A Lightweight General Adaptive Optimization Tool for Relational DBMSs under HTAP Workloads
2022 IEEE International Conference on Services Computing (SCC)(2022)
摘要
It is well-known challenging to operate data-intensive applications on RDBMS(Relational DBMS) because the static storage model does not perform well for real-time processing queries and historical analyses. Existing HTAP systems pursue the robustness and functional completeness of the system excessively, which leads to over-design in the scenario where OLAP and OLTP alternate instead of coming simultaneously. Therefore, we present a lightweight general and adaptive optimization tool for RDBMS to address this issue. By deep learning-based workload forecasting, our tool optimizes the storage model continuously and ensures that the database is always at its best when handling coming HTAP requests. It differs from previous methods in that it adaptively optimizes existing databases as a plug-in but not with intrusive programming. Through experimental validation, our tool has an average performance improvement of 13.1% compared with static row-store RDBMS and 45.3% compared with static column-store RDBMS under a typical HTAP workload. The validation result illustrates that our tool makes RDBMSs more efficient in handling HTAP workloads at a tiny cost.
更多查看译文
关键词
Database Workload,Workload Forecasting,Data Storage Model,Adaptive Optimization
AI 理解论文
溯源树
样例
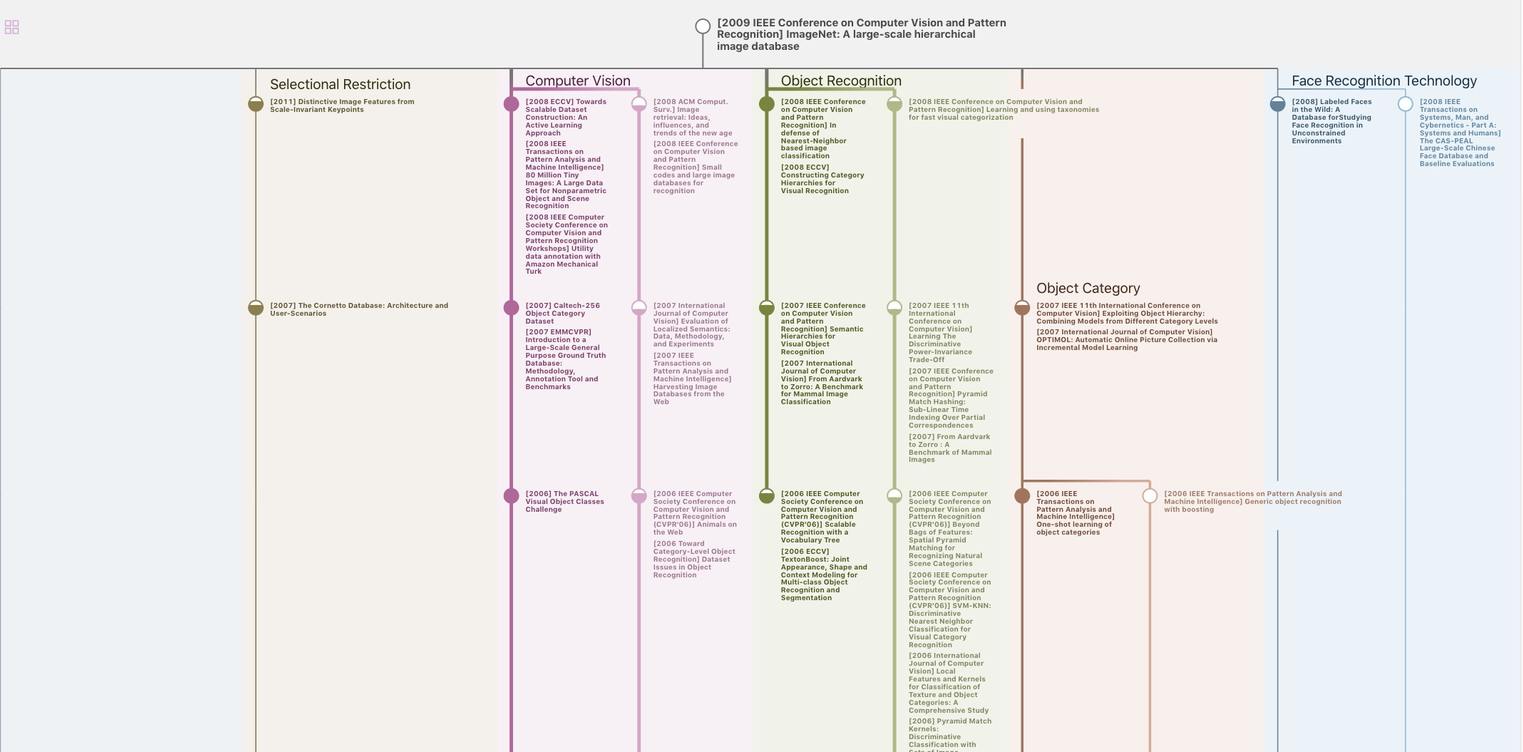
生成溯源树,研究论文发展脉络
Chat Paper
正在生成论文摘要