Solving Full Waveform Inversion Enhanced by Efficient Progressive Transfer Learning
2022 United States National Committee of URSI National Radio Science Meeting (USNC-URSI NRSM)(2022)
摘要
The full waveform inversion (FWI) is highly non-linear and underdetermined. Due to the limitation of acquisition devices, low-frequency information may be missing from obtained data. The absence of low-frequency information could severely compromise FWI results. To solve these problems, we propose a learning-based approach to extrapolate the low-frequency data using a progressive transfer learning workflow to eliminate the labeling requirement for training. The system is implemented on a differentiable program platform to take advantage of automatic differentiation. The performance of the system is validated by an electromagnetic (EM) inverse problem. Our experiment results show that the proposed method is efficient and effective.
更多查看译文
关键词
electromagnetic inversion,finite-difference method,low-frequency extrapolation,transfer learning,deep learning
AI 理解论文
溯源树
样例
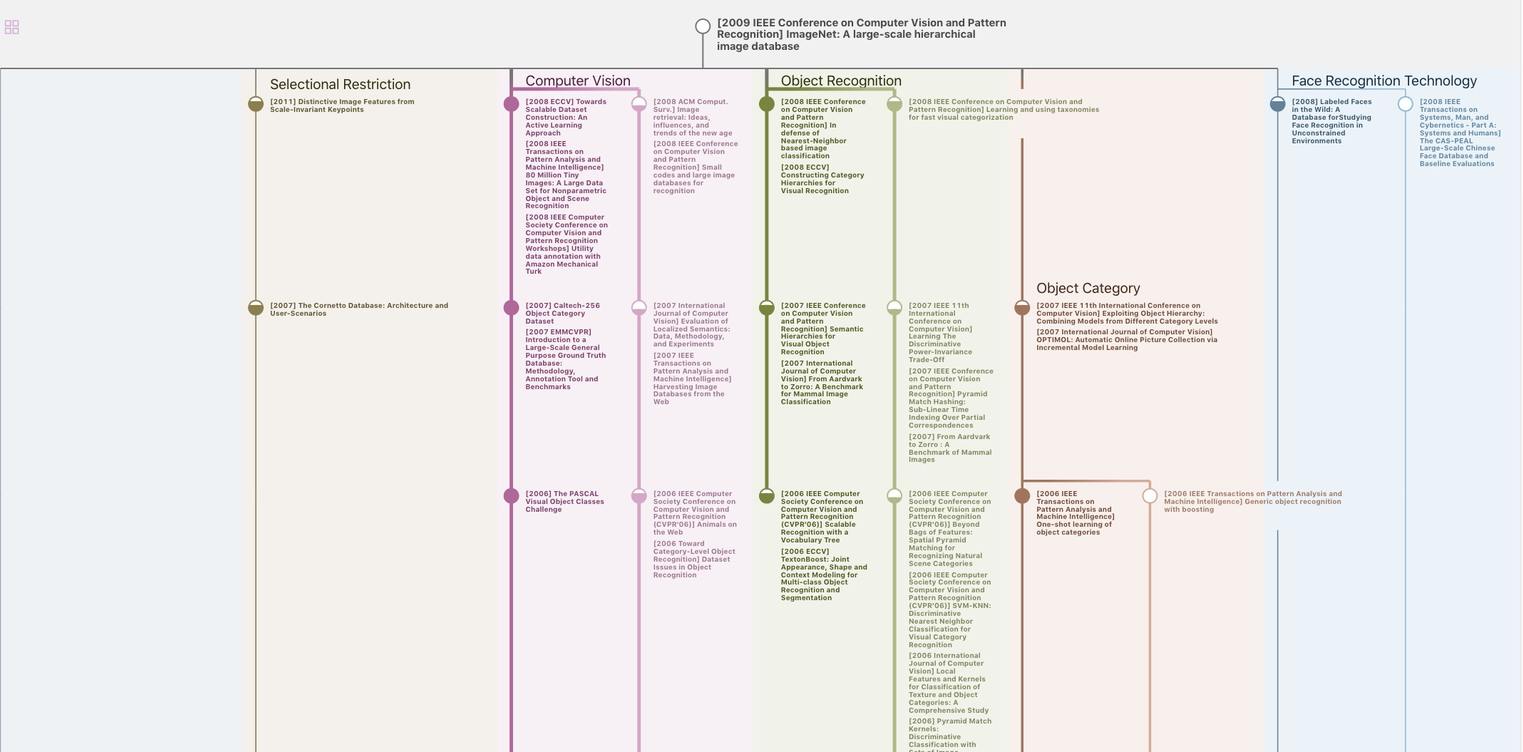
生成溯源树,研究论文发展脉络
Chat Paper
正在生成论文摘要