Scalable Reasoning and Sensing Using Processing-In-Memory With Hybrid Spin/CMOS-Based Analog/Digital Blocks
IEEE Transactions on Emerging Topics in Computing(2022)
摘要
In this article, we leverage in-memory computation for data-intensive applications to surmount the bandwidth restrictions inherent in the Von-Neumann computing paradigm, while addressing transistor technology scaling challenges facing Moore's Law. We introduce
the Spintronically Configurable Analog Processing in-memory Environment (SCAPE)
which incorporates top-down architectural approaches along with bottom-up intrinsic device switching behaviors of spin-based post-CMOS devices. SCAPE embeds analog arithmetic capabilities providing a selectable thresholding functionality to realize generalized neuron activation functions that are integrated within the 2-dimensional memory array. Within each module, circuit-switched connections allow in-field configuration of the partly reconfigurable neuron activation function to suit the target application, and the intrinsic computation is performed using spin-based devices. This hybrid-technology design advances in-memory computation beyond previous approaches by integrating analog arithmetic, runtime reconfigurability, and non-volatile devices within a selectable 2-dimensional topology. Simulation results of error rates, power consumption, power-error-product metric, are examined for real-world applications including edge-of-network based Compressive Sensing and Machine Learning use cases, along with process variation analysis. Results show up to 7% improvement in error rate using proposed implementation of enhanced activation function versus baseline conventional sigmoidal activation, whereas realization of AMP signal processing algorithm shows ∼95% reduction in energy consumption at comparable accuracy.
更多查看译文
关键词
analog/digital blocks,processing-in-memory,cmos-based
AI 理解论文
溯源树
样例
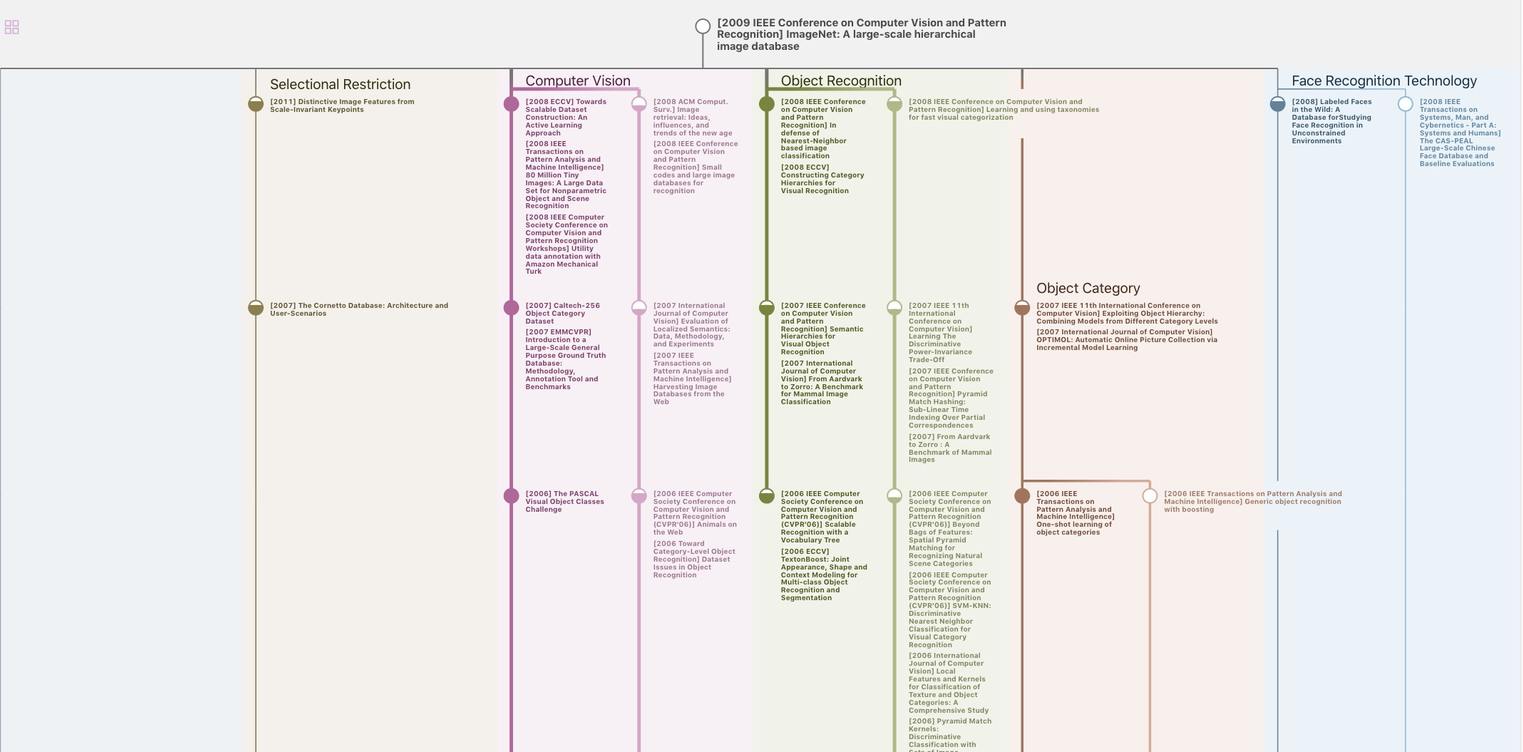
生成溯源树,研究论文发展脉络
Chat Paper
正在生成论文摘要