A Vehicle Classification Model Based on Multi-scale Feature Fusion
2022 41st Chinese Control Conference (CCC)(2022)
摘要
In the field of intelligent transportation, the classification of vehicle types plays a very important role. This paper presents a model of SPP-RepVGG to overcome the problems of long training time and low classification accuracy of existing vehicle types classification model. Firstly, the RepVGG is employed in extracting effective feature data of the vehicle image. Then, the Spatial Pyramid Pooling (SPP) is connected between the convolution layer and the fully connected layer of the model, and the multi-scale features of the image are extracted through three different sizes of pooling windows, thus feature extraction and fusion of the vehicle image are realized. Finally, the classification results are obtained using the fully connected layer. The Stochastic Gradient Descent (SGD) method with Gradient Centralization (GC) is used to accelerate the network training. Four classification models are applied to the BIT-Vehicle data sets for comparative experiments. The experimental results demonstrate that the training time of SPP-RepVGG model is shortened, and the accuracy on the test sets is 96.653%. Compared with the VGG-16, ResNet-18 and RepVGG-A0 models, the Top-1 accuracy of the proposed model is increased by 3.034%, 2.197% and 1.988% respectively, this illustrates that our proposed model can extract vehicle image features more effectively, which can provide help for intelligent traffic management.
更多查看译文
关键词
Vehicle Types Classification,RepVGG,Multi-scale Feature,SPP,GC
AI 理解论文
溯源树
样例
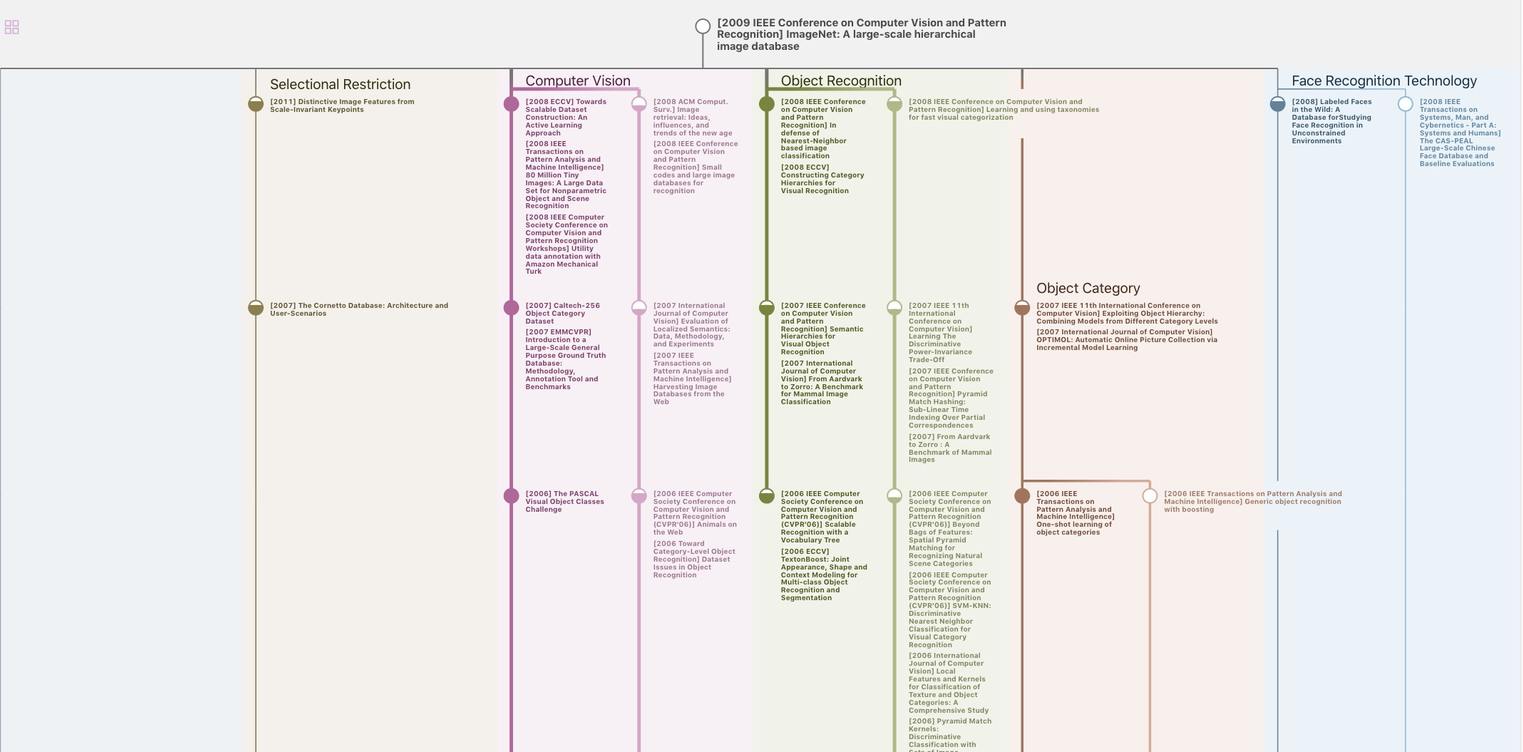
生成溯源树,研究论文发展脉络
Chat Paper
正在生成论文摘要