Contrastive Training Improves Zero-Shot Classification of Semi-structured Documents
conf_acl(2022)
摘要
We investigate semi-structured document classification in a zero-shot setting. Classification of semi-structured documents is more challenging than that of standard unstructured documents, as positional, layout, and style information play a vital role in interpreting such documents. The standard classification setting where categories are fixed during both training and testing falls short in dynamic environments where new document categories could potentially emerge. We focus exclusively on the zero-shot setting where inference is done on new unseen classes. To address this task, we propose a matching-based approach that relies on a pairwise contrastive objective for both pretraining and fine-tuning. Our results show a significant boost in Macro F$_1$ from the proposed pretraining step in both supervised and unsupervised zero-shot settings.
更多查看译文
关键词
contrastive training,classification,documents,zero-shot,semi-structured
AI 理解论文
溯源树
样例
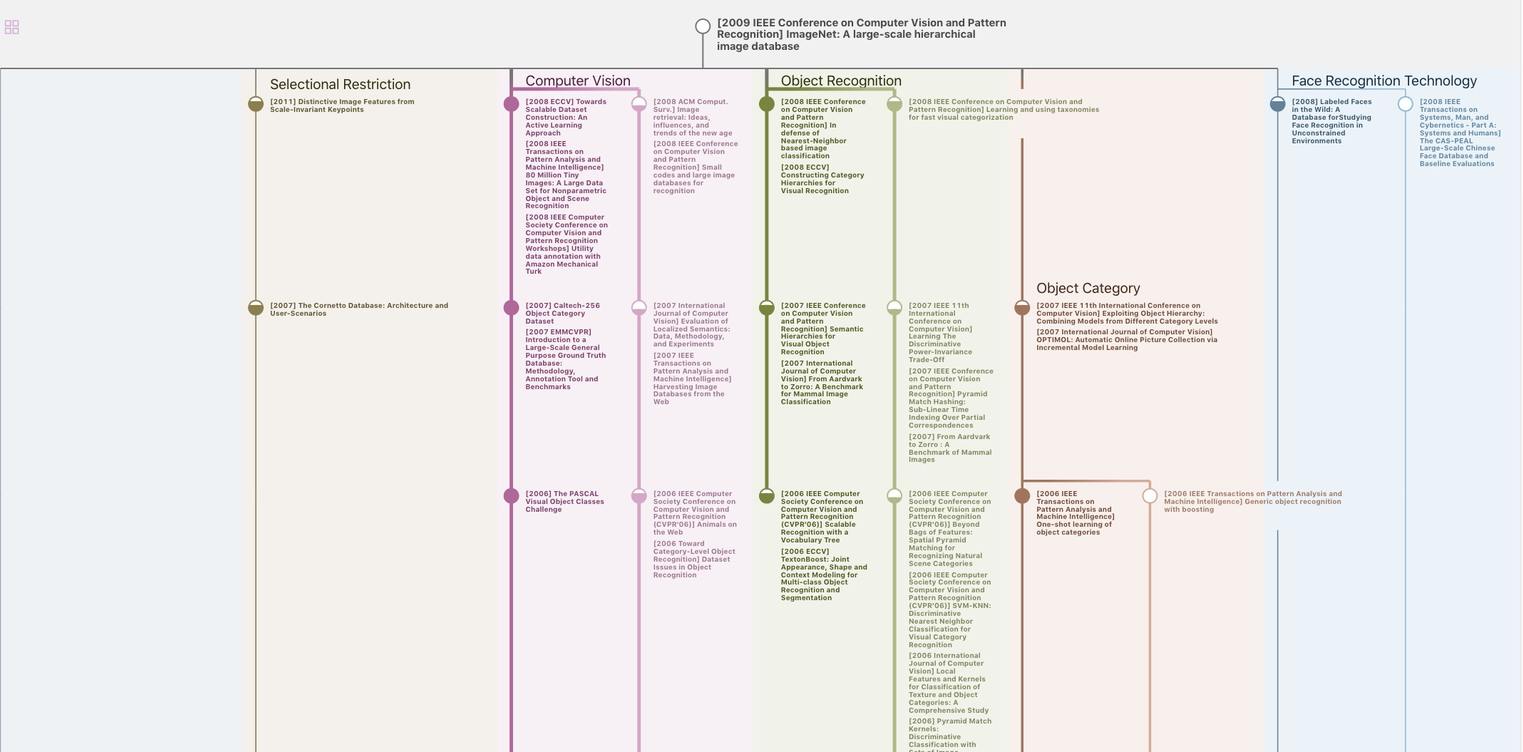
生成溯源树,研究论文发展脉络
Chat Paper
正在生成论文摘要