Broad Minimax Probability Learning System and its Application in Regression Modeling
IEEE Transactions on Systems, Man, and Cybernetics: Systems(2023)
摘要
Broad learning system (BLS), a novel incremental learning algorithm, has attracted more and more attention and application. BLS achieves a perfect balance between learning performance and modeling efficiency based on the mean square error (MSE) criterion. However, MSE assumes that a random error between an observed value and real value is a Gaussian distribution, which may be inconsistent to the real-world situation. Moreover, the distributional assumptions may cast doubt on the generalization and validity of the built BLS model. In this article, we propose a novel distribution-free BLS for regression analysis, called broad minimax probability learning system (BMPLS). Learning from the idea of minimax probability machine regression, BMPLS builds a regression model by maximizing the worst probability of the regression function being within the allowed error range. It adequately utilizes the mean and covariance information of the hidden-space feature without making any distributional assumptions of the random error. In order to further improve the learning performance of the model, a regularized BMPLS (RBMPLS) is proposed by applying elastic-net regularization term to BMPLS. The corresponding optimization algorithm is also designed for calculating the output weights of the model. Multiple experiments based on public datasets were carried out to demonstrate the feasibility of the proposed algorithms.
更多查看译文
关键词
Broad learning system (BLS),distribution-free,elastic-net,minimax probability machine (MPM) regression,regression modeling
AI 理解论文
溯源树
样例
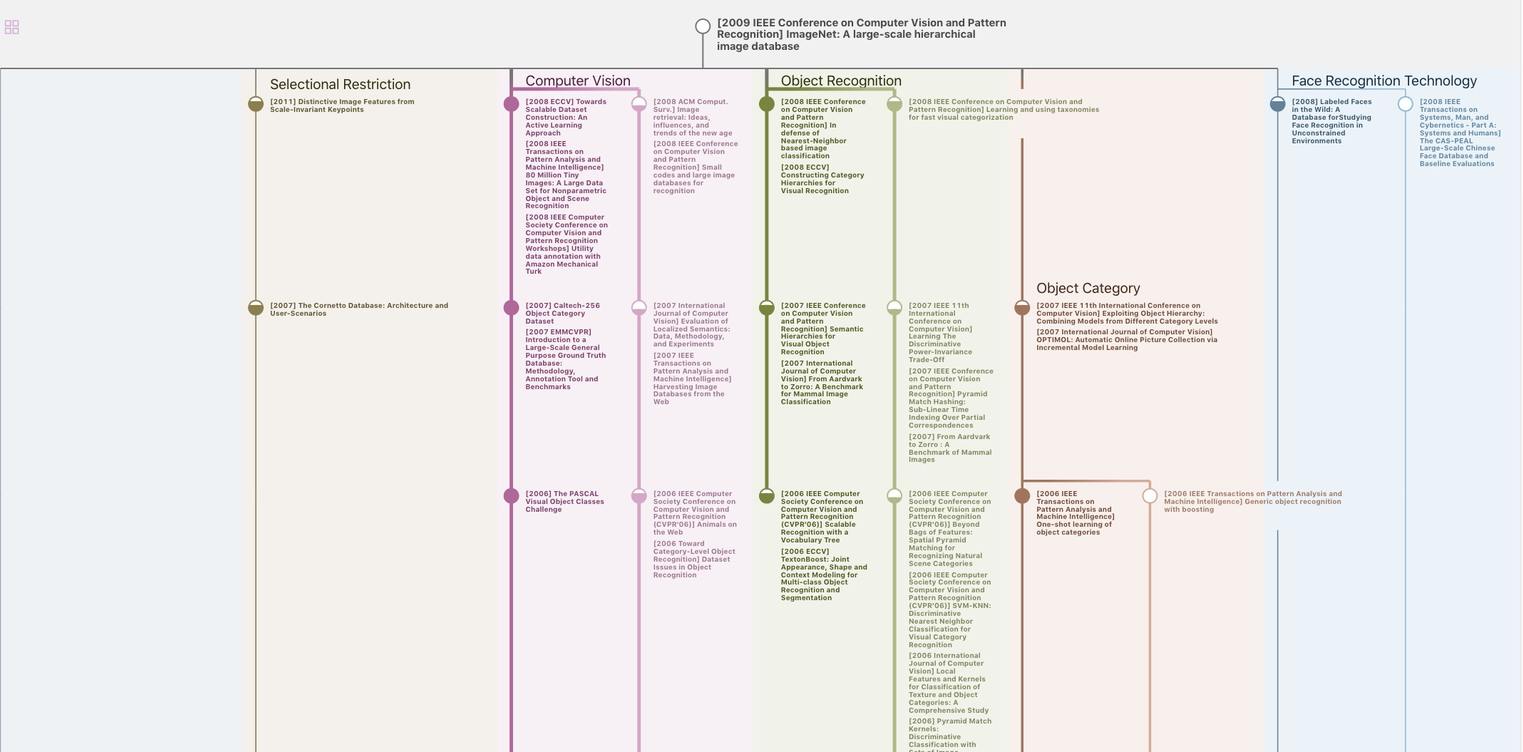
生成溯源树,研究论文发展脉络
Chat Paper
正在生成论文摘要