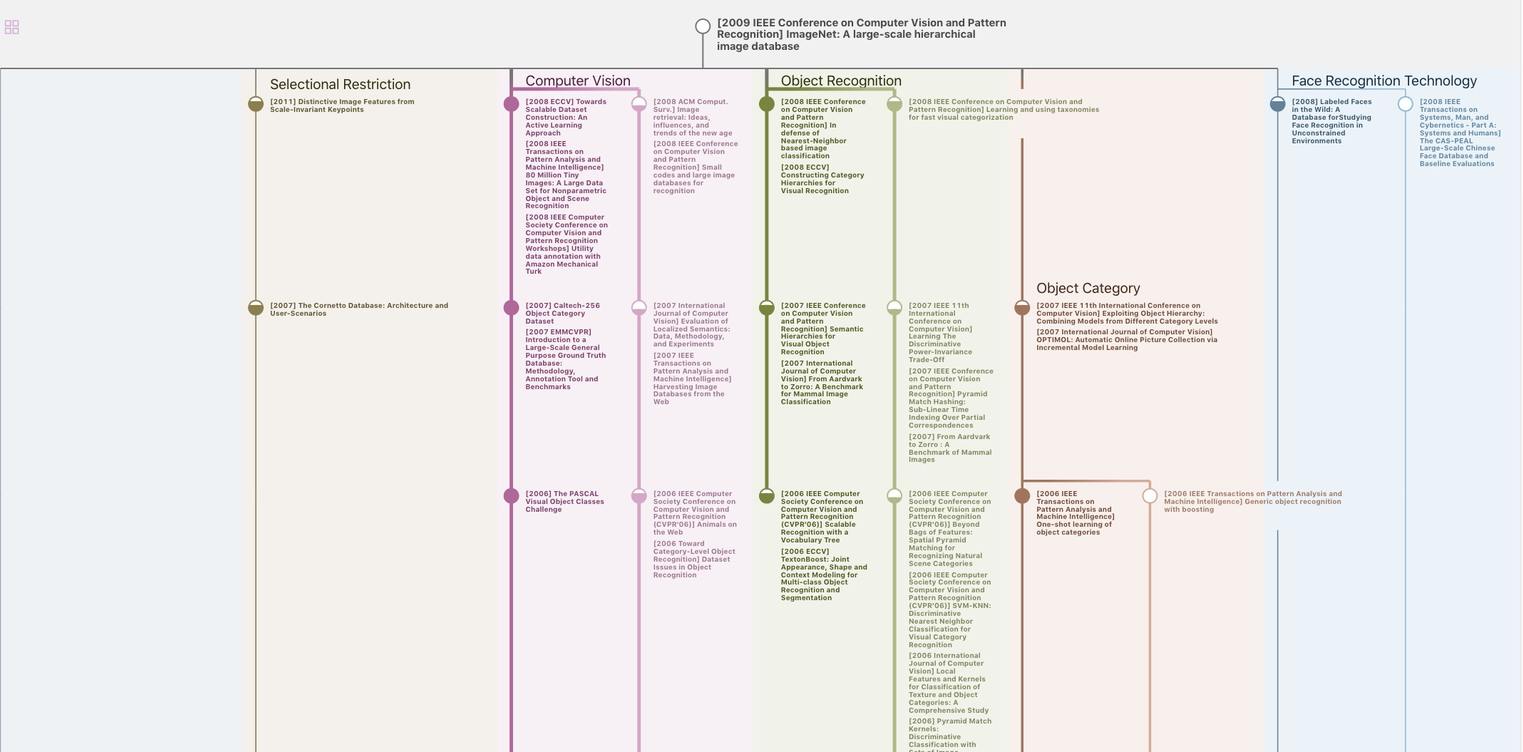
生成溯源树,研究论文发展脉络
Chat Paper
正在生成论文摘要