Domain Adaptation via Maximizing Surrogate Mutual Information
arXiv: Learning(2021)
摘要
Unsupervised domain adaptation (UDA) aims to predict unlabeled data from target domain with access to labeled data from the source domain. In this work, we propose a novel framework called SIDA (Surrogate Mutual Information Maximization Domain Adaptation) with strong theoretical guarantees. To be specific, SIDA implements adaptation by maximizing mutual information (MI) between features. In the framework, a surrogate joint distribution models the underlying joint distribution of the unlabeled target domain. Our theoretical analysis validates SIDA by bounding the expected risk on target domain with MI and surrogate distribution bias. Experiments show that our approach is comparable with state-of-the-art unsupervised adaptation methods on standard UDA tasks.
更多查看译文
关键词
domain adaptation,surrogate mutual information
AI 理解论文
溯源树
样例
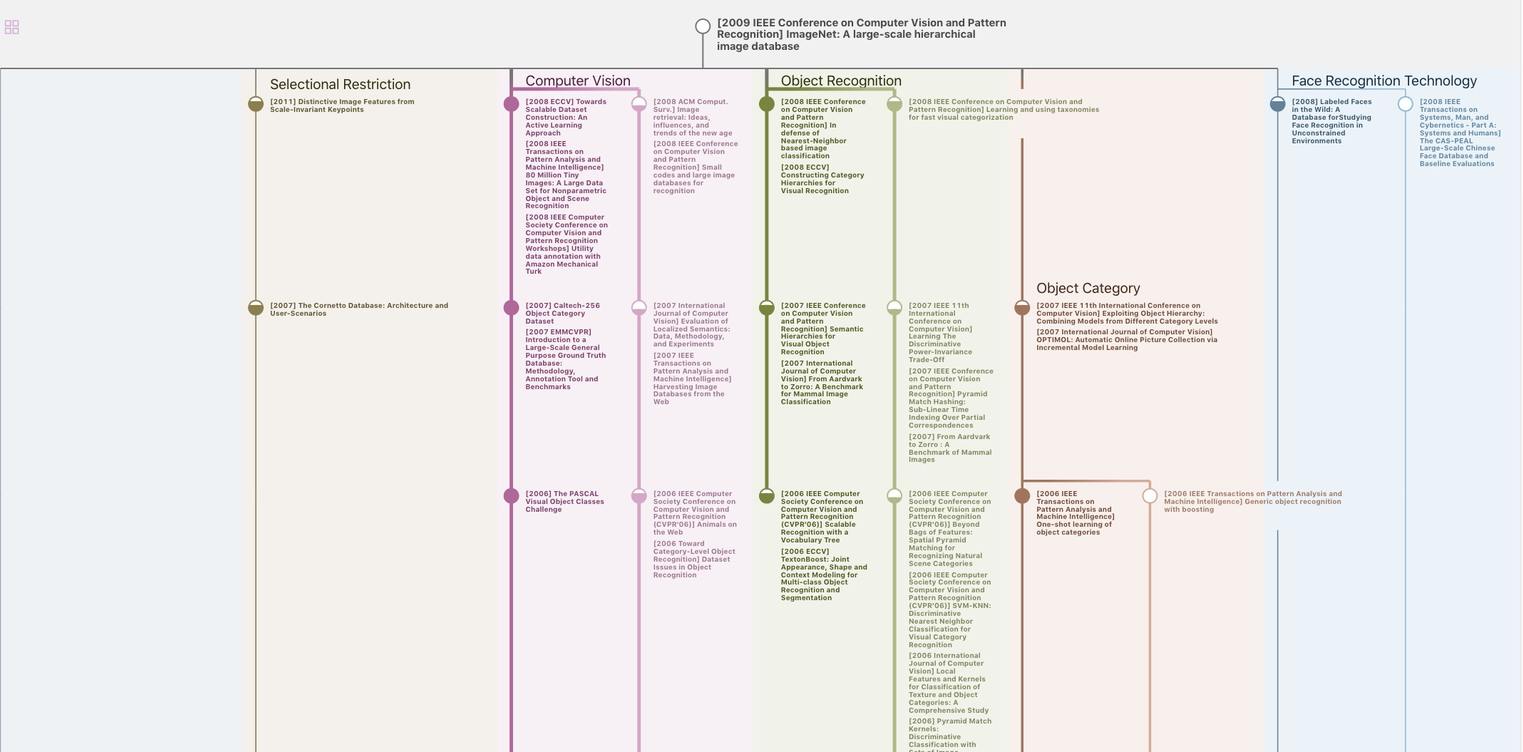
生成溯源树,研究论文发展脉络
Chat Paper
正在生成论文摘要