A novel semi-supervised learning approach for State of Health monitoring of maritime lithium-ion batteries
Social Science Research Network(2023)
摘要
Lithium-ion batteries are a prominent technology for the electrification of the transport sector, which itself is a key measure towards the departure from fossil fuels. The "green shift"is taking place in the marine industry too, where the number of battery-powered vessels is fastly growing. In this case, monitoring the battery State of Health is essential more than ever to optimise battery use, promote safety, and ensure the coverage of ship power and energy demands. Classification societies typically require annual capacity tests for this purpose; however, the tests are disruptive, costly and time-consuming. As a consequence they are seldom, in addition to not being always fully reliable. We propose a novel alternative semi-supervised learning approach to estimate the State of Health of a lithium-ion battery system with no labelled data, starting from a minimal set of weakly labelled data from another similar system. The method is based on operational sensor data gathered from the battery, together with the battery State of Charge. Our results show that the procedure is valid, and the obtained estimates can be used to significantly progress in failure prevention, operational optimisation, and for planning batteries at the design stage.
更多查看译文
关键词
State of Health monitoring,Maritime battery systems,Data-driven modelling,Semi-supervised learning,Multivariable Fractional Polynomial regression
AI 理解论文
溯源树
样例
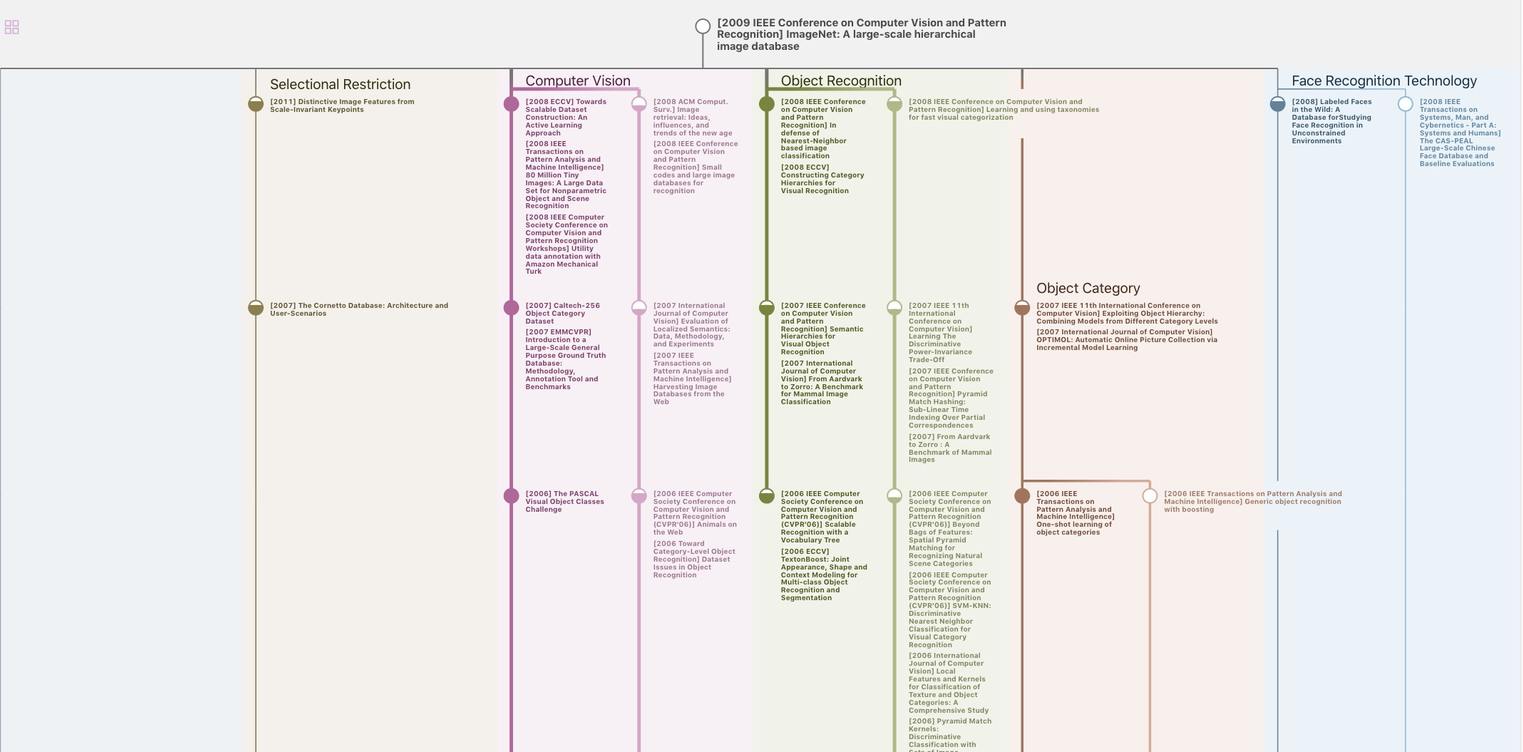
生成溯源树,研究论文发展脉络
Chat Paper
正在生成论文摘要