Progressive 3D biomedical image registration network based on deep self-calibration
FRONTIERS IN NEUROINFORMATICS(2022)
摘要
Three dimensional deformable image registration (DIR) is a key enabling technique in building digital neuronal atlases of the brain, which can model the local non-linear deformation between a pair of biomedical images and align the anatomical structures of different samples into one spatial coordinate system. And thus, the DIR is always conducted following a preprocessing of global linear registration to remove the large global deformations. However, imperfect preprocessing may leave some large non-linear deformations that cannot be handled well by existing DIR methods. The recently proposed cascaded registration network gives a primary solution to deal with such large non-linear deformations, but still suffers from loss of image details caused by continuous interpolation (information loss problem). In this article, a progressive image registration strategy based on deep self-calibration is proposed to deal with the large non-linear deformations without causing information loss and introducing additional parameters. More importantly, we also propose a novel hierarchical registration strategy to quickly achieve accurate multi-scale progressive registration. In addition, our method can implicitly and reasonably implement dynamic dataset augmentation. We have evaluated the proposed method on both optical and MRI image datasets with obtaining promising results, which demonstrate the superior performance of the proposed method over several other state-of-the-art approaches for deformable image registration.
更多查看译文
关键词
biomedical image registration, progressive registration, hierarchical registration, deep self-calibration, dynamic dataset augmentation
AI 理解论文
溯源树
样例
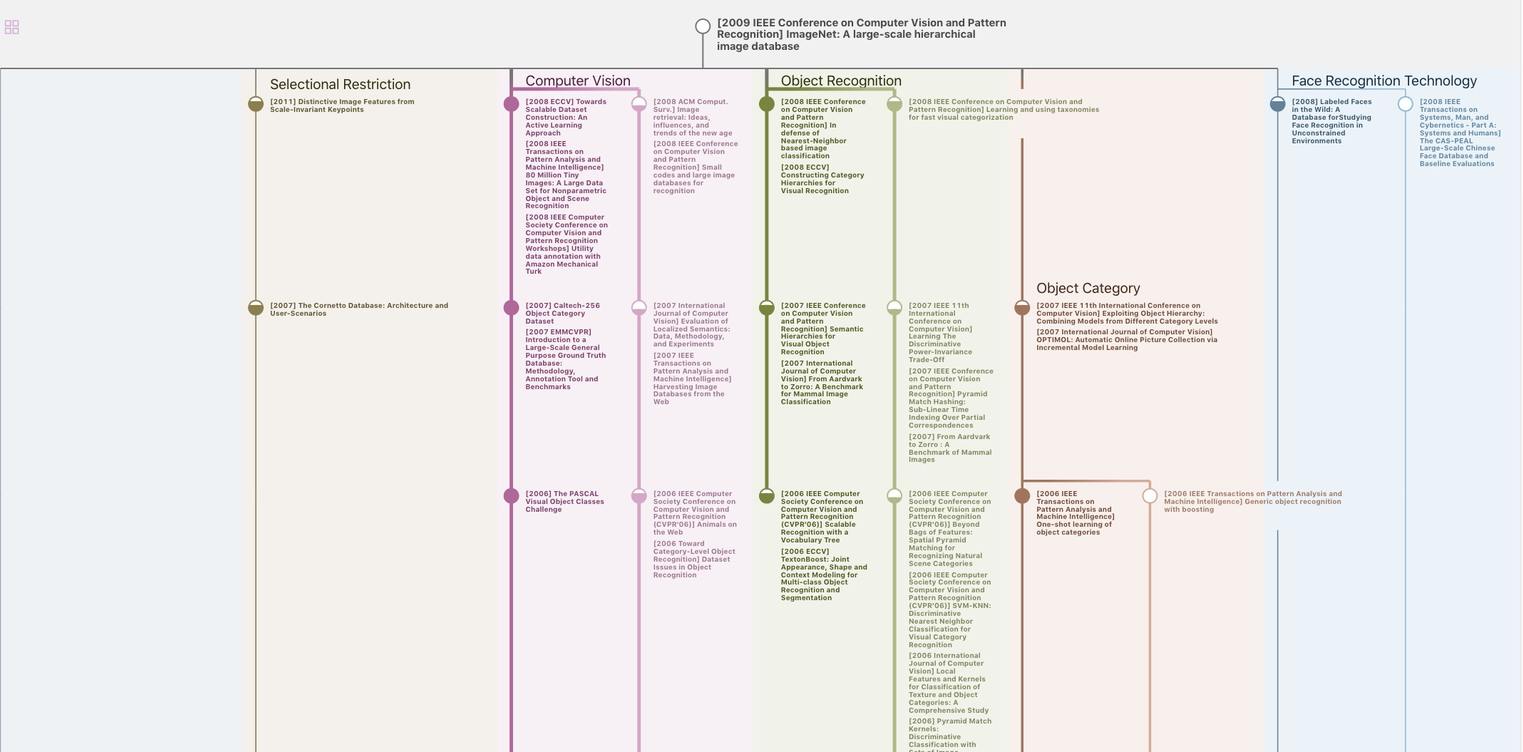
生成溯源树,研究论文发展脉络
Chat Paper
正在生成论文摘要