OPERA: Omni-Supervised Representation Learning with Hierarchical Supervisions
2023 IEEE/CVF International Conference on Computer Vision (ICCV)(2022)
摘要
The pretrain-finetune paradigm in modern computer vision facilitates the success of self-supervised learning, which tends to achieve better transferability than supervised learning. However, with the availability of massive labeled data, a natural question emerges: how to train a better model with both self and full supervision signals? In this paper, we propose Omni-suPErvised Representation leArning with hierarchical supervisions (OPERA) as a solution. We provide a unified perspective of supervisions from labeled and unlabeled data and propose a unified framework of fully supervised and self-supervised learning. We extract a set of hierarchical proxy representations for each image and impose self and full supervisions on the corresponding proxy representations. Extensive experiments on both convolutional neural networks and vision transformers demonstrate the superiority of OPERA in image classification, segmentation, and object detection. Code is available at: https://github.com/wangck20/OPERA.
更多查看译文
关键词
representation,learning,omni-supervised
AI 理解论文
溯源树
样例
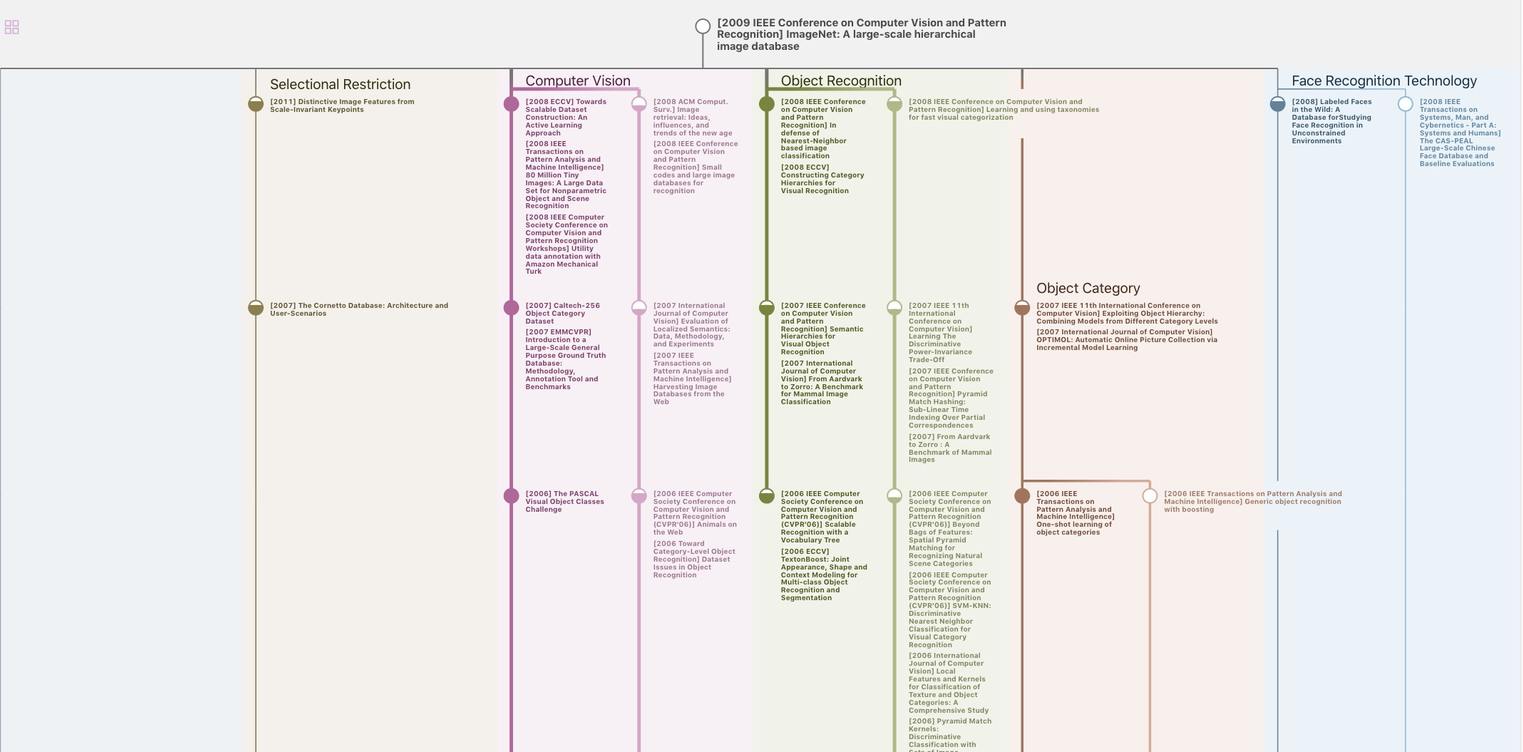
生成溯源树,研究论文发展脉络
Chat Paper
正在生成论文摘要