Deep Learning-Based Channel Modeling for Free Space Optical Communications
Journal of Lightwave Technology(2023)
摘要
Instead of conventional knowledge-driven modeling, this study introduced data-driven channel modeling methods based on deep learning (DL) in a free-space optical (FSO) communication system to achieve low complexity, less modeling difficulty, high fidelity, and differentiability. The FSO channel was modeled via three DL algorithms: generative adversarial network (GAN), bidirectional long short-term memory (BiLSTM), and Bayesian neural network (BNN). Consequently, their performance was comprehensively compared in terms of optical amplitude waveforms, Kullback-Leibler (KL) divergence, 95% confidence interval, data distribution, and fitted probability density functions. The results indicated that the GAN-based channel exhibited optimal performance, implying its suitability for learning the stochastic distribution of the FSO channel. In addition, the performance of GAN-based channels was examined under different conditions: turbulence intensities (weak, moderate, and strong), transmission distances (3, 4, and 5 km), launch powers (0, 10, and 20 dBm), modulation formats (on-off keying, pulse position modulation, and pulse amplitude modulation 4), and the adaptability to channel variation. The test results were satisfactory and confirmed the ability of the GAN to learn the approximate distribution function of the FSO channel. Thus, the primary aim was to prove that for FSO channels with statistical distribution characteristics, a data-driven DL model can not only serve as an effective surrogate model for specific scenarios but also provide a potential tool for future modeling methods.
更多查看译文
关键词
And generative adversarial network (GAN),Bayesian neural network (BNN),bidirectional long short-term memory (BiLSTM),data-driven,deep learning,free-space optical channel modeling
AI 理解论文
溯源树
样例
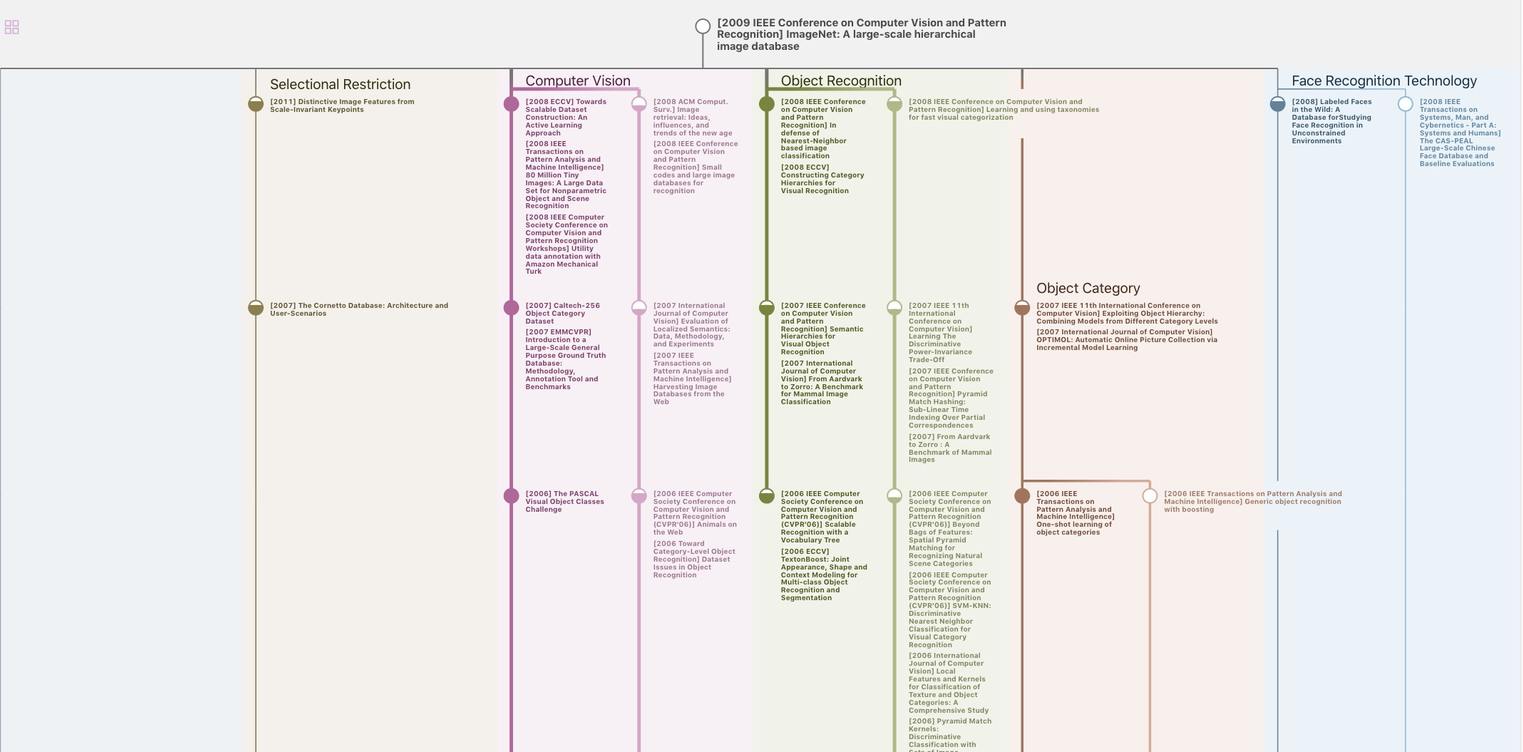
生成溯源树,研究论文发展脉络
Chat Paper
正在生成论文摘要