Patch-Based Training of Fully Convolutional Network for Hyperspectral Image Classification With Sparse Point Labels
IEEE JOURNAL OF SELECTED TOPICS IN APPLIED EARTH OBSERVATIONS AND REMOTE SENSING(2022)
摘要
Fully convolutional network (FCN), which has excellent capability for capturing spatial context, was introduced to improve the performance of hyperspectral image classification (HSIC). However, training FCN usually requires a huge amount of pixel-level labels, which is difficult to obtain for HSIC in practical applications. How to train an FCN effectively with the supervision of limited sparse point labels has attracted the attention. The patch-free training pattern with sparse point labels was proved to be effective for HSIC task. Then, as a general training mode for remote sensing image semantic segmentation, is patch-based training of FCN also effective for HSIC with sparse point labels? To answer this question, a patch-based training framework with a novel fully convolutional network is proposed for HSIC in this article. First, cropped hyperspectral image (HSI) patches with sparse labels are input for training. Second, considering the limitation of supervision with sparse points for training, a lightweight network on the basis of an encoder-decoder structure with shallow channels is specially designed for HSIC with the aid of residual connections in the encoder and the integration of multiple attention modules to fully exploit the spectral-spatial information of HSI. Third, conditional random field-loss is adopted as a prior complement to the point supervision for further excavation of spatial context information. The performance of the proposed method is quantitatively evaluated on three HSI datasets and achieves state-of-the-art performance in comparison with other representative methods, demonstrating the effectiveness of the patch-based training framework for HSIC.
更多查看译文
关键词
Training,Feature extraction,Hyperspectral imaging,Logic gates,Convolutional neural networks,Decoding,Task analysis,Convolutional neural network,fully convolutional network,hyperspectral image classification,patch-based training,point label
AI 理解论文
溯源树
样例
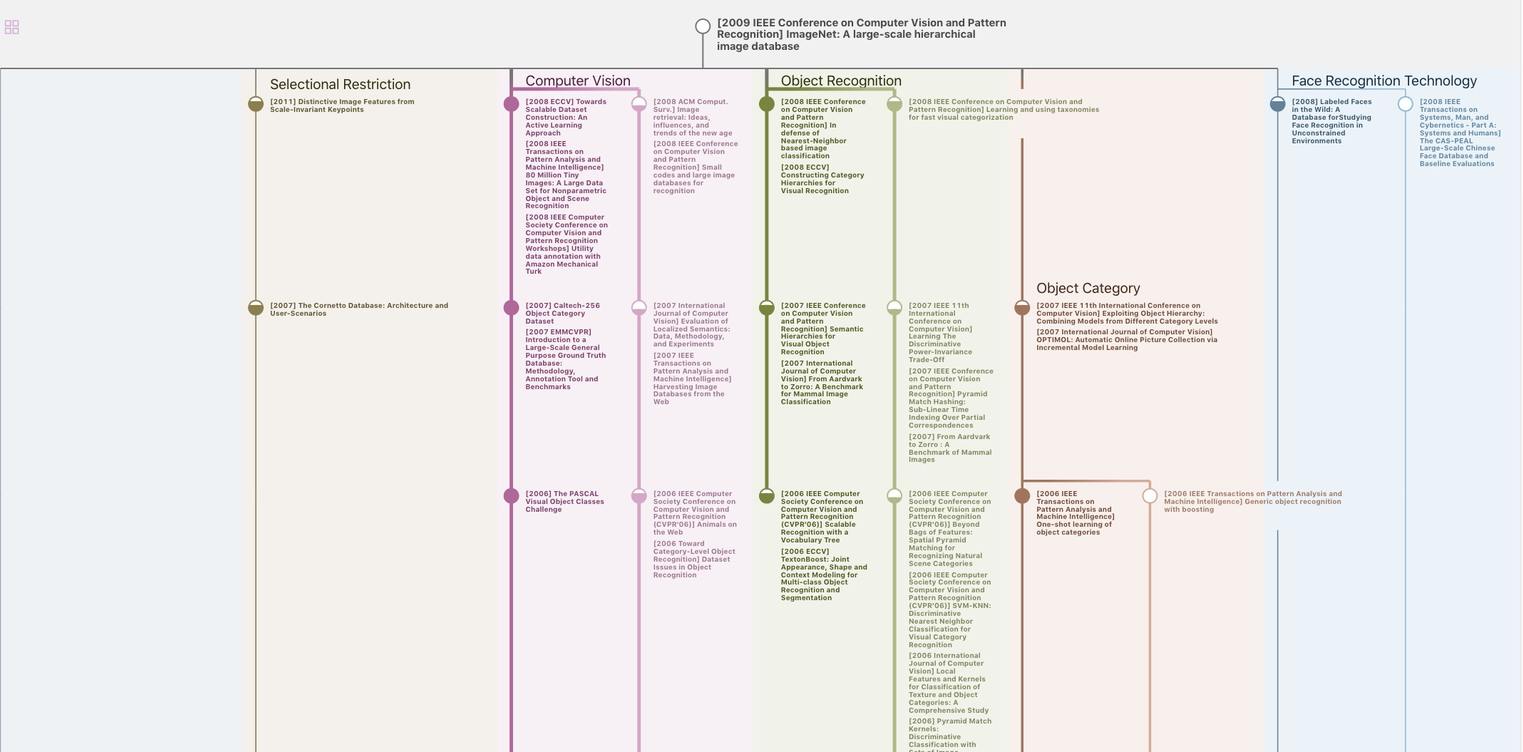
生成溯源树,研究论文发展脉络
Chat Paper
正在生成论文摘要