AI-accelerated materials informatics method for the discovery of ductile alloys
JOURNAL OF MATERIALS RESEARCH(2022)
摘要
In computational materials science, a common means for predicting macroscopic (e.g., mechanical) properties of an alloy is to define a model using combinations of descriptors that depend on some material properties (elastic constants, misfit volumes, etc.), representative for the macroscopic behavior. The material properties are usually computed using special quasi-random structures, in tandem with density functional theory (DFT). However, DFT scales cubically with the number of atoms and is thus impractical for a screening over many alloy compositions. Here, we present a novel methodology which combines modeling approaches and machine-learning interatomic potentials. Machine-learning interatomic potentials are orders of magnitude faster than DFT, while achieving similar accuracy, allowing for a predictive and tractable high-throughput screening over the whole alloy space. The proposed methodology is illustrated by predicting the room temperature ductility of the medium-entropy alloy Mo–Nb–Ta. Graphical abstract
更多查看译文
关键词
Materials informatics,Moment tensor potential,Active learning,Random alloy,Ductility,Average-atom potential
AI 理解论文
溯源树
样例
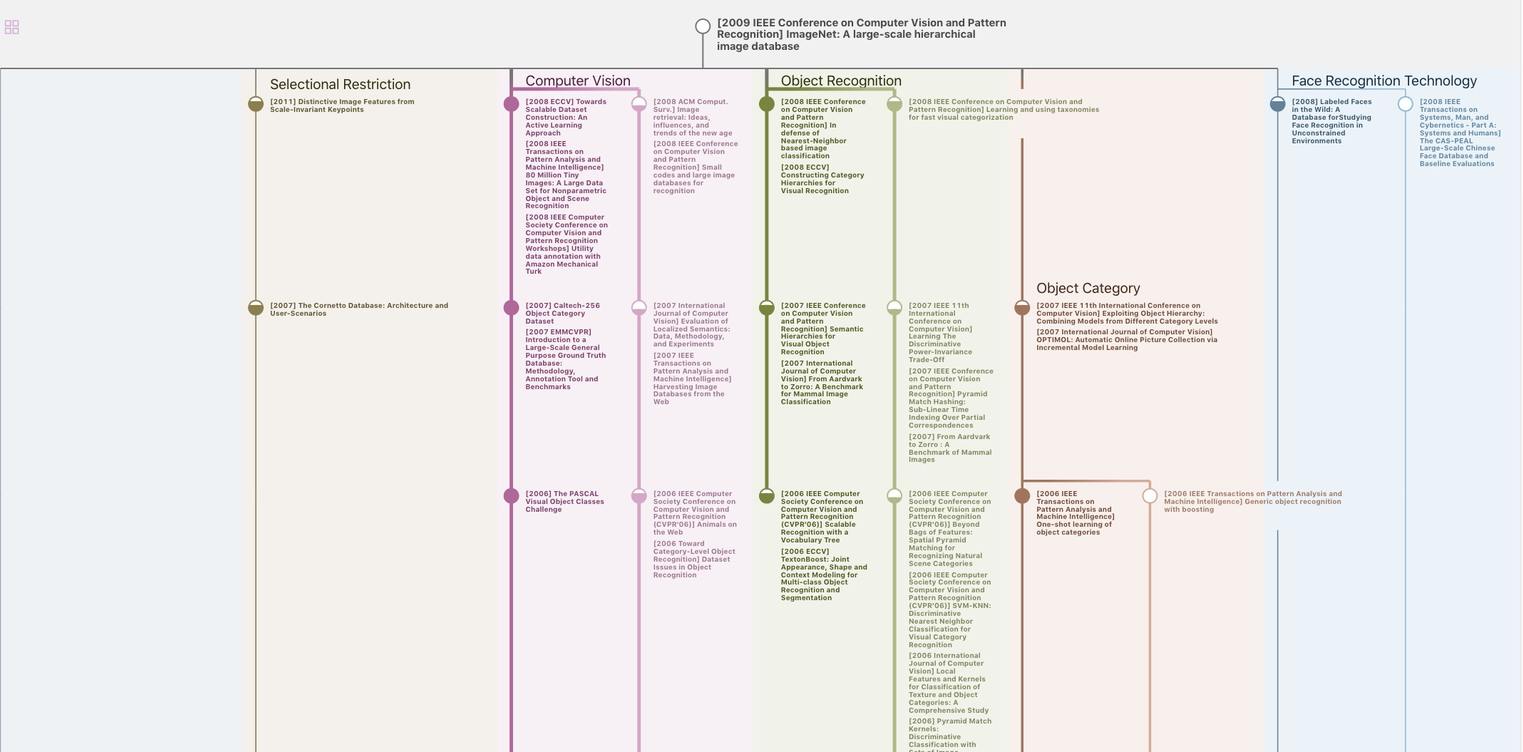
生成溯源树,研究论文发展脉络
Chat Paper
正在生成论文摘要