Transfer Learning For Crowed Counting
2022 IEEE 2nd International Maghreb Meeting of the Conference on Sciences and Techniques of Automatic Control and Computer Engineering (MI-STA)(2022)
摘要
Transfer learning for crowd counting via CNN is explored in this research to minimize training time and computational cost. The Mall dataset is used to evaluate the effectiveness of the transfer learning approach and is compared with other recent techniques that use their own deep architecture. By using transfer learning, less computation is involved because the pretrained model has already learned the necessary weightage and architecture thus reducing time consumption for training. The ResNet50 model is fine-tuned to be applying to the Mall dataset. The result of this project shows that using ResNet50 for transfer learning achieved mediocre MAE and MSE compared to other recent techniques such as the DeepCount and DecideNet models. Therefore, application of transfer learning in crowd counting using CNN for ResNet50 on Mall dataset was proven inefficient and further improvements needs to be carried out for this application to be beneficial
更多查看译文
关键词
Crowd counting,convolutional neural network,Deepcount,MAE,MSE,DecideNet,ResNet50
AI 理解论文
溯源树
样例
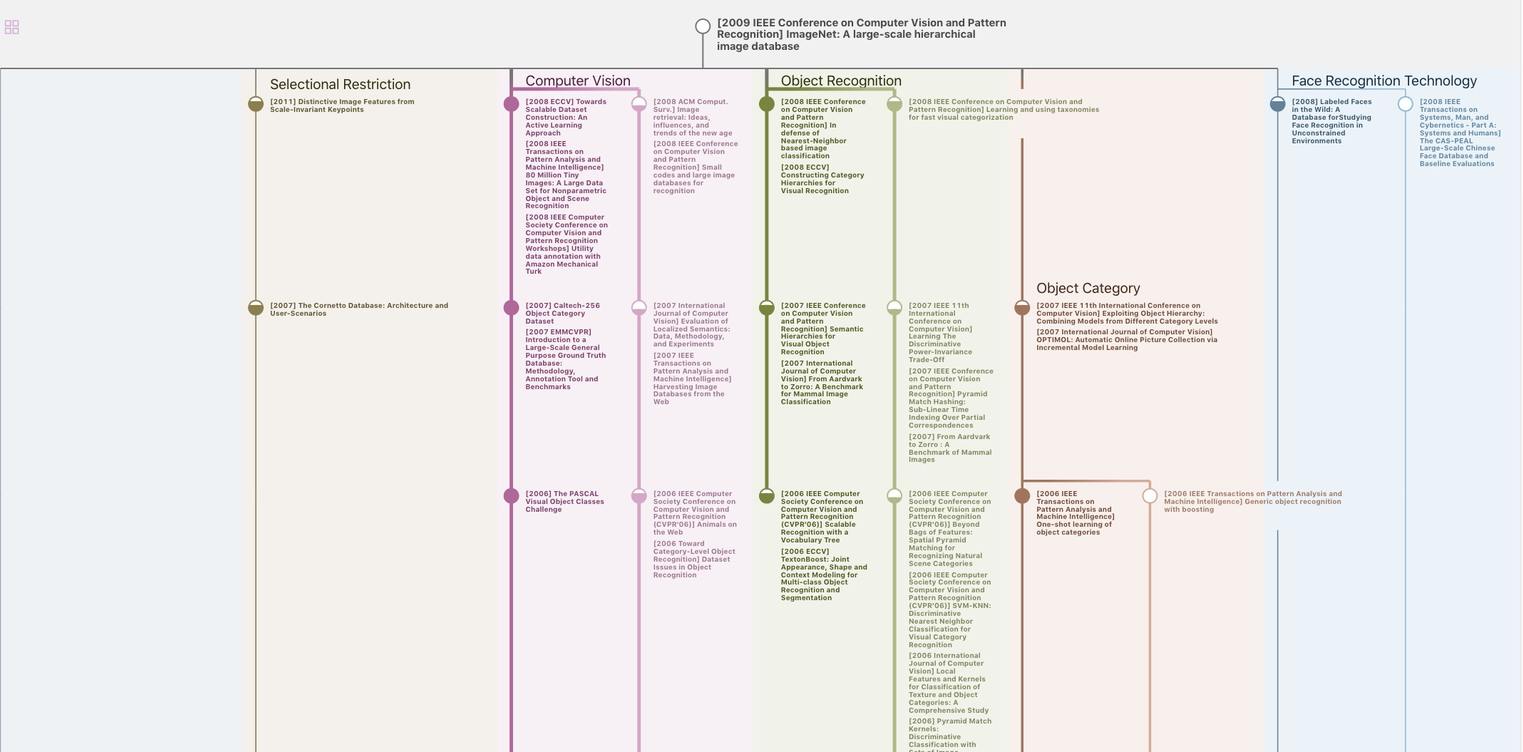
生成溯源树,研究论文发展脉络
Chat Paper
正在生成论文摘要