LOCALIZING ATYPICAL ATRIAL FLUTTER WITH AN ADVANCED MACHINE LEARNING PIPELINE
Cardiovascular Digital Health Journal(2022)
摘要
BackgroundAtypical atrial flutter (AFL) is an increasingly encountered arrythmia. Unlike typical AFL, it may arise from either atrium. There are currently no accurate methods for distinguishing atrium of origin on surface ECG, which has important implications for procedural planning and risk-benefit discussions.ObjectiveDevelop a machine learning algorithm that can localize atypical AFL to the atrium of origin.MethodsWe identified patients who had undergone AFL ablation with electroanatomical mapping at Northwestern Hospital. Patients were included if the location of AFL was stated in the procedure report and they had an ECG of AFL available for analysis. Machine learning (ML) training / prediction was implemented in two stages, (i) typical vs atypical and (ii) right vs left atrial atypical (Figure 1). The first stage used a pipeline that suppresses the QRS, smooths the signal and extracts the frequency-based coefficients, which are fed into an ensemble ML model of random forests, support vector machines and k-nearest neighbors. In the second stage, the atypical ECGs underwent Dower transformation to vectorcardiograms and manual flutter wave extraction, which were used to calculate a new set of features prior to ensemble learning. Majority voting classified each atypical flutter as left or right atrial.ResultsStage one classified AFL as typical or atypical with 98% accuracy. The second stage classified each unique wave as right or left atrial with 74% accuracy. When combining flutter waves that belong to each individual patient using a majority vote as the final classifier, the entire two-stage pipeline had an overall accuracy of 98% for the 3-group AFL classification task (Figure 2).ConclusionView Large Image Figure ViewerDownload Hi-res image Download (PPT)Classification matrix BackgroundAtypical atrial flutter (AFL) is an increasingly encountered arrythmia. Unlike typical AFL, it may arise from either atrium. There are currently no accurate methods for distinguishing atrium of origin on surface ECG, which has important implications for procedural planning and risk-benefit discussions. Atypical atrial flutter (AFL) is an increasingly encountered arrythmia. Unlike typical AFL, it may arise from either atrium. There are currently no accurate methods for distinguishing atrium of origin on surface ECG, which has important implications for procedural planning and risk-benefit discussions. ObjectiveDevelop a machine learning algorithm that can localize atypical AFL to the atrium of origin. Develop a machine learning algorithm that can localize atypical AFL to the atrium of origin. MethodsWe identified patients who had undergone AFL ablation with electroanatomical mapping at Northwestern Hospital. Patients were included if the location of AFL was stated in the procedure report and they had an ECG of AFL available for analysis. Machine learning (ML) training / prediction was implemented in two stages, (i) typical vs atypical and (ii) right vs left atrial atypical (Figure 1). The first stage used a pipeline that suppresses the QRS, smooths the signal and extracts the frequency-based coefficients, which are fed into an ensemble ML model of random forests, support vector machines and k-nearest neighbors. In the second stage, the atypical ECGs underwent Dower transformation to vectorcardiograms and manual flutter wave extraction, which were used to calculate a new set of features prior to ensemble learning. Majority voting classified each atypical flutter as left or right atrial. We identified patients who had undergone AFL ablation with electroanatomical mapping at Northwestern Hospital. Patients were included if the location of AFL was stated in the procedure report and they had an ECG of AFL available for analysis. Machine learning (ML) training / prediction was implemented in two stages, (i) typical vs atypical and (ii) right vs left atrial atypical (Figure 1). The first stage used a pipeline that suppresses the QRS, smooths the signal and extracts the frequency-based coefficients, which are fed into an ensemble ML model of random forests, support vector machines and k-nearest neighbors. In the second stage, the atypical ECGs underwent Dower transformation to vectorcardiograms and manual flutter wave extraction, which were used to calculate a new set of features prior to ensemble learning. Majority voting classified each atypical flutter as left or right atrial. ResultsStage one classified AFL as typical or atypical with 98% accuracy. The second stage classified each unique wave as right or left atrial with 74% accuracy. When combining flutter waves that belong to each individual patient using a majority vote as the final classifier, the entire two-stage pipeline had an overall accuracy of 98% for the 3-group AFL classification task (Figure 2). Stage one classified AFL as typical or atypical with 98% accuracy. The second stage classified each unique wave as right or left atrial with 74% accuracy. When combining flutter waves that belong to each individual patient using a majority vote as the final classifier, the entire two-stage pipeline had an overall accuracy of 98% for the 3-group AFL classification task (Figure 2). ConclusionClassification matrix Classification matrix
更多查看译文
AI 理解论文
溯源树
样例
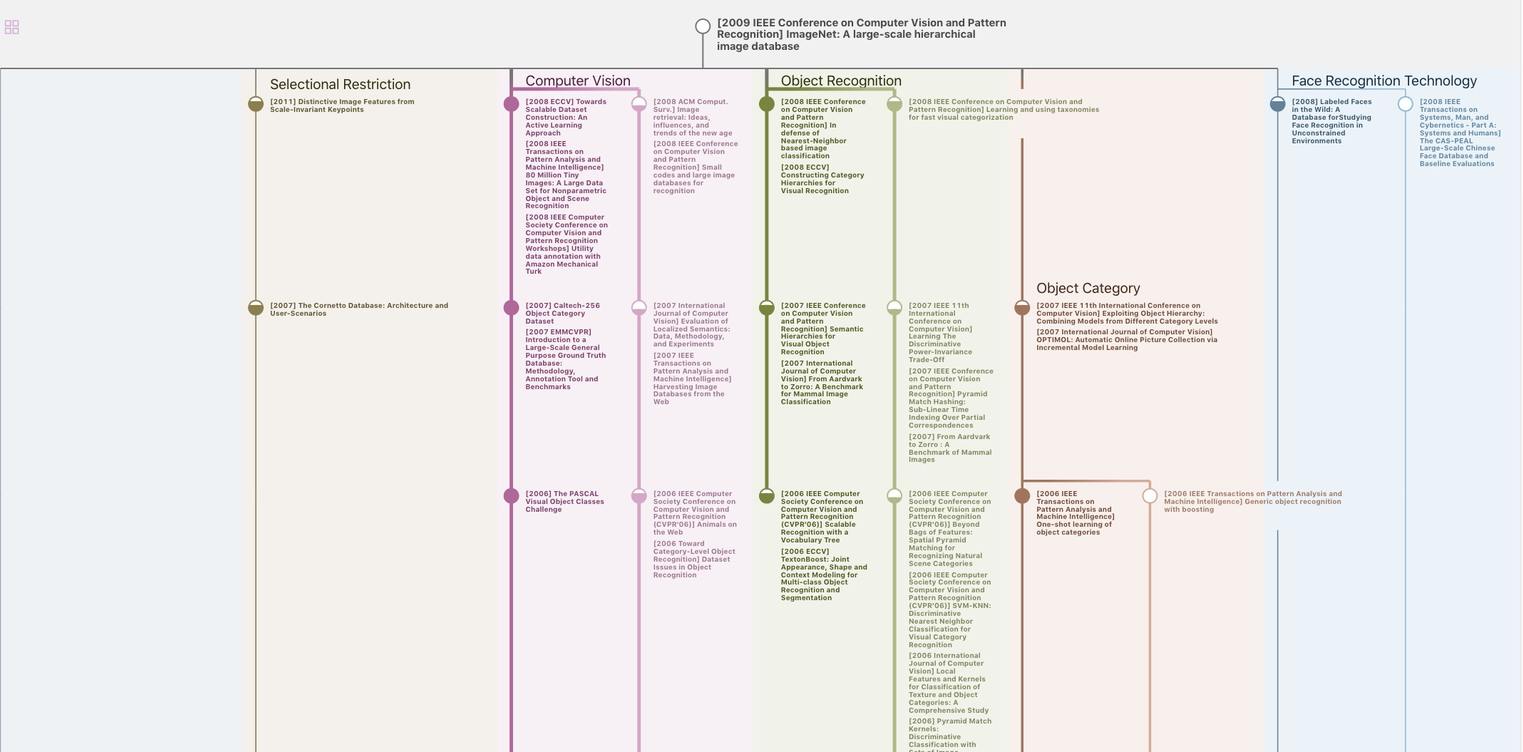
生成溯源树,研究论文发展脉络
Chat Paper
正在生成论文摘要