Multi-omic modeling of antidepressant response implicates dynamic immune and inflammatory changes in individuals who respond to treatment
biorxiv(2022)
摘要
Major depressive disorder (MDD) is a leading cause of disability worldwide, and is commonly treated with antidepressant drugs (AD). Although effective, many patients fail to respond to AD treatment, and accordingly identifying factors that can predict AD response would greatly improve treatment outcomes. In this study, we developed a machine learning tool to integrate multi-omic datasets (gene expression, DNA methylation, and genotyping) to identify biomarker profiles associated with AD response in a cohort of individuals with MDD. To address this rich multi-omic dataset with high dimensional features, we developed integrative Geneset-Embedded non-negative Matrix factorization (iGEM), a non-negative matrix factorization (NMF) based model, supplemented with auxiliary information regarding genesets and gene-methylation relationships. Using our model, we identified a number of meta-phenotypes which were related to AD response. By integrating geneset information into the model, we were able to relate these meta-phenotypes to biological processes, including immune and inflammatory functions. This represents both biomarkers to predict response, as well as potential new treatment targets. Our method is applicable to other diseases with multi-omic data, and the software is open source and available on Github ().
### Competing Interest Statement
The authors have declared no competing interest.
更多查看译文
关键词
antidepressant response,inflammatory changes,multi-omic
AI 理解论文
溯源树
样例
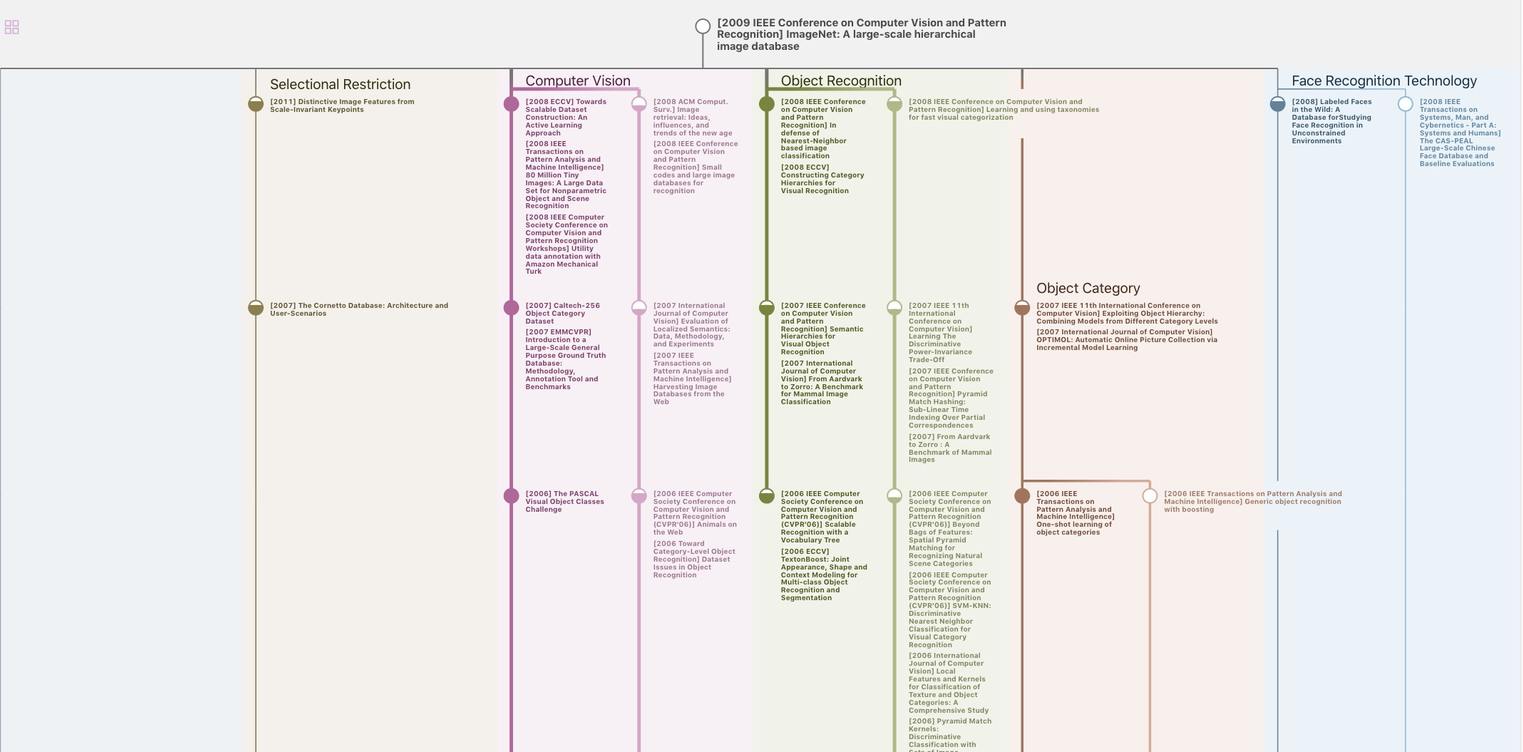
生成溯源树,研究论文发展脉络
Chat Paper
正在生成论文摘要