Proposition-Level Clustering for Multi-Document Summarization
Proceedings of the 2022 Conference of the North American Chapter of the Association for Computational Linguistics: Human Language Technologies(2022)
摘要
Text clustering methods were traditionally incorporated into multi-document summarization (MDS) as a means for coping with considerable information repetition. Particularly, clusters were leveraged to indicate information saliency as well as to avoid redundancy. Such prior methods focused on clustering sentences, even though closely related sentences usually contain also non-aligned parts. In this work, we revisit the clustering approach, grouping together sub-sentential propositions, aiming at more precise information alignment. Specifically, our method detects salient propositions, clusters them into paraphrastic clusters, and generates a representative sentence for each cluster via text fusion. Our summarization method improves over the previous state-of-the-art MDS method in the DUC 2004 and TAC 2011 datasets, both in automatic ROUGE scores and human preference.(1)
更多查看译文
关键词
clustering,proposition-level,multi-document
AI 理解论文
溯源树
样例
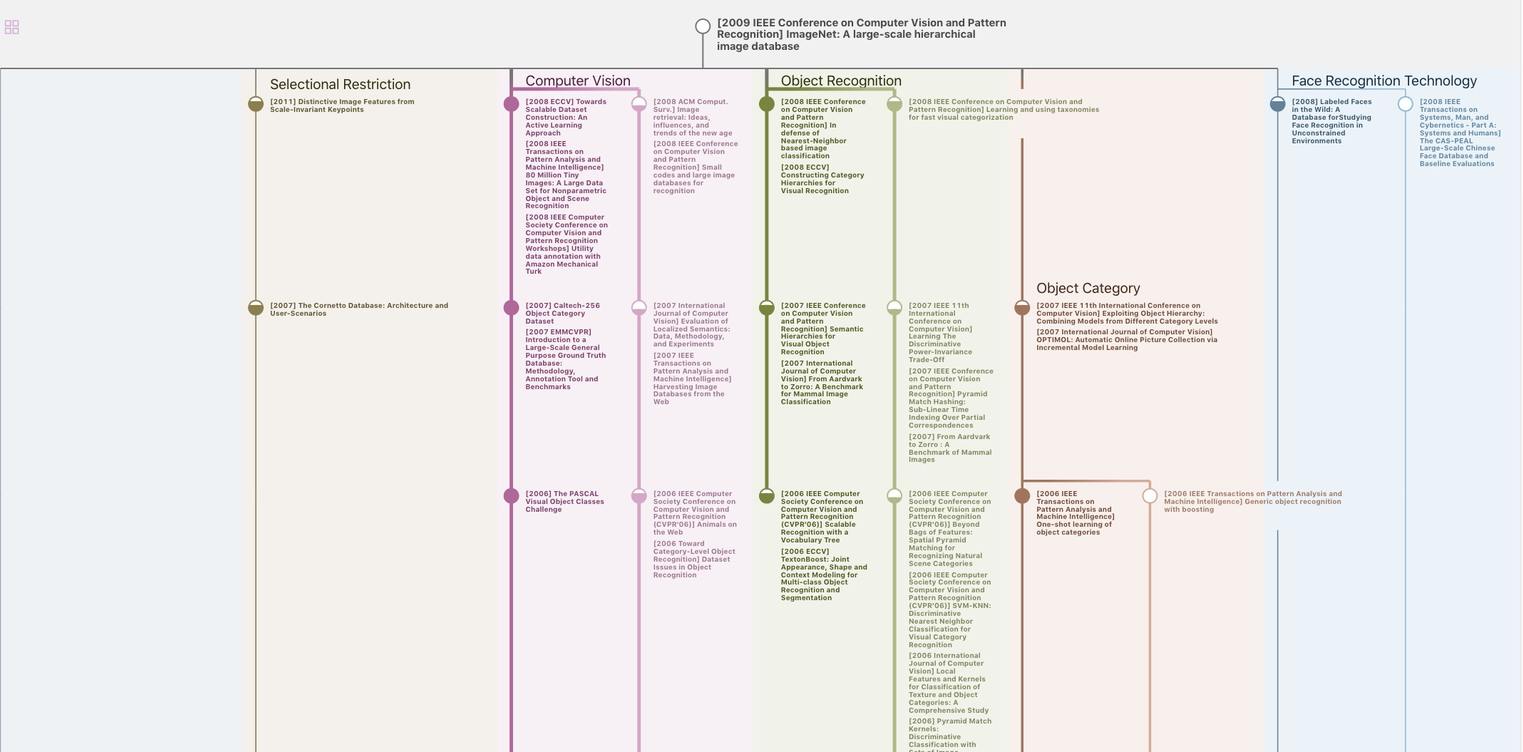
生成溯源树,研究论文发展脉络
Chat Paper
正在生成论文摘要