Rice yield estimation using a CNN-based image-driven data assimilation framework
Field Crops Research(2022)
摘要
The accurate prediction and estimation of grain yield for smallholder farms are crucial for agricultural management. In this study, we propose an image-driven data assimilation framework that enables smallholder farmers to estimate crop yield. In the framework, a convolutional neural network was trained using label distribution learning to estimate the probability distribution of rice crop states from images. The mean and variance of the estimated probability were regarded as observed values and variances, which were then assimilated into the ORYZA2000 crop growth model to estimate the final yield. Compared with the accuracy (R2 = 0.410, RMSE = 1.059 t ha−1) of yield estimation by assimilating field sampling observations, the proposed method significantly improved accuracy, with R2 = 0.646 and RMSE = 0.679 t ha−1. The results demonstrated the feasibility of driving a crop growth model using easily available camera images. Additionally, the probability distribution estimation method made it possible to quantify the error for each observation. The method provides a new perspective for quantifying the observation error, thereby indicating a potential research direction for data assimilation. After that, we used numerous simulation cases to determine the most valuable observation combination for final grain yield estimation, the final yield prediction ability at different development stages, and the model performance affected by number of images. Finally, a series of factors that may affect the applicability of the framework was discussed. This study establishes an intelligent data assimilation framework for smallholder farmers that can absorb crop phenotyping data from digital cameras with a low cost and simple implementation.
更多查看译文
关键词
Data assimilation,Deep learning,Yield estimation,Rice,CNN,Observation uncertainty
AI 理解论文
溯源树
样例
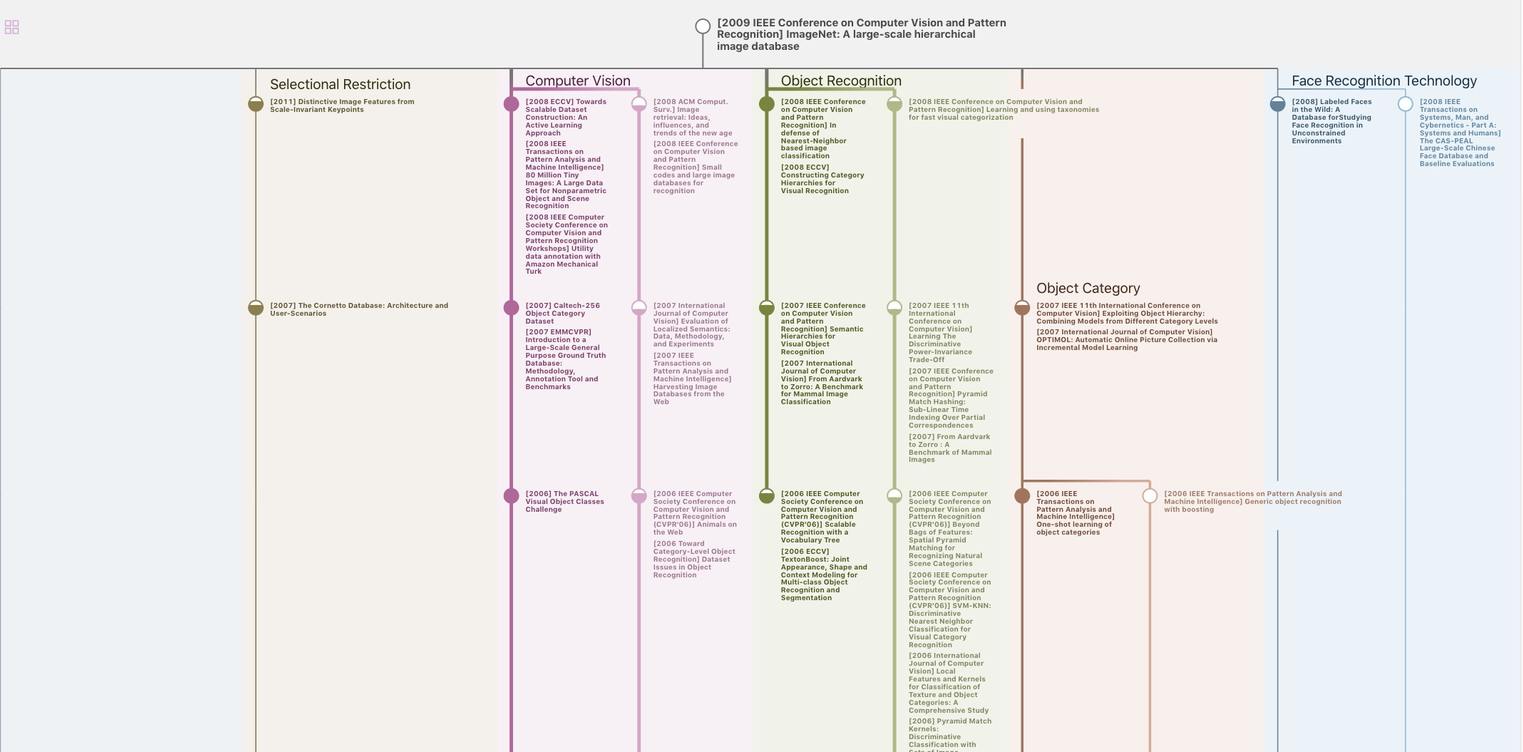
生成溯源树,研究论文发展脉络
Chat Paper
正在生成论文摘要