Machine Learning Models Are Superior to Non‐Invasive Tests in Identifying Clinically Significant Stages of NAFLD and NAFLD‐Related Cirrhosis
Hepatology(2022)
摘要
We assessed the performance of machine learning (ML) models in identifying clinically significant NAFLD-associated liver fibrosis and cirrhosis. Methods We implemented ML models including logistic regression (LR), random forests (RF), and artificial neural network (ANN) to predict histological stages of fibrosis using 17 demographic/clinical features in 1370 NAFLD patients who underwent liver biopsy, FibroScan®, and labs within a 6-month period at multiple US centers. Histological stages of fibrosis (≥F2, ≥F3, F4) were predicted using ML, FibroScan® liver stiffness measurements, and Fibrosis-4 index (FIB-4). NASH with significant fibrosis (NAS≥4+≥F2) was assessed using ML, FibroScan-AST (FAST) score, FIB-4, and NAFLD fibrosis score (NFS). We used 80% of the cohort to train and 20% to test the ML models.For ≥F2, ≥F3, F4, and NASH+NAS≥4+≥F2, all ML models, especially RF, had mainly higher accuracy and AUC compared to FibroScan®, FIB-4, FAST, and NFS. AUC for RF vs FibroScan® and FIB-4 for ≥F2, ≥F3, F4 were (0.86 vs 0.81, 0.78), (0.89 vs 0.83, 0.82) and (0.89 vs 0.86, 0.85), respectively. AUC for RF vs FAST, FIB-4, and NFS for NASH+NAS≥4+≥F2 were (0.80 vs 0.77, 0.66, 0.63). For NASH+NAS≥4+≥F2, all ML models had lower/similar percentages within the indeterminate zone compared to FIB-4 and NFS. Overall, ML models performed better in sensitivity, specificity, PPV, and NPV compared to traditional non-invasive tests.ML models performed better overall than FibroScan®, FIB-4, FAST, and NFS. ML could be an effective tool for identifying clinically significant liver fibrosis and cirrhosis in NAFLD patients.
更多查看译文
AI 理解论文
溯源树
样例
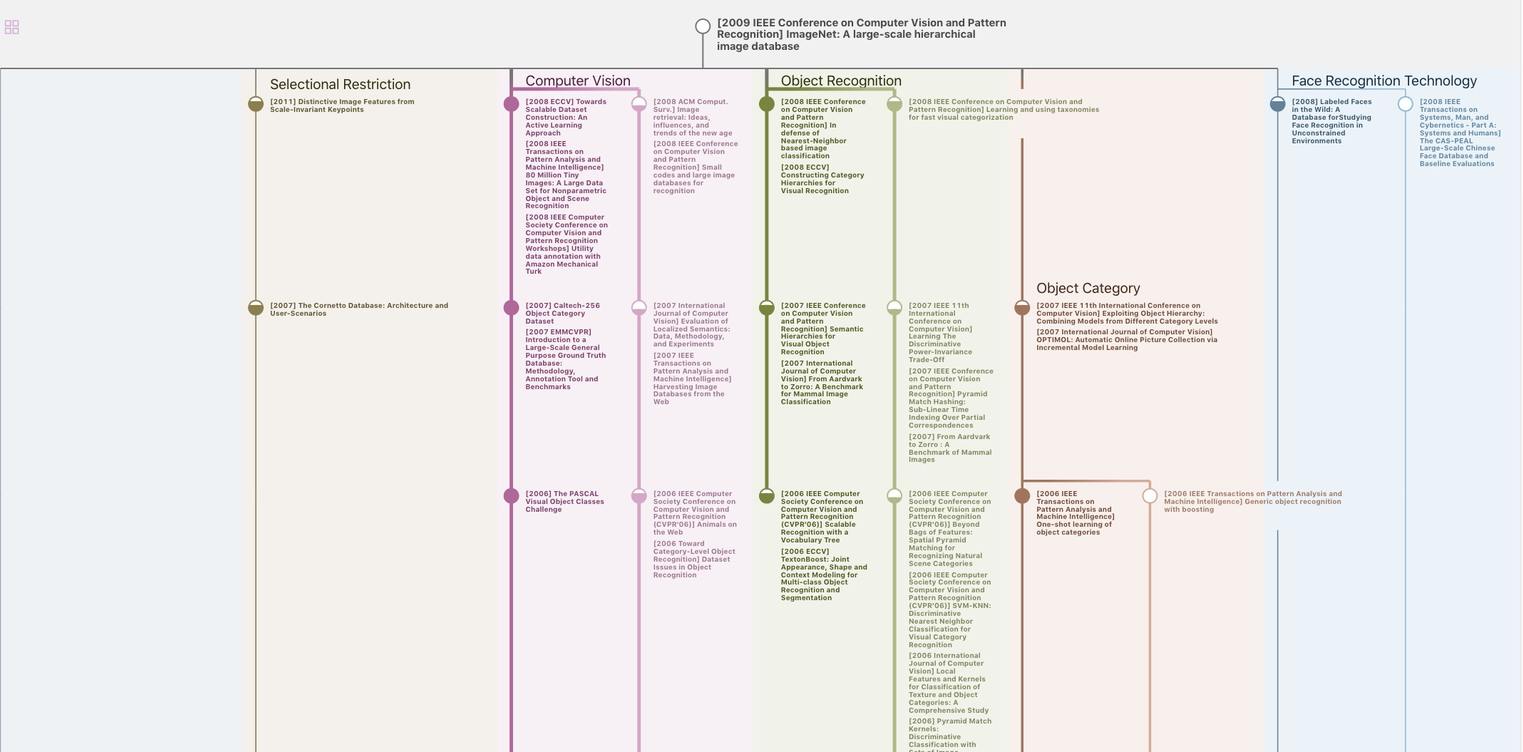
生成溯源树,研究论文发展脉络
Chat Paper
正在生成论文摘要