Multi-Objective Data-Driven Production Optimization with a Feedback Mechanism
IEEE Transactions on Industrial Informatics(2022)
摘要
Operation recommendations and automation for various industries rely on optimization over the top of the real-time Internet of Things data streams. Such recommendation applications have become imperative for industrial manufacturing, such as petroleum, chemicals, and food processing. We build a novel system of user-in-the-loop multiobjective optimization under the initial uncertainty of the optimization objectives, wherein the uncertainty is iteratively resolved via user feedback. We propose an interactive optimization system wherein both business and operational goals become defined as the optimization processes and where objective selection is incorporated as part of the optimization procedure. We show that such a solution exists during the iteration process if the feasibility space is not empty initially and is constrained by industry operations. Using an oil sands application, we demonstrate this approach and compare modeling results in values, response to business and operational priorities, and interpretability to the weighted sum optimization.
更多查看译文
关键词
Interpretability,linear weighted sum (LWS),mixed integer linear programming (MILP),multistage production,multistep optimization,optimization,production optimization
AI 理解论文
溯源树
样例
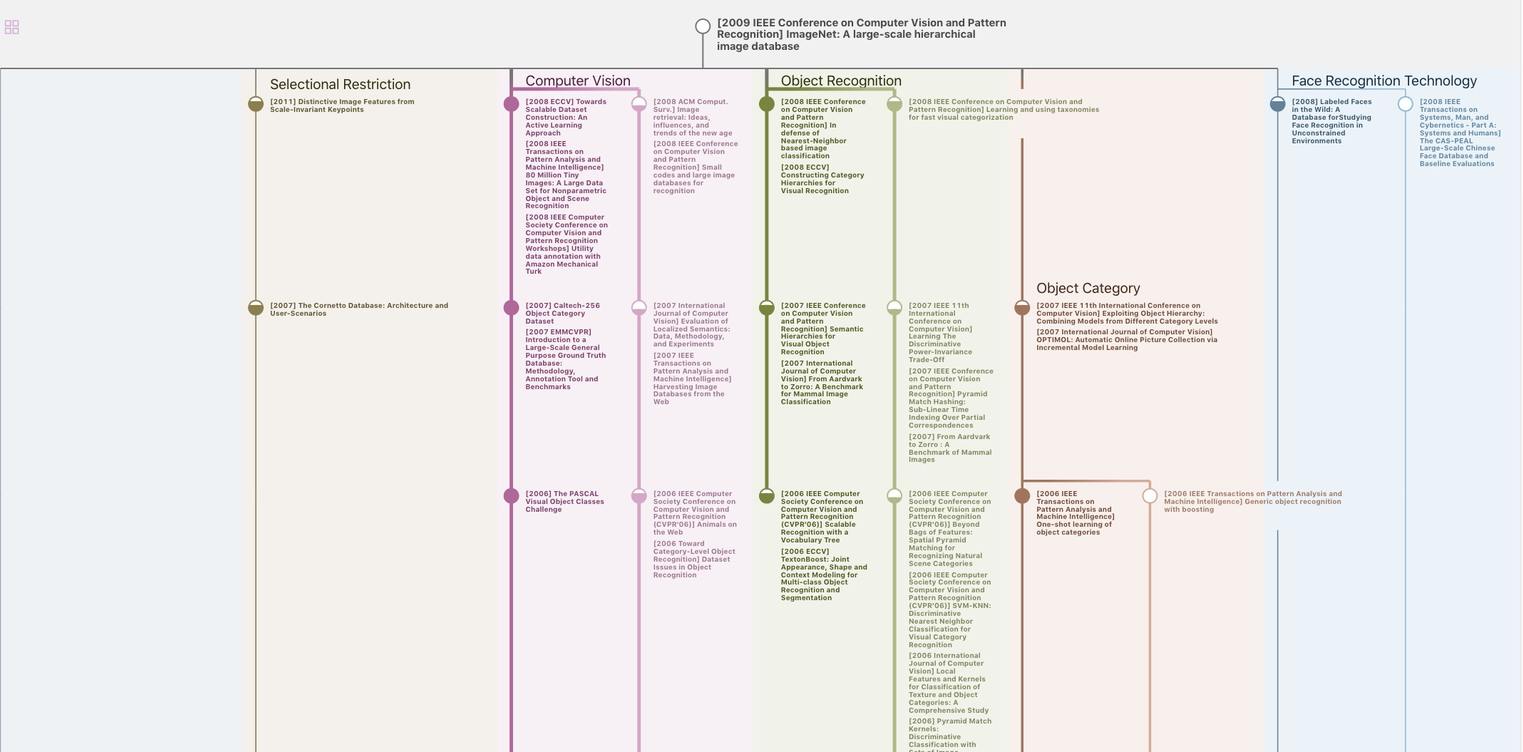
生成溯源树,研究论文发展脉络
Chat Paper
正在生成论文摘要