Uplifting Message Passing Neural Network with Graph Original Information
arxiv(2023)
摘要
Message passing neural networks (MPNNs) learn the representation of graph-structured data based on graph original information, including node features and graph structures, and have shown astonishing improvement in node classification tasks. However, the expressive power of MPNNs is upper bounded by the first-order Weisfeiler-Leman test and its accuracy still has room for improvement. This work studies how to improve MPNNs' expressiveness and generalizability by fully exploiting graph original information both theoretically and empirically. It further proposes a new GNN model called INGNN (INformation-enhanced Graph Neural Network) that leverages the insights to improve node classification performance. Extensive experiments on both synthetic and real datasets demonstrate the superiority (average rank 1.78) of our INGNN compared with state-of-the-art methods.
更多查看译文
关键词
message passing neural network,neural network,graph
AI 理解论文
溯源树
样例
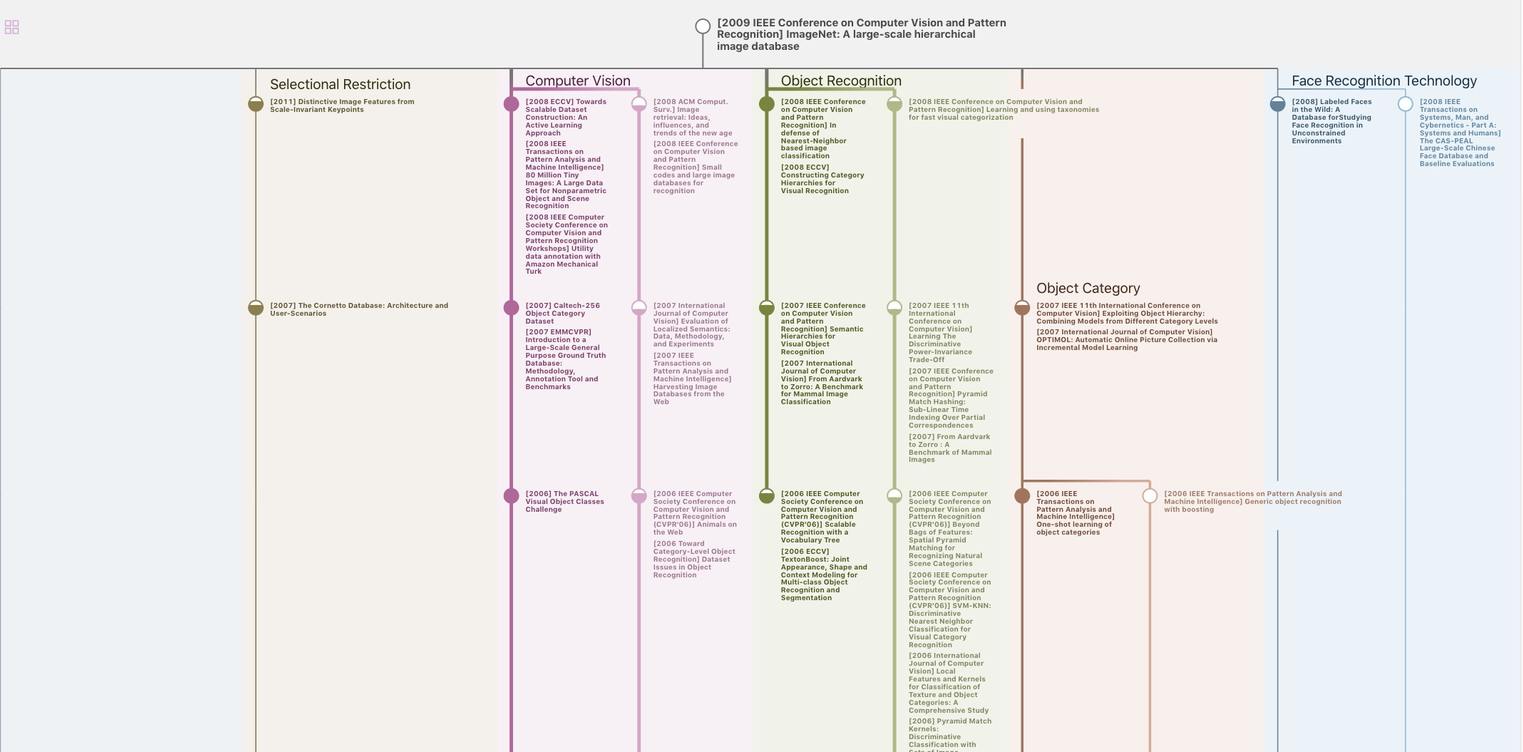
生成溯源树,研究论文发展脉络
Chat Paper
正在生成论文摘要