DeepViFi: detecting oncoviral infections in cancer genomes using transformers
Bioinformatics, Computational Biology and Biomedicine(2022)
摘要
BSTRACTWe consider the problem of identifying viral reads in human host genome data. We pose the problem as open-set classification as reads can originate from unknown sources such as bacterial and fungal genomes. Sequence-matching methods have low sensitivity in recognizing viral reads when the viral family is highly diverged. Hidden Markov models have higher sensitivity but require domain-specific training and are difficult to repurpose for identifying different viral families. Supervised learning methods can be trained with little domain-specific knowledge but have reduced sensitivity in open-set scenarios. We present DeepViFi, a transformer-based pipeline, to detect viral reads in short-read whole genome sequence data. At 90% precision, DeepViFi achieves 90% recall compared to 15% for other deep learning methods. DeepViFi provides a semi-supervised framework to learn representations of viral families without domain-specific knowledge, and rapidly and accurately identify target sequences in open-set settings.
更多查看译文
AI 理解论文
溯源树
样例
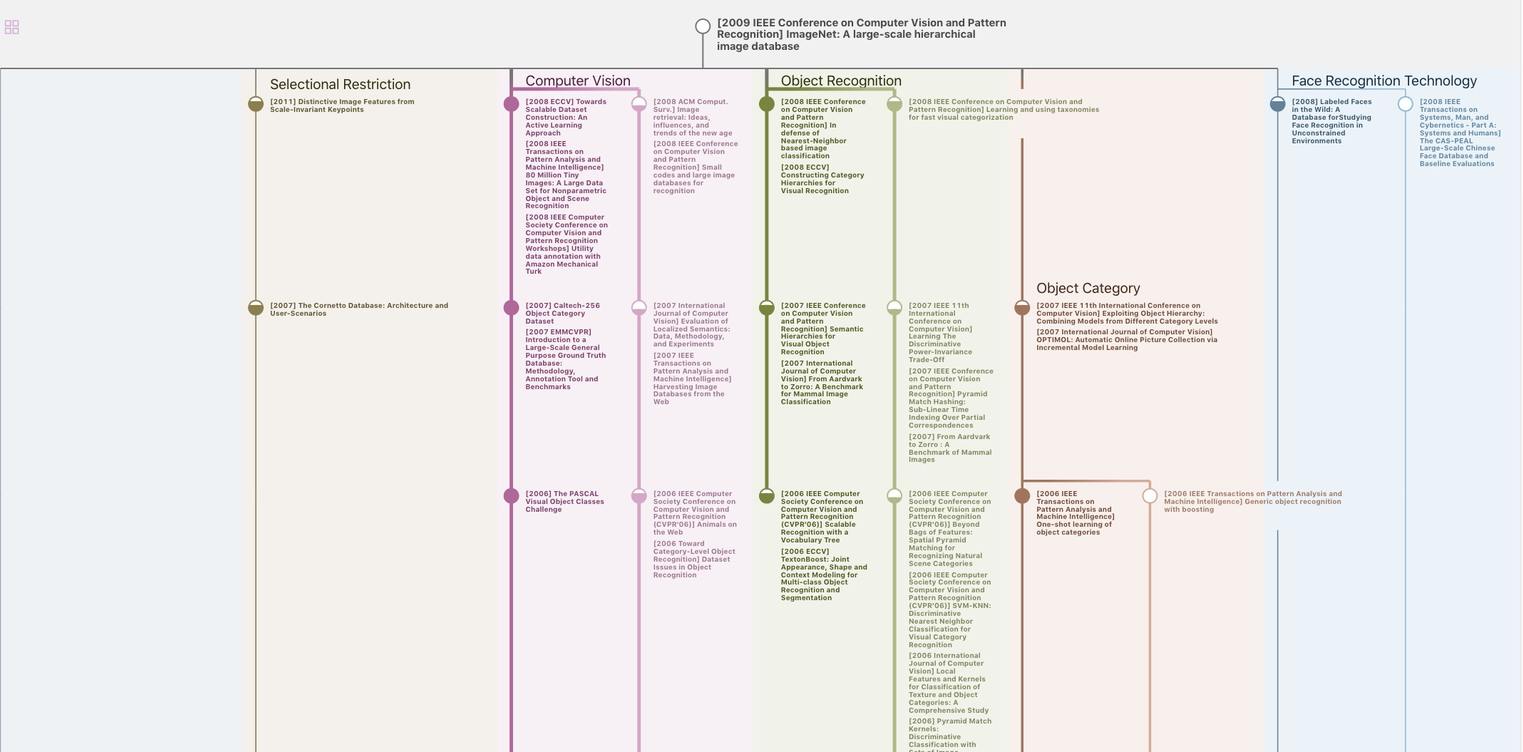
生成溯源树,研究论文发展脉络
Chat Paper
正在生成论文摘要