Semantic similarity information discrimination for video captioning
Expert Systems with Applications(2023)
摘要
Video captioning is a task that aims to automatically describe objects and their actions in videos using natural language sentences. The correct understanding of vision and language information is critical for video captioning tasks. Many existing methods usually fuse different features to generate sentences. However, the sentences have many improper nouns and verbs. Inspired by the successes of fine-grained visual recognition, we treat the problem of improper words to discriminate semantic similarity information. In this paper, we designed a semantic bilinear block (SBB) to widen the gap between the probability of existing and nonexistent words, which can capture more fine-grained features to discriminate semantic information. Moreover, our designed linear attention block (LAB) implements the channelwise attention for the 1-D feature by simplifying the squeeze-and-excitation structure. Furthermore, we designed a semantic discrimination network (SDN) that integrates the LAB and SBB into video encoder and decoder to leverage successful channelwise attention and discriminate semantic similarity information for better video captioning. Experiments on two widely used datasets, MSVD and MSR-VTT, demonstrate that our proposed SDN can achieve better performance than state-of-the-art methods.
更多查看译文
关键词
SDN,CMB,LAB,SBB,S-LSTM
AI 理解论文
溯源树
样例
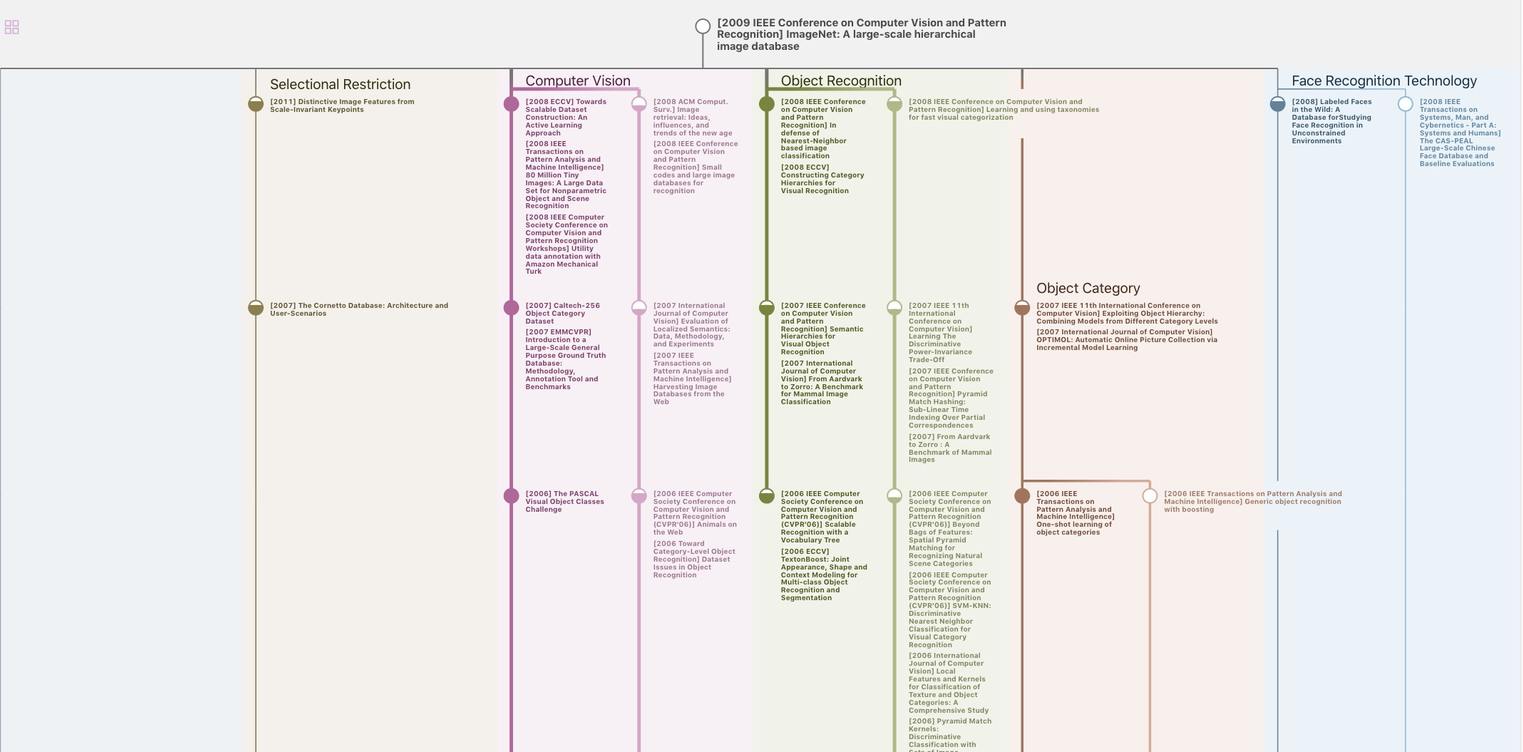
生成溯源树,研究论文发展脉络
Chat Paper
正在生成论文摘要