Testing for ordered alternatives in heteroscedastic ANOVA under normality
Statistical Papers(2023)
摘要
In a one-way design with unknown and unequal error variances, we are interested in testing the null hypothesis H_0:μ _1=μ _2=⋯ =μ _k against the ordered alternative H_1:μ _1≤μ _2≤⋯≤μ _k (with at least one strict inequality). We propose the likelihood ratio test (LRT) as well as two Min-T tests (Min-T and AMin-T) and study their asymptotic behaviour. For better finite sample properties, a parametric bootstrap procedure is used. Asymptotic accuracy of the parametric bootstrap is established. Simulation results show that all the tests control the nominal level in case of small, moderate, and highly unbalanced sample sizes. This even holds for combinations with large variances. However, the asymptotic likelihood ratio test (ALRT) achieves the nominal size only for large samples and k=3 . Power comparisons between LRT and Min-T test show that the LRT has more power than Min-T. However, the Min-T test is easier to use as it does not require evaluation of MLEs. Similar observations are made while comparing ALRT and AMin-T test. The robustness of these tests under departure from normality is also investigated by considering five non-normal distributions (t-distribution, Laplace, exponential, Weibull, and lognormal). When high variances are combined with small or highly unbalanced sample sizes, the tests are observed to be robust only for symmetric distributions. If variances of groups are equal and sample sizes of each group are at least 10, the proposed tests perform satisfactorily for symmetric and skewed distributions. R packages are developed for all tests and a practical example is provided to illustrate their application.
更多查看译文
关键词
ANOVA,Heteroscedasticity,Likelihood ratio test,Parametric bootstrap,Critical points,Robustness
AI 理解论文
溯源树
样例
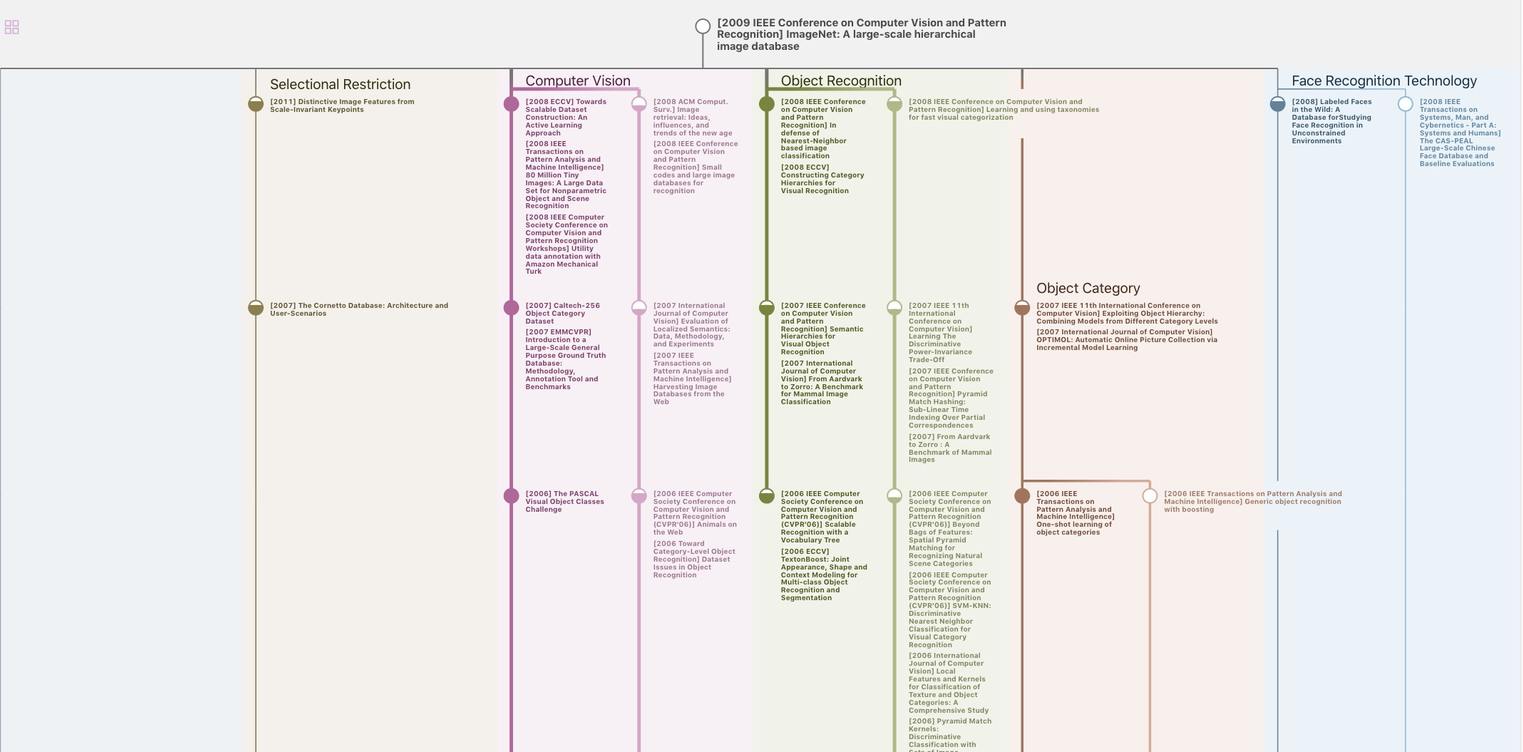
生成溯源树,研究论文发展脉络
Chat Paper
正在生成论文摘要