A Neural Network Based Data Imputation Model for Missing Data of Rail Corrugation Measurement
2021 IEEE 5th Information Technology,Networking,Electronic and Automation Control Conference (ITNEC)(2021)
摘要
The measurement data of rail corrugation is an important data source used by railway maintenance departments to monitor the safety of running rails. The accuracy of rail corrugation measurement and the integrity of data play a great decisive role in the strategy of rail maintenance. However, due to the complex measurement environment, the missing of rail corrugation measurement data is common problem for dynamic measurement. In order to solve the negative impact of data loss on the accuracy of the overall measurement data, a network model based on improved LSTM (Bi-LSTM) neural network is proposed to fill the missing data. The network model is trained by using the historical rail corrugation data, and the missing data of the measurement data of the laser displacement sensor is filled by the trained Bi-LSTM model. The experimental results effectively prove the effectiveness and accuracy of the data imputation method proposed in this paper, and the performance is better than some state-of-art prediction models. Furthermore, the extensive experiments also prove the proposed data imputation method has high robustness and can still maintain high filling accuracy in the case of high loss rate.
更多查看译文
关键词
Rail Corrugation,Missing data,Data imputation,Neural Network,LSTM,Bi-LSTM
AI 理解论文
溯源树
样例
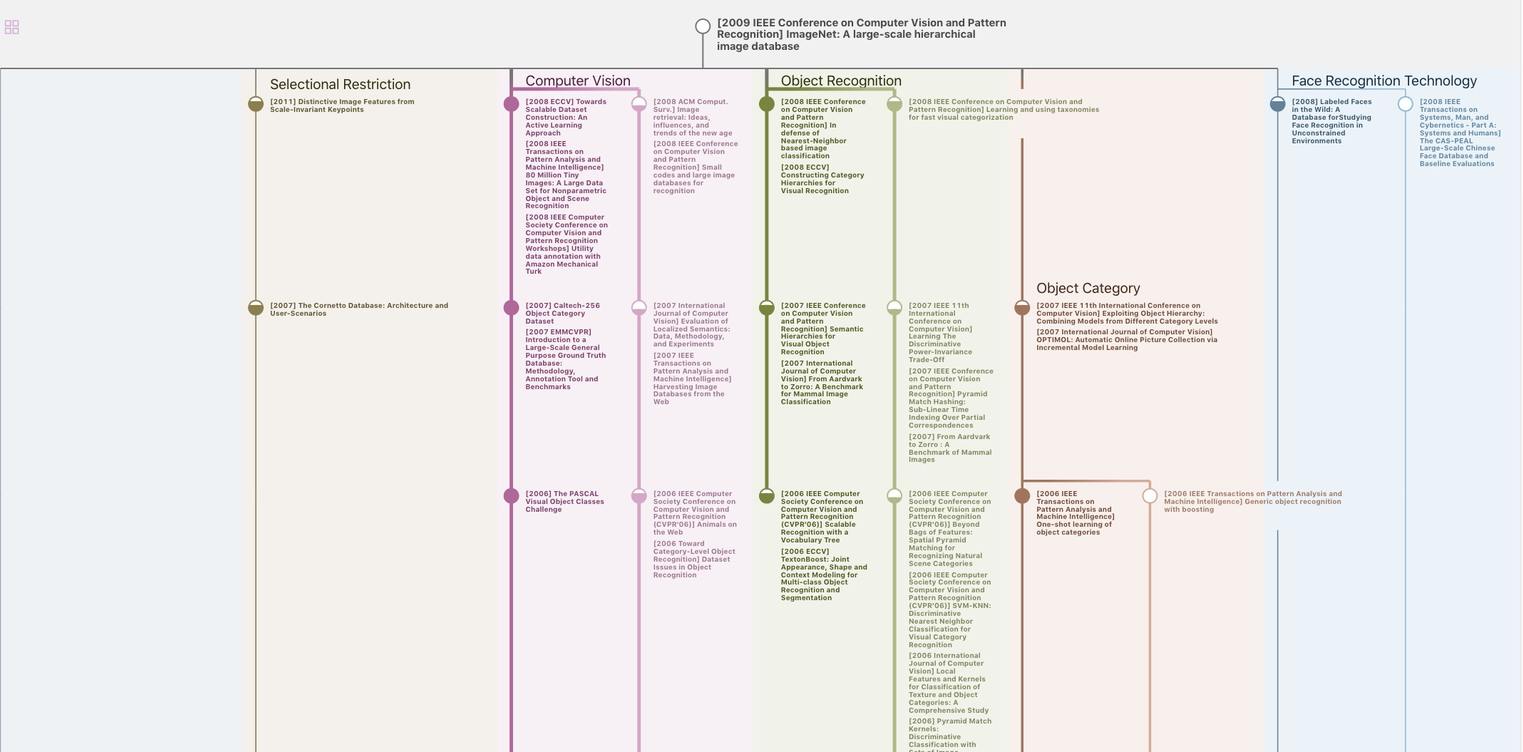
生成溯源树,研究论文发展脉络
Chat Paper
正在生成论文摘要