A MobileNetV2-SENet-based method for identifying fish school feeding behavior
Aquacultural Engineering(2022)
摘要
Analyzing fish school feeding behavior can assist aquaculturists in making successful feeding decisions, which is critical for enhancing farming efficiency and supporting healthy fish growth. Due to the fierce gathering, jumping, chasing and other actions that occur during fish school feeding, images contain varying degrees of noise and significant overlap between fish targets. Therefore, accurately identifying fish school feeding behavior becomes challenging. To address this problem, the study proposes a MobileNetV2-SENet-based method for identifying fish school feeding behavior. To enhance sample diversity, fish school images are preprocessed with some operations, such as random cropping and brightness-contrast adjustment. Then, MobileNetV2 is used to extract fish school image features, and a feature weighting network based on SENet (Squeeze-and-Excitation Networks) is built. Weights are assigned to features with varying degrees of value. The feeding behavior of fish school is identified using the linear classifier. Finally, a method of determining feeding amount is provided based on the identification result to reduce feed consumption. The SENet is introduced in the proposed method based on the original MobileNetV2 feature extraction network, and a feature extraction and weighting network that integrates MobileNetV2 and SENet is built to improve the weights of features relevant for fish school state identification while suppressing the weights of interference elements such as noise, resulting in more accurate identification. The proposed method was tested on the real fish school images and yielded an accuracy of 97.76%. The accuracy of our model was improved by 3.81%, 9.99%, 50.35%, and 100.37% when compared to the fish school feeding behavior identification model based on EfficientNet_B0, ShuffleNetV2, AlexNet, and EfficientNetV2, respectively, indicating that our method can accurately identify the feeding behavior of fish school in real aquaculture.
更多查看译文
关键词
Aquaculture,Feeding behavior,Deep learning,MobileNetV2,SENet
AI 理解论文
溯源树
样例
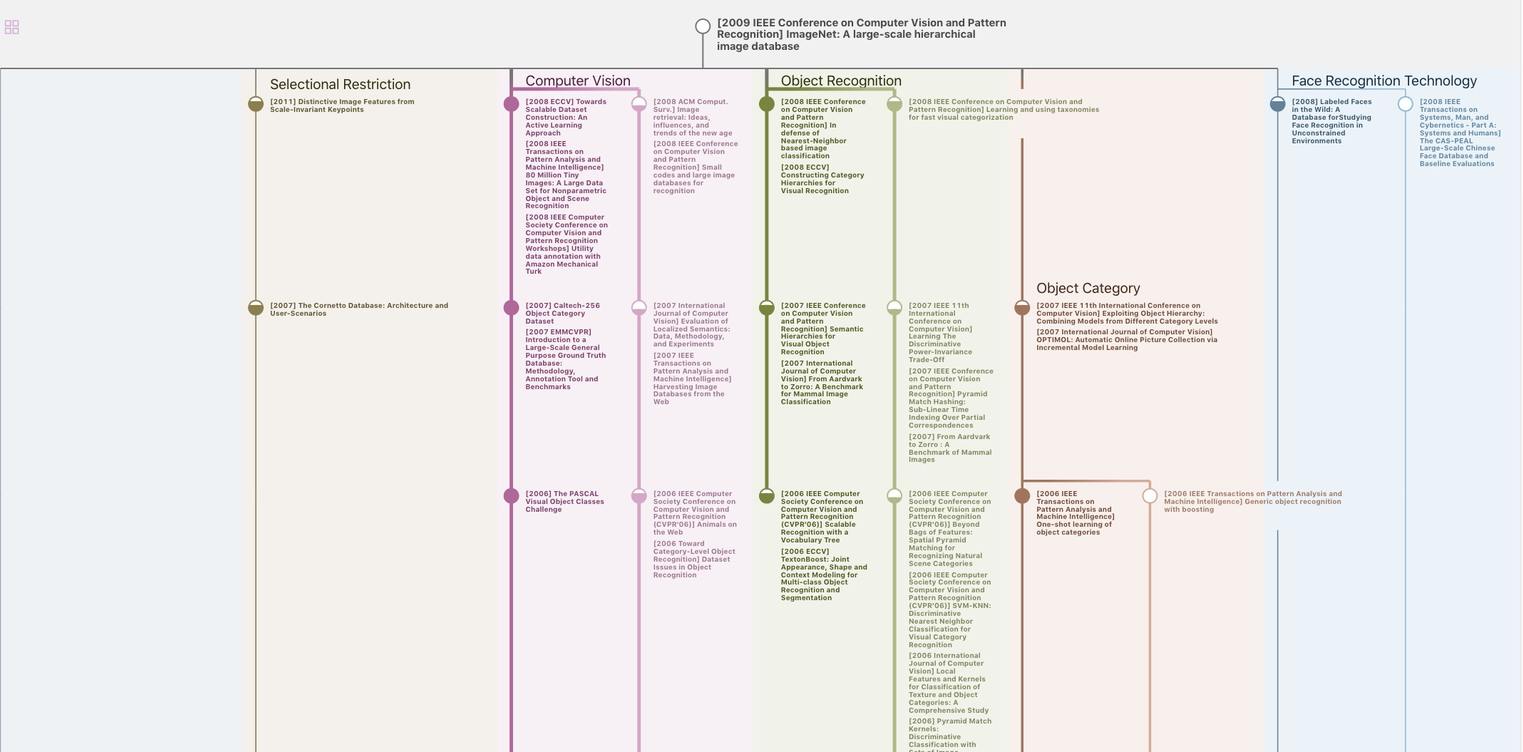
生成溯源树,研究论文发展脉络
Chat Paper
正在生成论文摘要