Smooth Trajectory Collision Avoidance Through Deep Reinforcement Learning
2022 21ST IEEE INTERNATIONAL CONFERENCE ON MACHINE LEARNING AND APPLICATIONS, ICMLA(2022)
摘要
Collision avoidance is a crucial task in vision-guided autonomous navigation. Solutions based on deep reinforcement learning (DRL) has become increasingly popular. In this work, we proposed several novel agent state and reward function designs to tackle two critical issues in DRL-based navigation solutions: 1) smoothness of the trained flight trajectories; and 2) model generalization to handle unseen environments. Formulated under a DRL framework, our model relies on margin reward and smoothness constraints to ensure UAVs fly smoothly while greatly reducing the chance of collision. The proposed smoothness reward minimizes a combination of first-order and second-order derivatives of flight trajectories, which can also drive the points to be evenly distributed, leading to stable flight speed. To enhance the agent's capability of handling new unseen environments, two practical setups are proposed to improve the invariance of both the state and reward function when deploying in different scenes. Experiments demonstrate the effectiveness of our overall design and individual components.
更多查看译文
关键词
Deep reinforcement learning,collision avoidance,UAV,smoothness,rewards
AI 理解论文
溯源树
样例
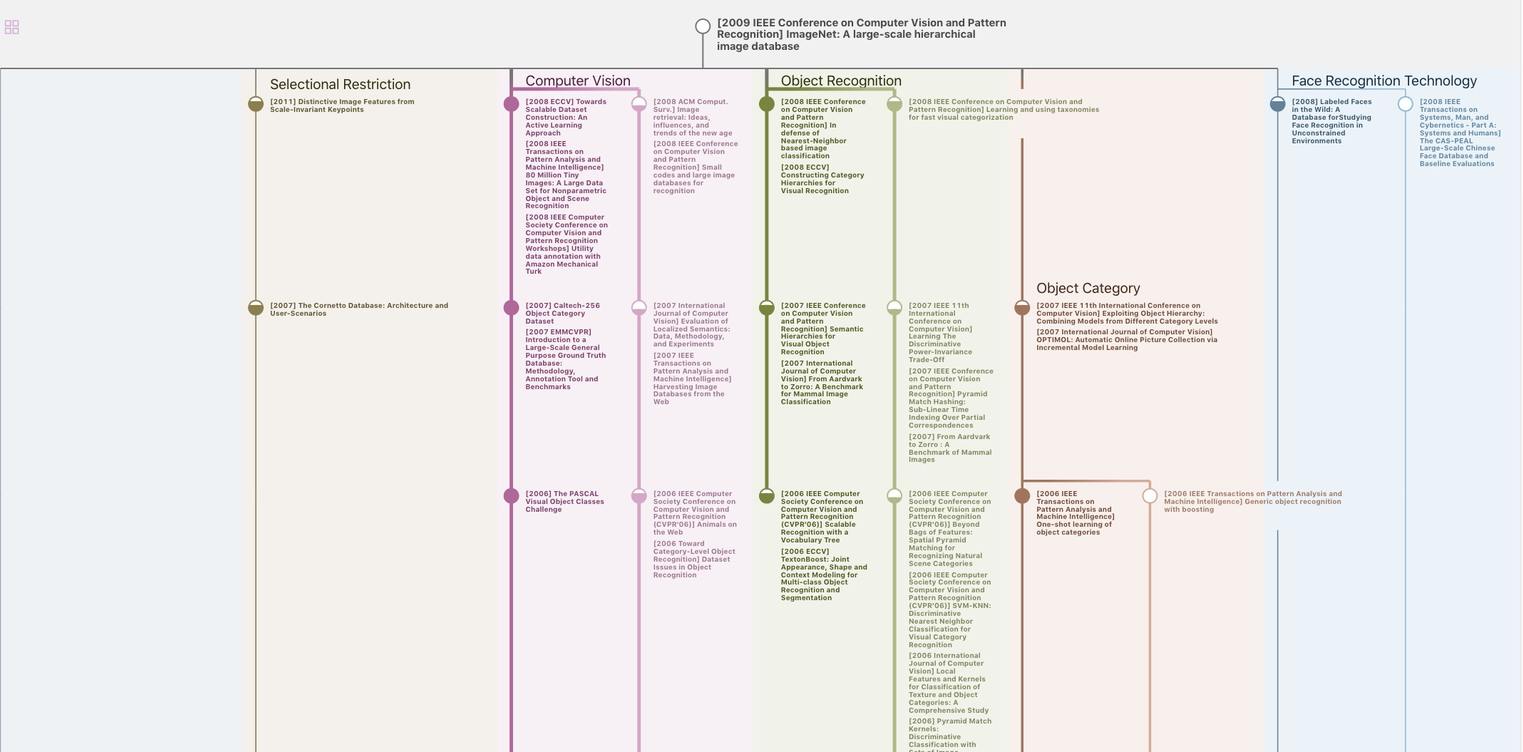
生成溯源树,研究论文发展脉络
Chat Paper
正在生成论文摘要