EFGNet: Encoder steered multi-modality feature guidance network for RGB-D salient object detection.
Digit. Signal Process.(2022)
摘要
Cross-modal complementary information from RGB images and depth maps hasbrought new vitality and progress into salient object detection (SOD). Most existing RGB-D SOD methods generate saliency results by exploring the additional information of depth features, or multi-modality information from RGB and depth data. However, these methods ignore the mutual role between RGB and depth features. Different from them, we reconsider the status of the two modalities and propose an Encoder Steered Multi-modality Feature Guidance Network (EFGNet) to explore the mutual role of the two modalities for RGB-D salient object detection. To this end, a bi-directional framework based on encoder steered strategy is employed to extract and enhance the unimodal features with the aid of another modal data via the circular interactions on the encoder strategy. Specifically, a Multi-modality Feature Guided Module (M2FGM) is proposed to achieve the exploration of multi-modality mutual role, which uses depth features to guide and enhance RGB features (Depth -> RGB), in turn, uses enhanced RGB features to guide and enhance depth features (RGB -> Depth). Furthermore, we also design a Deep Feature-guided Decoder (DGD), which constructs a guidance block by embedding deep decoder features. Comprehensive experiments on six public datasets demonstrate that the proposed EFGNet outperforms state-of-the-art (SOTA) RGB-D SOD methods. Particularly, our method can obtain the percentage gain of 11.1% in term of MAE score on large-scale STERE dataset. Furthermore, the EFGNet runs 160 FPS with 160M model size when testing a 256x256 image on a single NVIDIA 2080Ti GPU. (c) 2022 Elsevier Inc. All rights reserved.
更多查看译文
关键词
RGB-D salient object detection,Multi-modality,Mutual role,Circular interactions
AI 理解论文
溯源树
样例
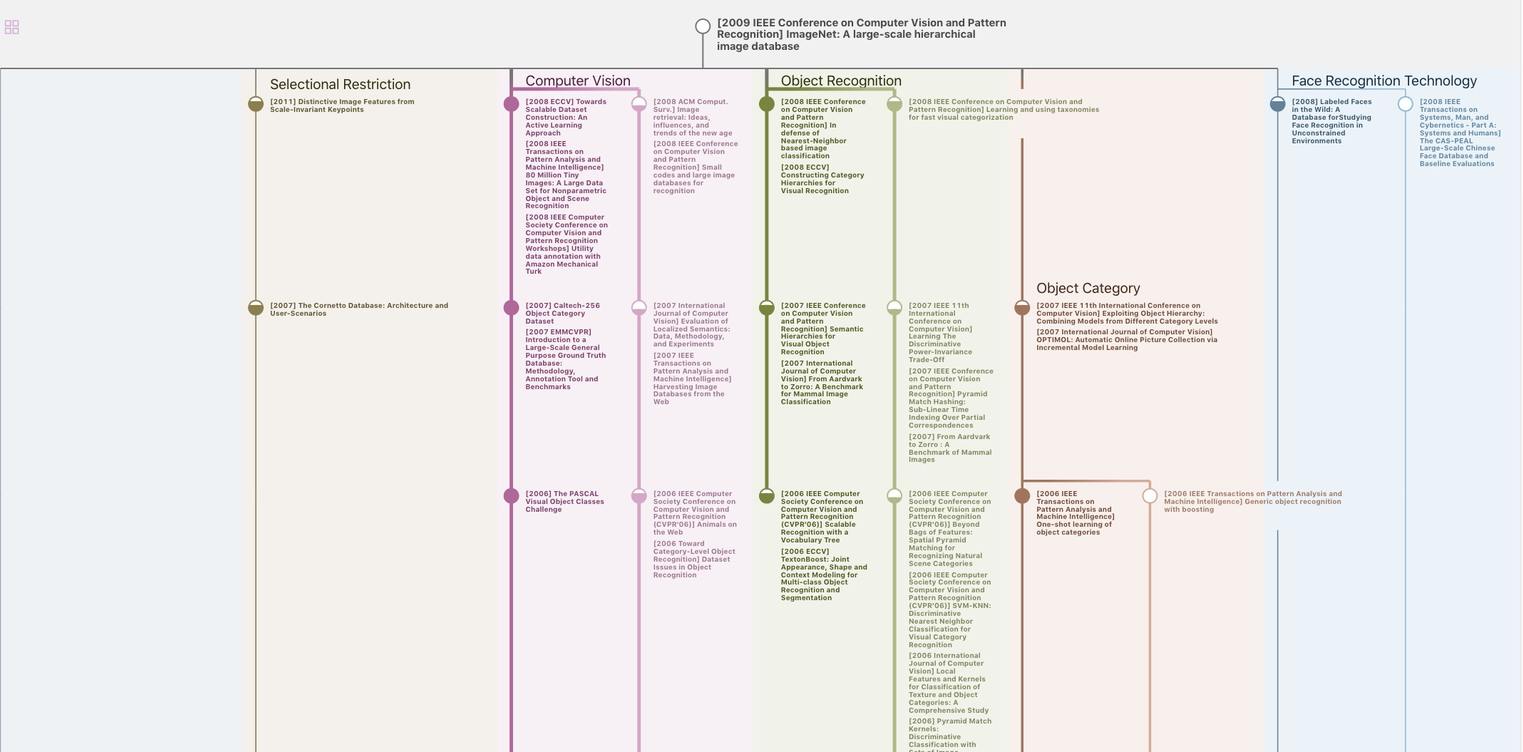
生成溯源树,研究论文发展脉络
Chat Paper
正在生成论文摘要