Machine Learning-Based Prediction for Time Series Damage Evolution of Ni-Based Superalloy Microstructures
Social Science Research Network(2022)
摘要
The Directionally Solidified (DS) superalloy DZ125 developed time-series related microstructure damage with serve time. The conventional grey prediction model (GM (1,1)), the fractional order accumulation grey pre-diction model (FAGM (1,1)), and the long short-term memory (LSTM) neural network prediction model were used to forecast the values of the time-related damage variables. The damage variables of the superalloy microstructure evolution were obtained through experimental observations of the evolution characteristics of the gamma' precipitates and gamma matrix. The microstructure damage variables were predicted by three machine learning models, and the fatigue damage evolution mechanism of the superalloy was analyzed. It was found that the root mean square error of the three prediction models was 0.072, 0.001, and 0.008, respectively. Finally, a model to predict fatigue life was established based on the Chaboche fatigue damage theory, and the fatigue life was ob-tained using the damage variables predicted by machine learning. The results revealed that the fractional order cumulative grey model and the long short-term memory neural network prediction model were both more ac-curate, with the conventional grey model being better for exponentially small sample time series data.
更多查看译文
关键词
DZ125 superalloy,Long short-term memory neural network,Grey theory,Microstructure damage evolution,Fatigue life prediction
AI 理解论文
溯源树
样例
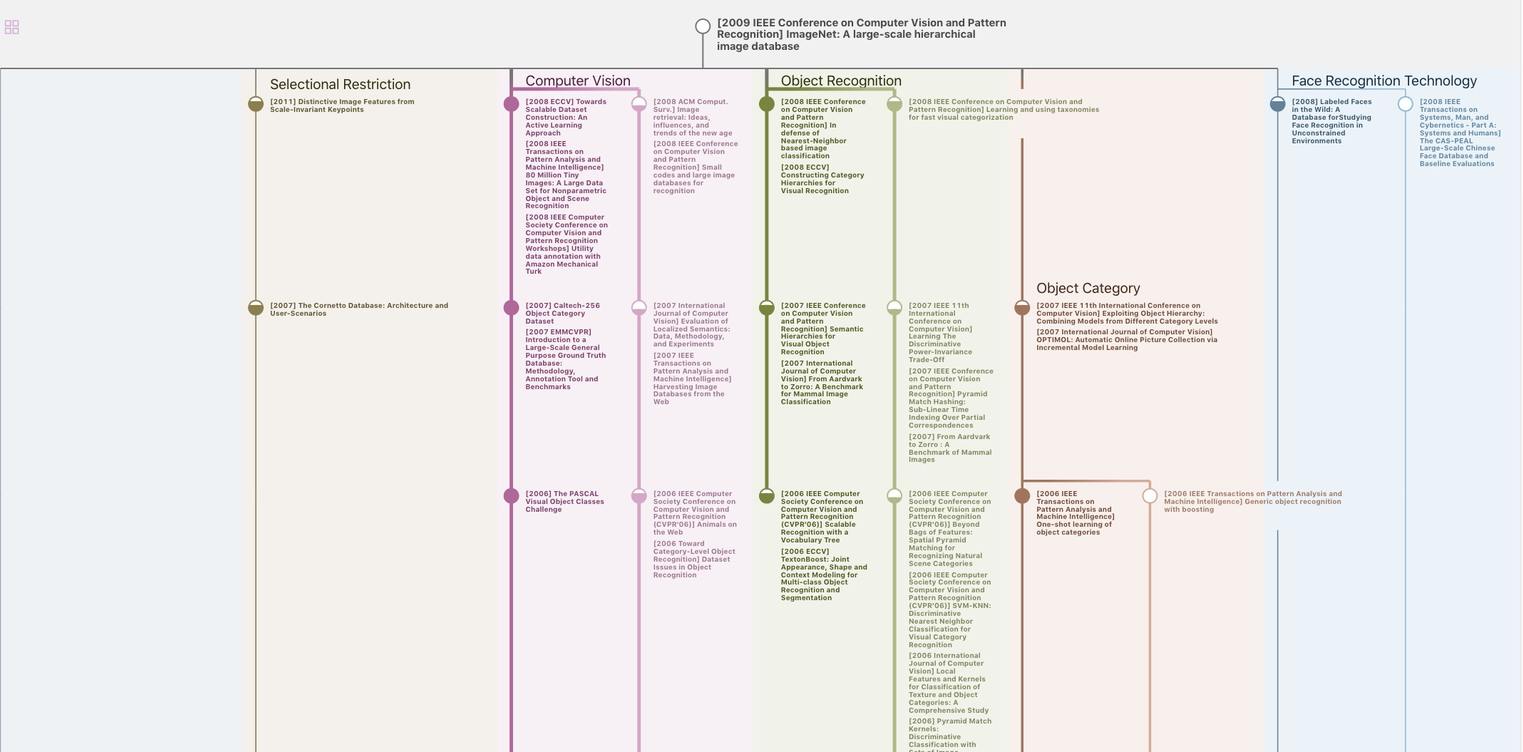
生成溯源树,研究论文发展脉络
Chat Paper
正在生成论文摘要