Data-Driven Feedback Linearization using the Koopman Generator
arxiv(2022)
摘要
This paper contributes a theoretical framework for data-driven feedback linearization of nonlinear control-affine systems. We unify the traditional geometric perspective on feedback linearization with an operator-theoretic perspective involving the Koopman operator. We first show that if the distribution of the control vector field and its repeated Lie brackets with the drift vector field is involutive, then there exists an output and a feedback control law for which the Koopman generator is finite-dimensional and locally nilpotent. We use this connection to propose a data-driven algorithm for feedback linearization. Particularly, we use experimental data to identify the state transformation and control feedback from a dictionary of functions for which feedback linearization is achieved in a least-squares sense. Finally, we provide numerical examples for the data-driven algorithm and compare it with model-based feedback linearization. We also numerically study the effect of the richness of the dictionary and the size of the data set on the effectiveness of feedback linearization.
更多查看译文
关键词
feedback linearization,data-driven
AI 理解论文
溯源树
样例
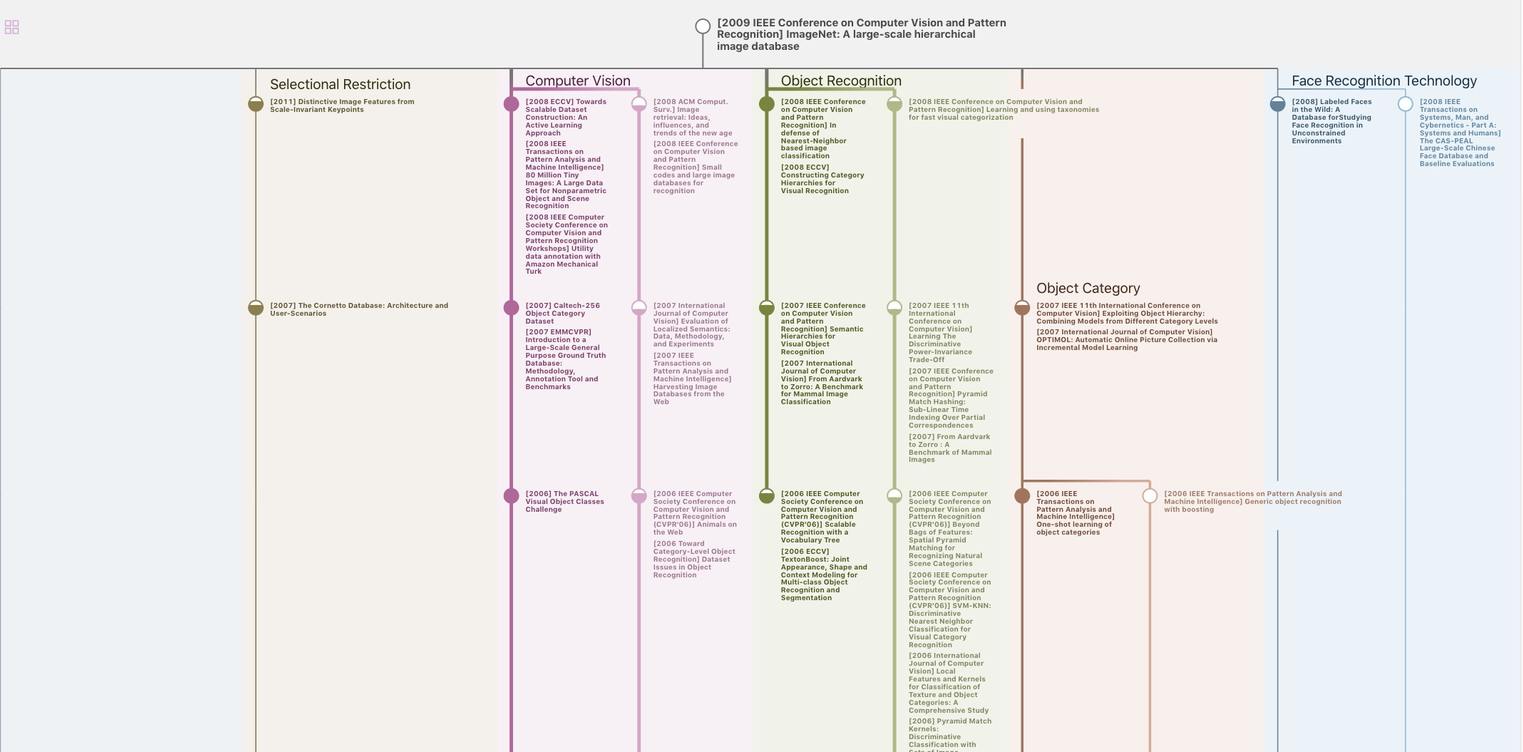
生成溯源树,研究论文发展脉络
Chat Paper
正在生成论文摘要