Data-driven scenario generation for two-stage stochastic programming
Chemical Engineering Research and Design(2022)
摘要
Optimisation under uncertainty has always been a focal point within the Process Systems Engineering (PSE) research agenda. In particular, the efficient manipulation of large amount of data for the uncertain parameters constitutes a crucial condition for effectively tackling stochastic programming problems. In this context, this work proposes a new data-driven Mixed-Integer Linear Programming (MILP) model for the Distribution & Moment Matching Problem (DMP). For cases with multiple uncertain parameters a copula-based simulation of initial scenarios is employed as preliminary step. Moreover, the integration of clustering methods and DMP in the proposed model is shown to enhance computational performance. Finally, we compare the proposed approach with state-of-the-art scenario generation methodologies. Through a number of case studies we highlight the benefits regarding the quality of the generated scenario trees by evaluating the corresponding obtained stochastic solutions.
更多查看译文
关键词
Scenario generation,Stochastic programming,Data-driven optimisation,Moment Matching Problem,Copulas
AI 理解论文
溯源树
样例
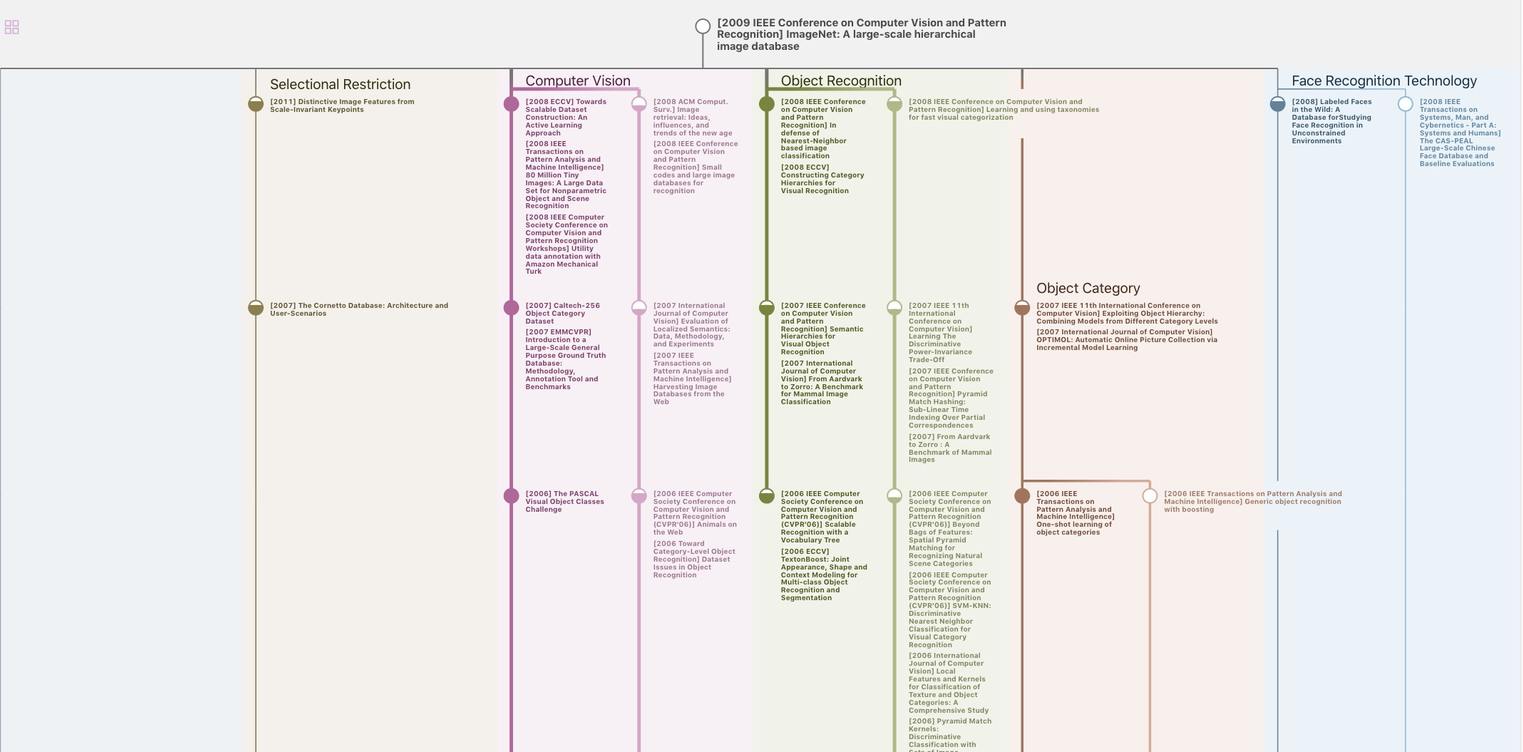
生成溯源树,研究论文发展脉络
Chat Paper
正在生成论文摘要