Channel Prediction over Irregular Terrains: Deep Autoencoder with Random Forest
2022 IEEE 23rd International Workshop on Signal Processing Advances in Wireless Communication (SPAWC)(2022)
摘要
Channel modeling is critical for coverage prediction, system level simulations, and wireless propagation characterization. Industry practice applies linear fit to the pathloss in decibels against the logarithm of the distance. Simple linear fit, however, cannot fully capture the shadowing effects in the channel, especially for a link with rich scatterings such as non-line-of-sight (NLOS) links in a complex propagation environment. In this paper, we propose an interpretable hybrid learning model with expert knowledge to predict the channel pathloss in desert-like environment using terrain profiles. We apply an autoencoder to extract compressed information from terrain profiles. The compressed representation of terrain, combined with features selected based on expert knowledge such as LOS/NLOS indicator and curvature of the terrain, are used to predict the pathloss. We show that a Random Forest regression model outperforms CNN/DNN models in generalizability of predicting unseen data by training and testing in disjoint sectors of the measured areas.
更多查看译文
关键词
pathloss prediction,autoencoder,random forest
AI 理解论文
溯源树
样例
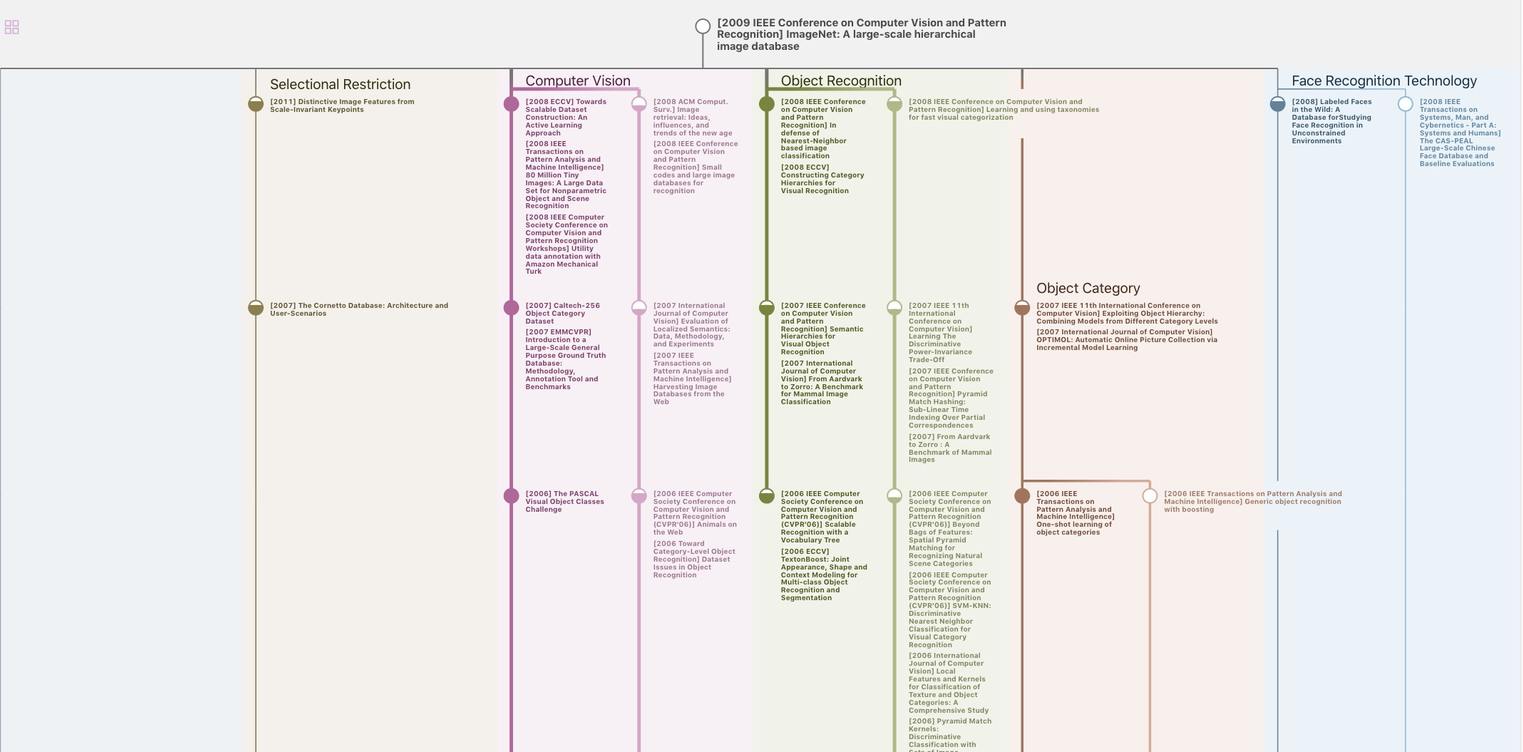
生成溯源树,研究论文发展脉络
Chat Paper
正在生成论文摘要