Cross-scenario transfer diagnosis of reciprocating compressor based on CBAM and ResNet
JOURNAL OF INTELLIGENT & FUZZY SYSTEMS(2022)
摘要
To address data distribution discrepancy across scenarios, deep transfer learning is used to help the target scenario complete the recognition task using similar scenario data. However, fault misrecognition or low diagnostic accuracy occurs due to the weak expression of the deep transfer model in cross-scenario application. The Convolutional Block Attention Module (CBAM) can independently learn the importance of each channel and space features, recalibrate the channel and space features, and improve image classification performance. This study introduces the CBAM module using the Residual Network (ResNet), and proposes a transfer learning model that combines the CBAM module with an improved ResNet, denoted as TL_CBAM_ResNet17. A miniature ResNet17 deep model is constructed based on the ResNet50 model. The location of the CBAM module embedded in the ResNet17 model is determined to strengthen model expression. For effective cross-scenario transfer and reduced data distribution discrepancy between source and target domains, a multi-kernel Maximum Mean Discrepancy (MK-MMD) layer is added in front of the classifier layer in the ResNet17 model to select data with common domain features. Considering a reciprocating compressor as the research object, cross-scenario datasets are produced by the vibration signals from the simulation test bench and simulation signals from the dynamic simulation model. Mutual transfer experiments are conducted using these datasets. The proposed method (TL_CBAM_ResNet17) demonstrates better classification performance than TCA, JDA, the TL_ResNet50_model, the TL_ResNet17_model, and the TL_ResNet17_model integrated with other attention mechanism module, and greatly improves the accuracy of fault diagnosis and generalization of the model in cross-scenario applications.
更多查看译文
关键词
Cross-scenario,transfer learning,reciprocating compressor,ResNet,CBAM,dynamic simulation
AI 理解论文
溯源树
样例
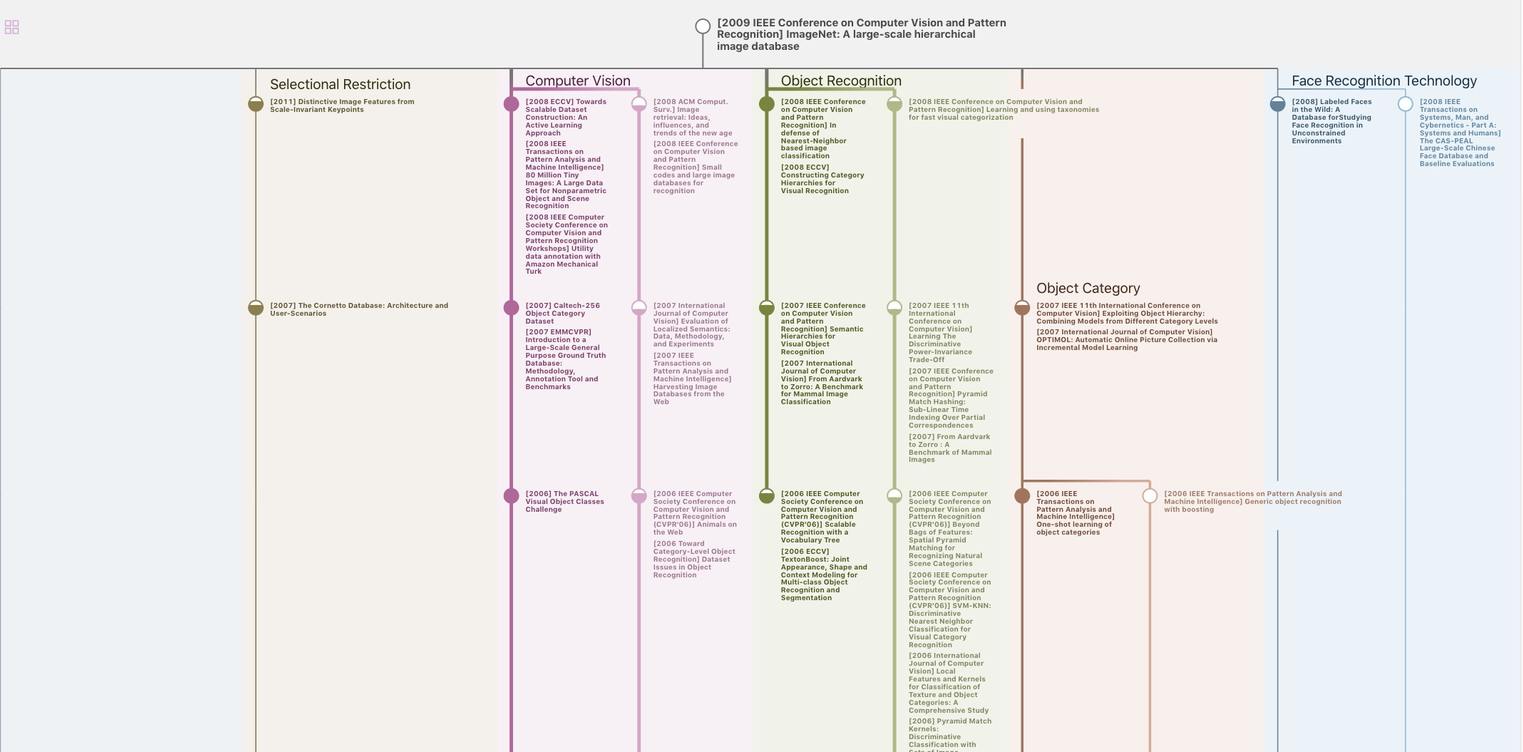
生成溯源树,研究论文发展脉络
Chat Paper
正在生成论文摘要