Comprehensive AI Model Development for Gleason Grading: From Scanning, Cloud-based Annotation to Pathologist-AI Interaction
Social Science Research Network(2022)
摘要
Abstract AI-based solutions for automated Gleason grading have been developed to assist pathologists to make rapid and quantitative assessments, but the generalization across various scanners and updating AI models continuously using new annotated data from end users remains a key bottleneck in the field. We proposed an comprehensive digital pathology workflow for AI-assisted Gleason grading, incorporating an image quality check software A!magQC, a cloud-based annotation platform A!HistoNotes and Pathologist-AI Interaction (PAI) strategy. To demonstrate and validate the pipeline, we employed it on prostate samples obtained from 5 scanners for Gleason grading. After training on 132 prostatectomy specimens scanned by an Akoya Biosciences scanner, validation on 55 prostatectomy specimens and 156 biopsy specimens yielded a sensitivity of 85%, specificity of 96% and F1 score of 78% on Gleason grading for prostatectomy specimens, and 96% sensitivity on tumor detection for biopsy specimens. For images scanned by other 4 scanners, the average F1 score increased from 67% to 75% on Gleason pattern detection after adopting our generalization solution. In clinical experiments conducted with 5 pathologists from Singapore and China, our pipeline accelerated Gleason scoring by 43%. Furthermore, it reduced annotation time by 60% via semi-automatic annotation, leading to improved model performance through incremental learning.
更多查看译文
关键词
gleason grading,comprehensive ai model development,scanning,cloud-based
AI 理解论文
溯源树
样例
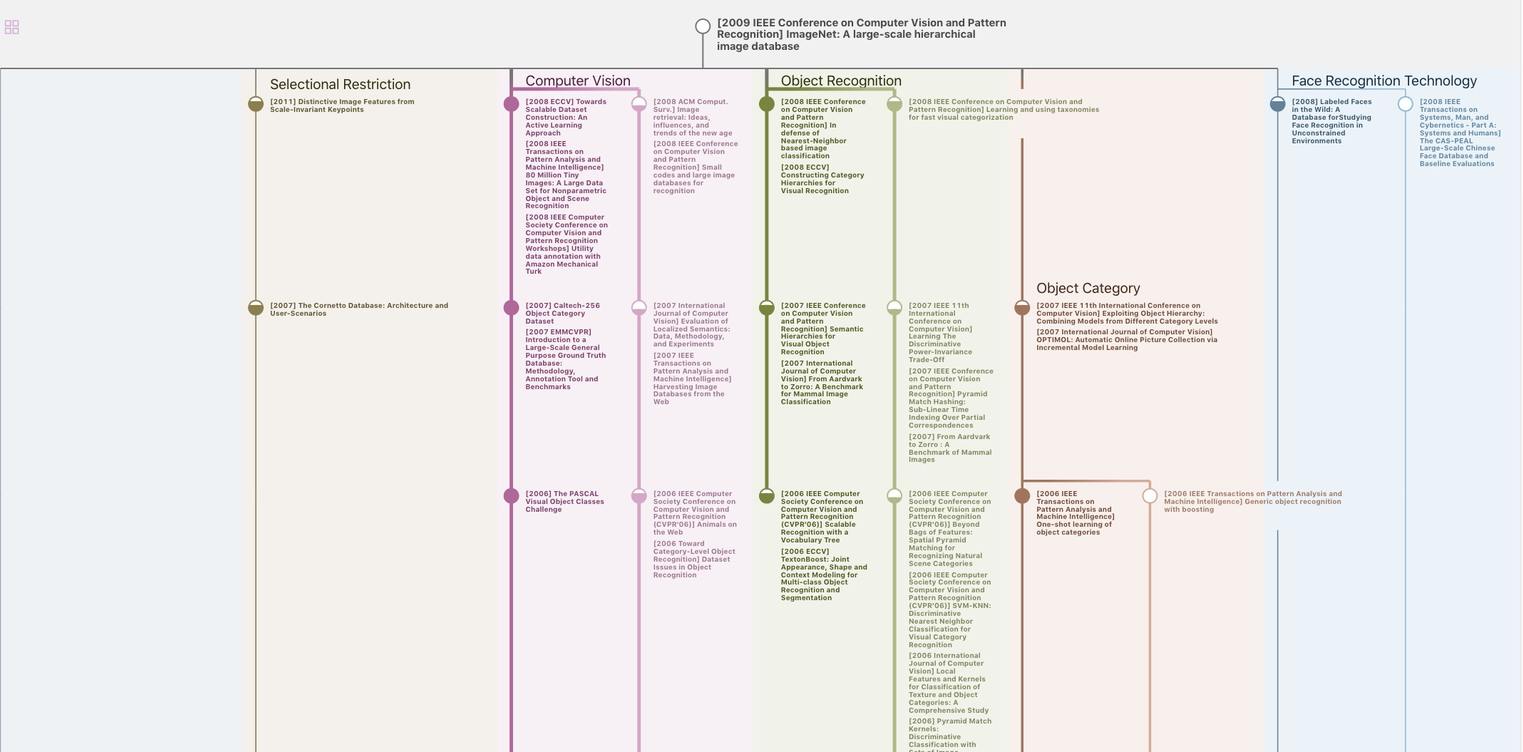
生成溯源树,研究论文发展脉络
Chat Paper
正在生成论文摘要