Dynamic Network Representation Learning Based on Community Structure and Evolutionary Clustering
2022 41st Chinese Control Conference (CCC)(2022)
摘要
Network representation learning, which transforms the nodes in the network into a low-dimensional vector space, has attracted considerable attention due to its flexible and effective ability to execute variable tasks on networks. Most works for network representation learning focus on static networks, and they cannot be effectively applied to dynamic networks that better reflect the underlying properties of complex systems. In this paper, we propose dynNEC, a method for dynamic network representation learning. DynNEC learns node representations using a multi-layer non-negative matrix factorization (NMF) model that integrates local topological structure at the microscopic level, community structure at the mesoscopic level, and dynamic evolutionary information on these two structures. The experimental results on synthetic and real dynamic networks demonstrate that, compared to existing methods, dynNEC provides a high-quality representation for each node in the dynamic networks, as well as effectively performs the tasks of node classification and dynamic community detection.
更多查看译文
关键词
Dynamic networks,representation learning,non-negative matric factorization,community structure
AI 理解论文
溯源树
样例
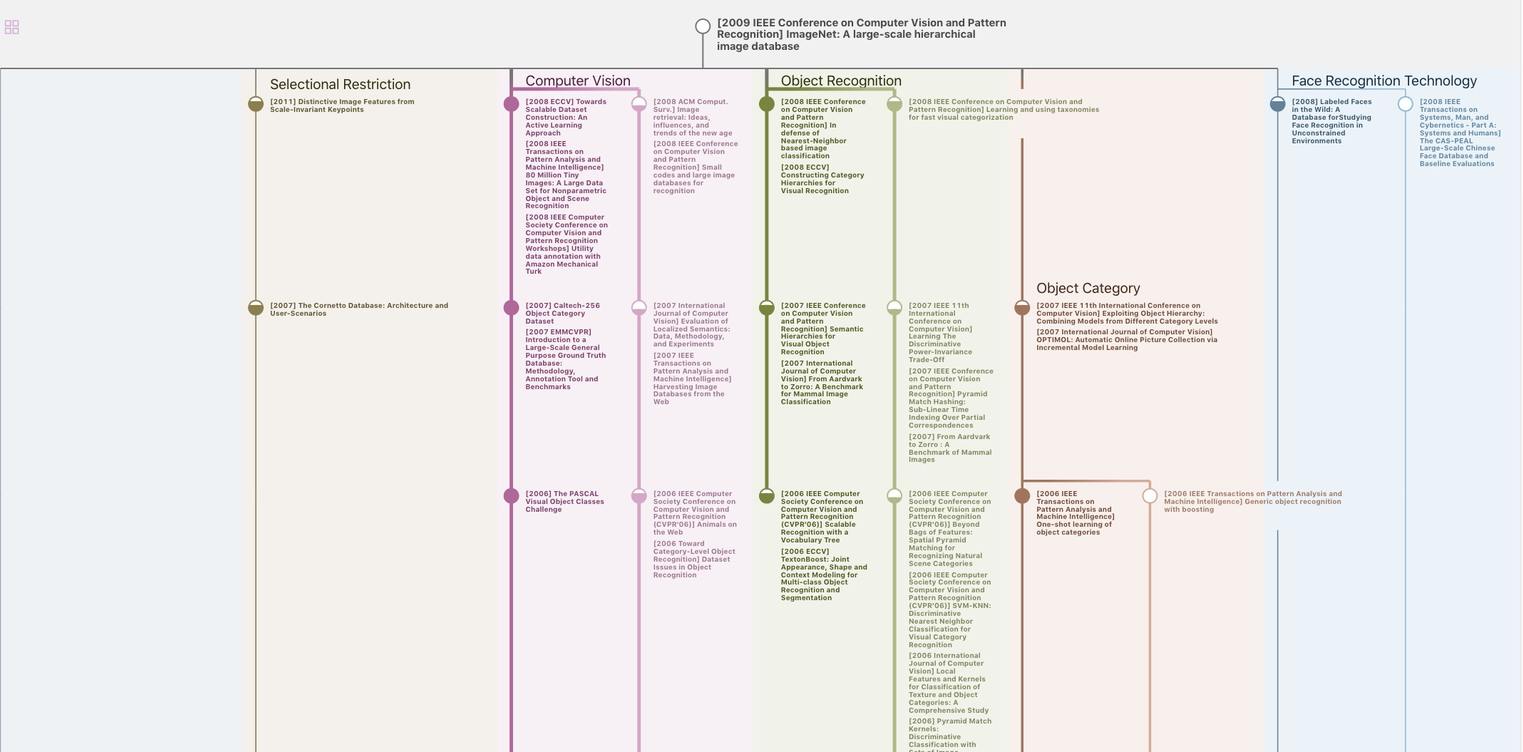
生成溯源树,研究论文发展脉络
Chat Paper
正在生成论文摘要