Comparison of logistic regression and machine learning methods for predicting postoperative delirium in elderly patients: A retrospective study
CNS NEUROSCIENCE & THERAPEUTICS(2022)
摘要
Aims To compare the performance of logistic regression and machine learning methods in predicting postoperative delirium (POD) in elderly patients. Method This was a retrospective study of perioperative medical data from patients undergoing non-cardiac and non-neurology surgery over 65 years old from January 2014 to August 2019. Forty-six perioperative variables were used to predict POD. A traditional logistic regression and five machine learning models (Random Forest, GBM, AdaBoost, XGBoost, and a stacking ensemble model) were compared by the area under the receiver operating characteristic curve (AUC-ROC), sensitivity, specificity, and precision. Results In total, 29,756 patients were enrolled, and the incidence of POD was 3.22% after variable screening. AUCs were 0.783 (0.765-0.8) for the logistic regression method, 0.78 for random forest, 0.76 for GBM, 0.74 for AdaBoost, 0.73 for XGBoost, and 0.77 for the stacking ensemble model. The respective sensitivities for the 6 aforementioned models were 74.2%, 72.2%, 76.8%, 63.6%, 71.6%, and 67.4%. The respective specificities for the 6 aforementioned models were 70.7%, 99.8%, 96.5%, 98.8%, 96.5%, and 96.1%. The respective precision values for the 6 aforementioned models were 7.8%, 52.3%, 55.6%, 57%, 54.5%, and 56.4%. Conclusions The optimal application of the logistic regression model could provide quick and convenient POD risk identification to help improve the perioperative management of surgical patients because of its better sensitivity, fewer variables, and easier interpretability than the machine learning model.
更多查看译文
关键词
aged,delirium,machine learning,nomograms,risk assessment
AI 理解论文
溯源树
样例
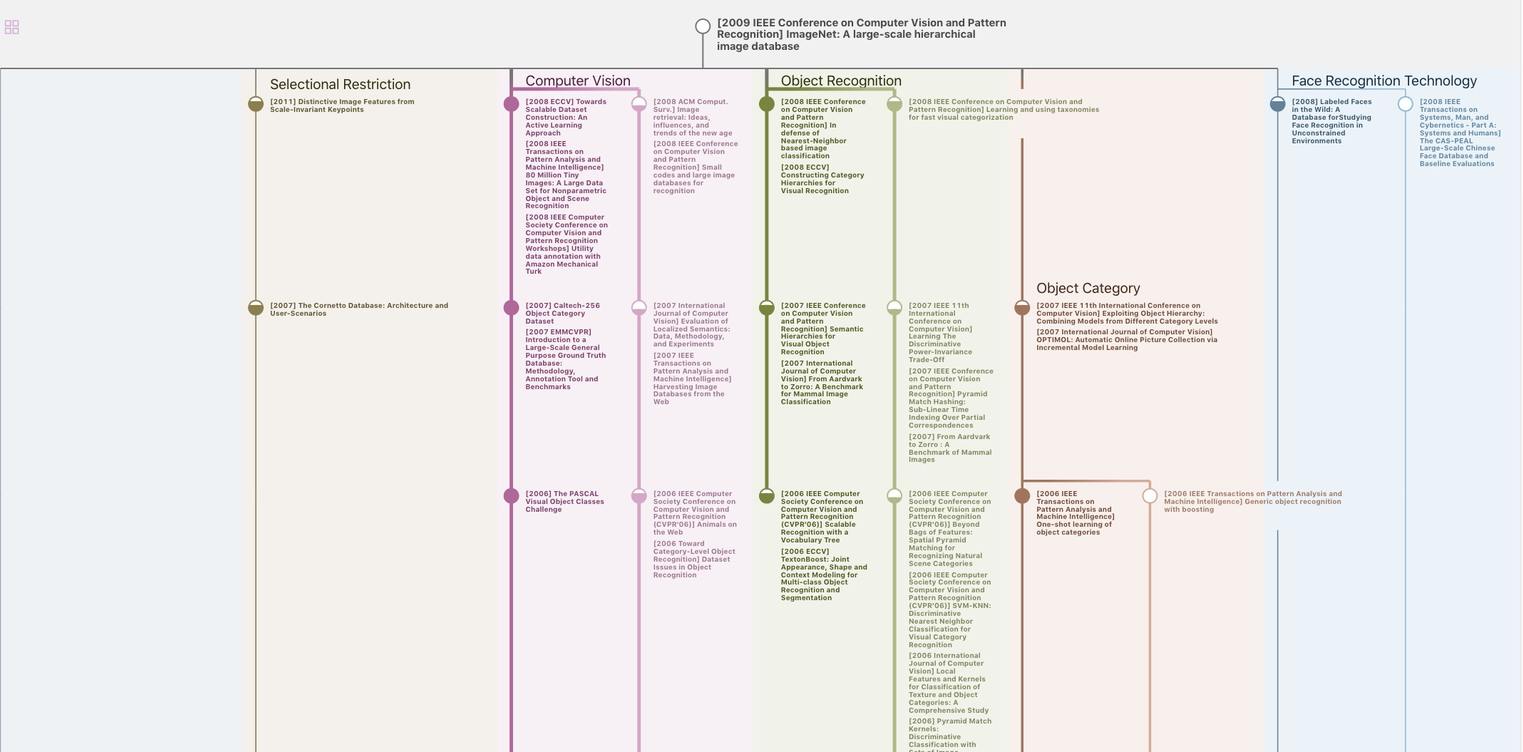
生成溯源树,研究论文发展脉络
Chat Paper
正在生成论文摘要