Local Control is All You Need: Decentralizing and Coordinating Reinforcement Learning for Large-Scale Process Control
2022 61st Annual Conference of the Society of Instrument and Control Engineers (SICE)(2022)
摘要
Deep reinforcement learning (RL) approaches are an appealing alternative to conventional controllers in process industries as such methods are inherently flexible and have generalization abilities to unseen situations. Namely, they alleviate the need for constant parameter tuning, tedious design of control laws, and re-identification procedures in the event of performance degradation. However, it remains challenging to apply RL to real-world process tasks, which commonly feature large state-action spaces and complex dynamics. Such tasks may be difficult to solve due to computational complexity and sample insufficiency. To tackle these limitations, we present a sample-efficient RL approach for large-scale control that expresses the global policy as a collection of local policies. Every local policy receives local observations and is responsible for controlling a different region of the environment. In order to enable coordination among local policies, we present a mechanism based on action sharing and message passing. The model is evaluated on a set of robotic tasks and a large-scale vinyl acetate monomer (VAM) plant. The experiments demonstrate that the proposed model exhibits significant improvements over baselines in terms of mean scores and sample efficiency.
更多查看译文
关键词
deep reinforcement learning,large-scale reinforcement learning,chemical process control
AI 理解论文
溯源树
样例
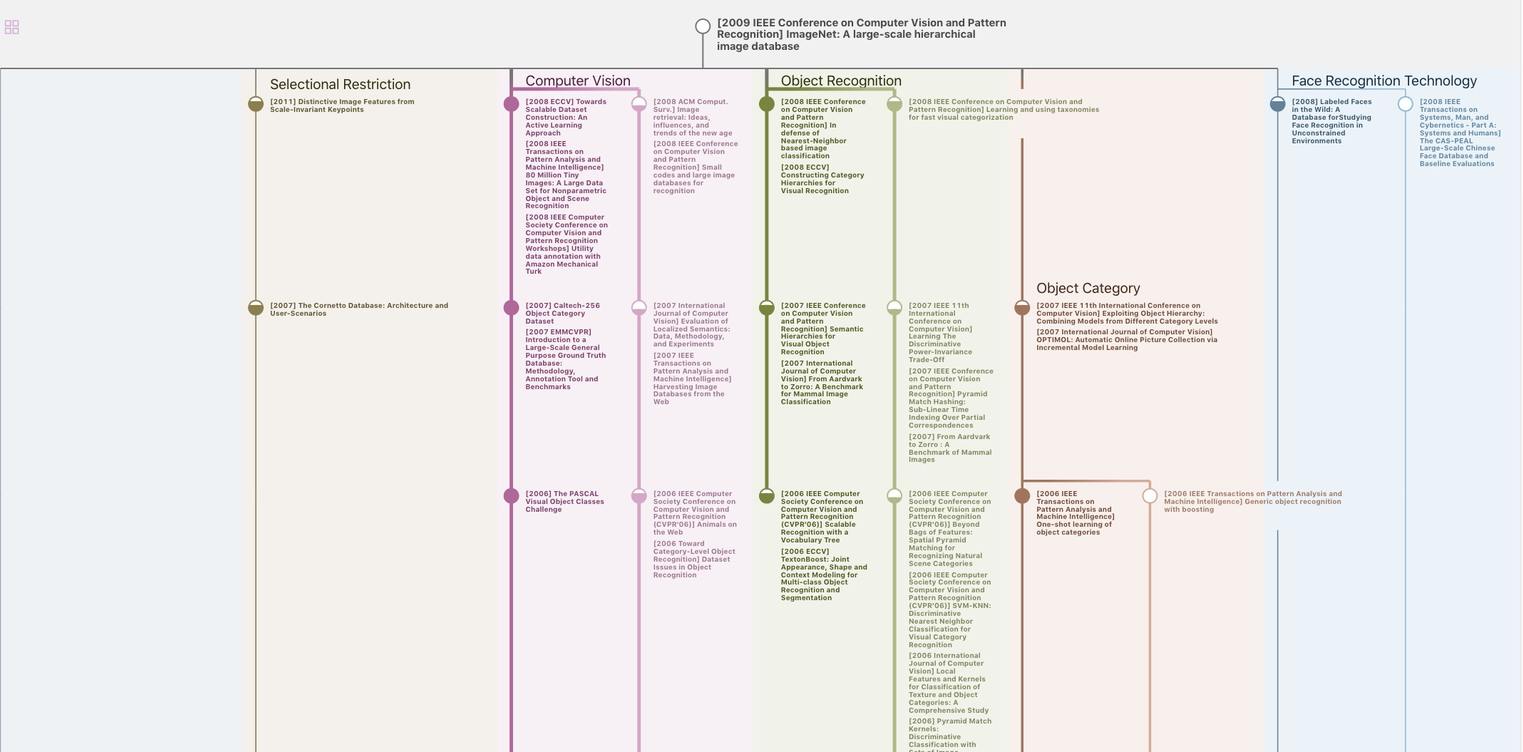
生成溯源树,研究论文发展脉络
Chat Paper
正在生成论文摘要