A Novel Machine Learning Classification Model for Human Brain States Based on Spatio-Temporal Spectral Profile: An Example of Eyes-Open/Eyes-Closed fMRI Data
2021 International Conference on Electronic Information Engineering and Computer Science (EIECS)(2021)
摘要
The decoding of human brain activity based on functional Magnetic Resonance Imaging (fMRI) technology has received wide attention nowadays, but there are some challenges in decoding brain states due to some limitations such as low signal-to-noise ratio of fMRI signal, complex cognition process, high dimensionality, non-linearity, etc. In this study, we proposed a machine learning classification model based on spatio-temporal spectral profile (STSP) features from fMRI data to better decode the states of human brain activity. The key steps were as follows: (1) fMRI data of all subjects were divided into two categories according to state one and state two; next, the STSP feature extraction technique was used to reduce the complexity of fMRI data from spatio-temporal dimension to spectral dimension. An STSP matrix for each subject was generated while preserving the main features of the original data. (2) Max-Relevance and Min-Redundancy (mRMR) or two-sample t-test on STSP matrices were used to screen out the most discriminative features between two states. (3) After that, four alternative classifiers, i.e., Support Vector Machine (SVM), Random Forest (RF), Naive Bayesian Classifier (NBC), and K-Nearest Neighbors (KNN), were applied to train and test data for classification. Finally, a validated classification study of brain activity states was conducted on the Eyes-Open and Eyes-Closed (EO-EC) dataset, and the results showed that mRMR feature screening combined with SVM could achieve the best classification results of brain states compared with other classifiers.
更多查看译文
关键词
fMRI,Spatio-temporal spectral profile,Max-Relevance and Min-Redundancy,Machine Learning
AI 理解论文
溯源树
样例
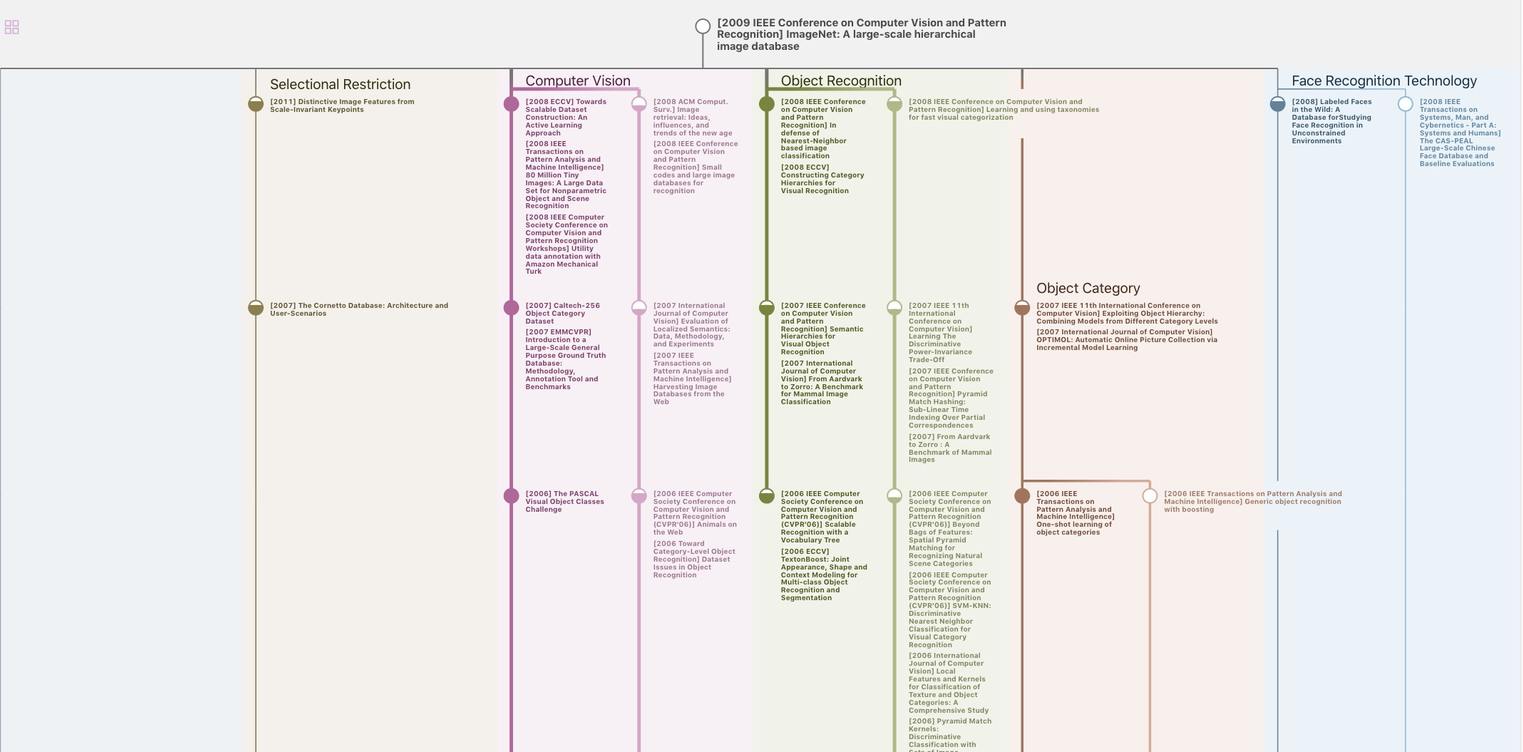
生成溯源树,研究论文发展脉络
Chat Paper
正在生成论文摘要