Hybrid modified marine predators algorithm with teaching-learning-based optimization for global optimization and abrupt motion tracking
Multimedia Tools and Applications(2022)
摘要
Marine predators algorithm (MPA) has solved many challenging optimization problems since proposed. However, corresponding to specific optimization tasks (e.g., visual tracking), it is usually hard to select correct multiple parameters in MPA, which will greatly limit the exploitation and exploration performance. As a result, MPA could be misled to a local minima or even did not converge. To solve this issue, we advise an enhanced version of MPA based on teaching-learning-based optimization (MMPA-TLBO) which can concurrently improve the solution accuracy and the convergence speed. Specifically, first, we propose a modified MPA (MMPA) that leverages chaotic map and opposition-based learning strategy in the initialization stage to generate high-quality individuals. Second, we introduce a parameter-free teaching-learning-based optimization method with strong exploitation operator into MPA, called MMPA-TLBO, which effectively trade-off between the exploitation and exploration procedures. Finally, extensive experiments over 23 benchmark functions, CEC2017 benchmark problems and two engineering design problems show that MMPA-TLBO is better than other algorithms. Furthermore, we perform a thought-provoking case study of MMPA-TLBO on visual tracking. The experimental results show that the MMPA-TLBO tracker can outperform other trackers with a satisfied margin, especially for abrupt motion tracking.
更多查看译文
关键词
Marine predators algorithm,Teaching-learning-based optimization,Benchmark functions,Engineering design problems,Visual tracking
AI 理解论文
溯源树
样例
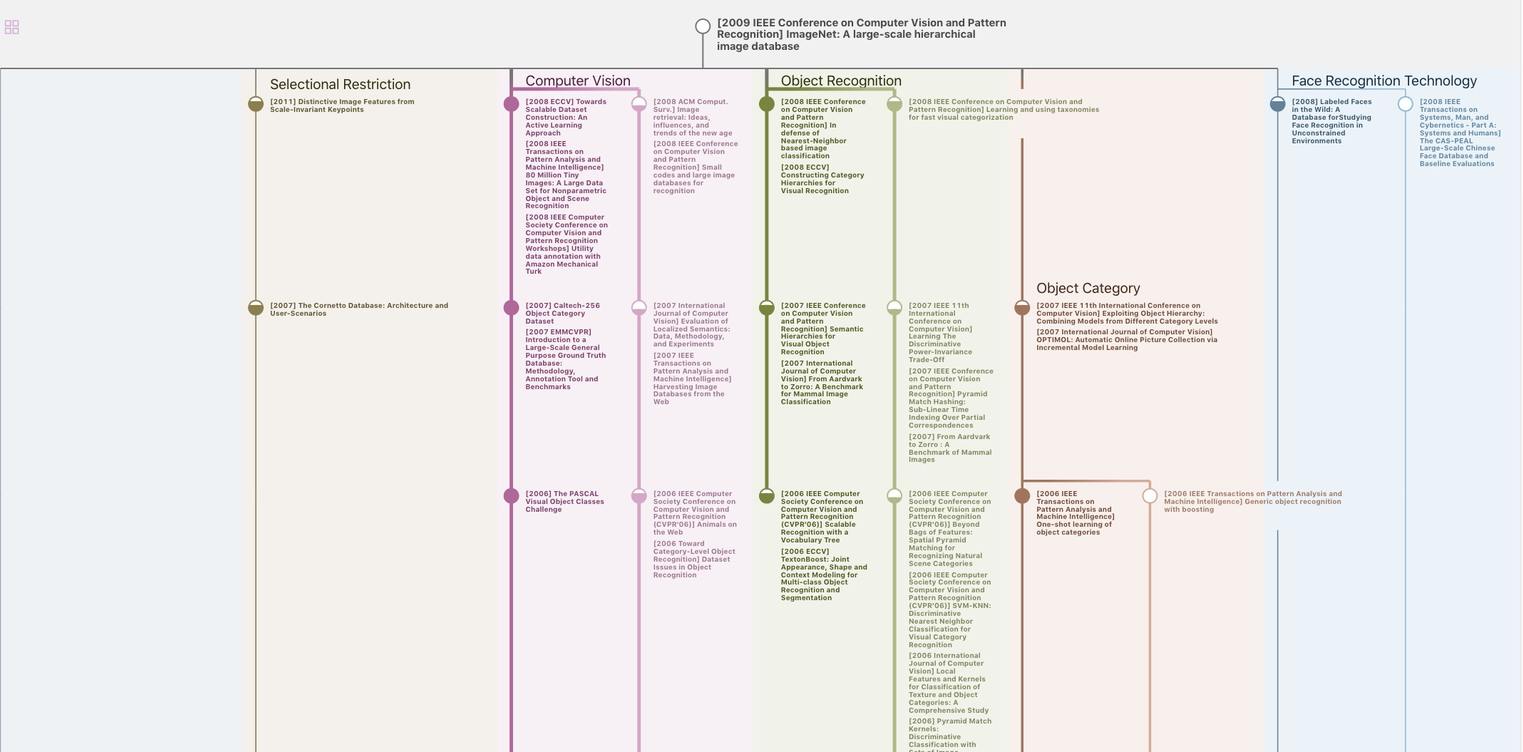
生成溯源树,研究论文发展脉络
Chat Paper
正在生成论文摘要