A Discrete Soft Actor-Critic Decision-Making Strategy With Sample Filter for Freeway Autonomous Driving
IEEE Transactions on Vehicular Technology(2022)
摘要
Autonomous driving is a promising technology to reduce traffic accidents and improve driving efficiency. Although significant progress has been achieved, existing decision-making systems of autonomous vehicle still cannot meet the safety and driving efficiency requirements in highly dynamic environments. In this work, we design a discrete decision-making strategy based on the discrete soft actor-critic with sample filter algorithm (DSAC-SF) to improve driving efficiency and safety on freeways with dynamics traffic. Specifically, we first propose a sample filter method for discrete soft actor-critic, which improves the sample efficiency and stability of the algorithm via enhancing the utilization of effective samples. Subsequently, we construct the discrete decision-making strategy for autonomous driving based on the DSAC-SF algorithm, and further design the area observation method and the multi-objective reward function to improve the driving safety and efficiency. Finally, we carry out comparison and ablation experiments on the the scalable multi-agent reinforcement learning training school (SMARTS) simulation environment. Experimental results indicate that our strategy obtains a high success rate and a fast vehicle speed in the decision-making tasks on freeways. Moreover, our DSAC-SF algorithm also achieves improved performance in training efficiency and stability compared to the commonly used discrete reinforcement learning algorithm.
更多查看译文
关键词
Discrete decision-making,autonomous driving,deep reinforcement learning,soft actor-critic
AI 理解论文
溯源树
样例
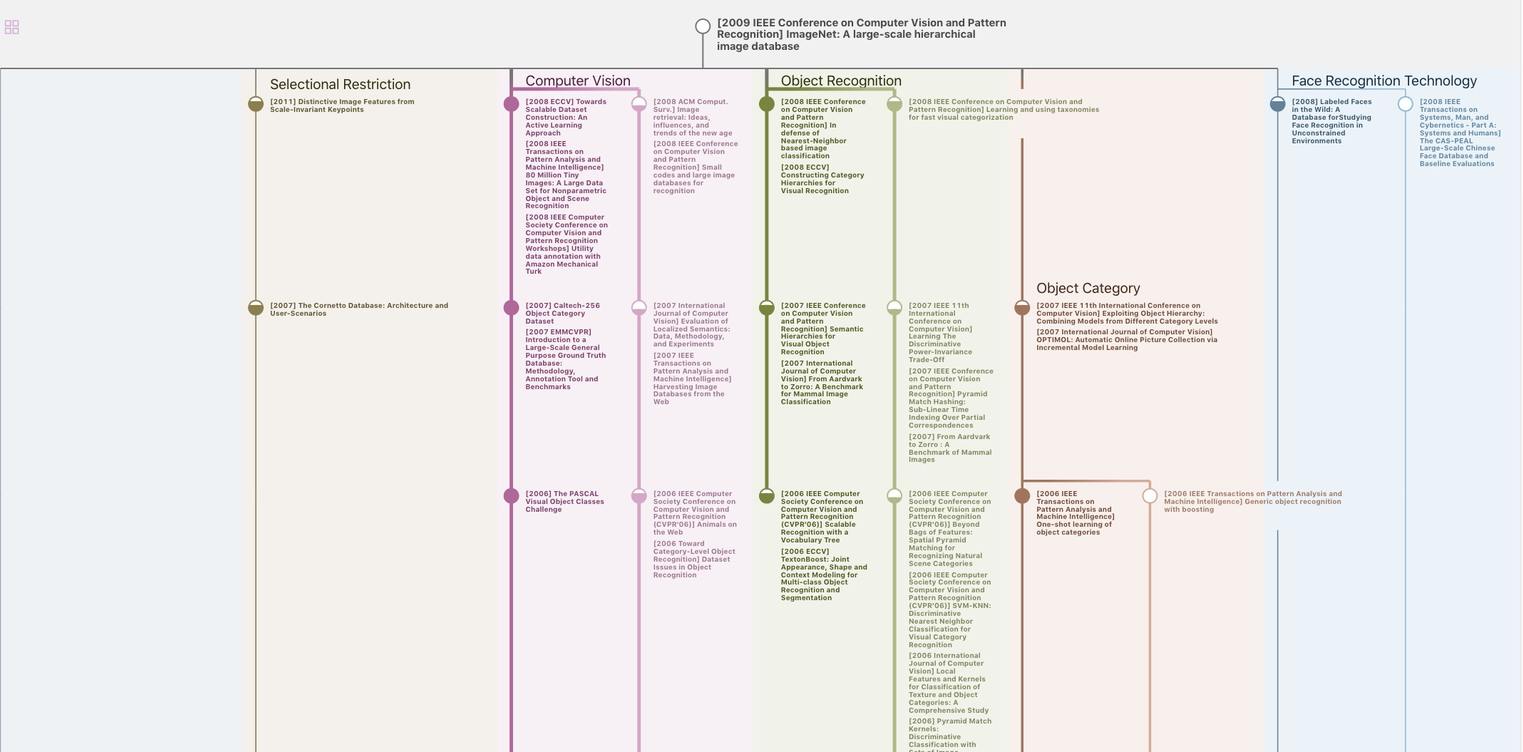
生成溯源树,研究论文发展脉络
Chat Paper
正在生成论文摘要