A Stream Learning Approach for Real-Time Identification of False Data Injection Attacks in Cyber-Physical Power Systems.
IEEE Trans. Inf. Forensics Secur.(2022)
摘要
This paper presents a novel data-driven framework to aid in system state estimation when the power system is under unobservable false data injection attacks. The proposed framework dynamically detects and classifies false data injection attacks. Then, it retrieves the control signal using the acquired information. This process is accomplished in three main modules, with novel designs, for detection, classification, and control signal retrieval. The detection module monitors historical changes in phasor measurements and captures any deviation pattern caused by an attack on a complex plane. This approach can help to reveal characteristics of the attacks including the direction, magnitude, and ratio of the injected false data. Using this information, the signal retrieval module can easily recover the original control signal and remove the injected false data. Further information regarding the attack type can be obtained through the classifier module. The proposed ensemble learner is compatible with harsh learning conditions including the lack of labeled data, concept drift, concept evolution, recurring classes, and independence from external updates. The proposed novel classifier can dynamically learn from data and classify attacks under all these harsh learning conditions. The introduced framework is evaluated w.r.t. real-world data captured from the Central New York Power System. The obtained results indicate the efficacy and stability of the proposed framework.
更多查看译文
关键词
Attack identification,false data injection,unobservable attacks,power systems,cyber-physical systems,statistical learning,change detection,non-stationary environments
AI 理解论文
溯源树
样例
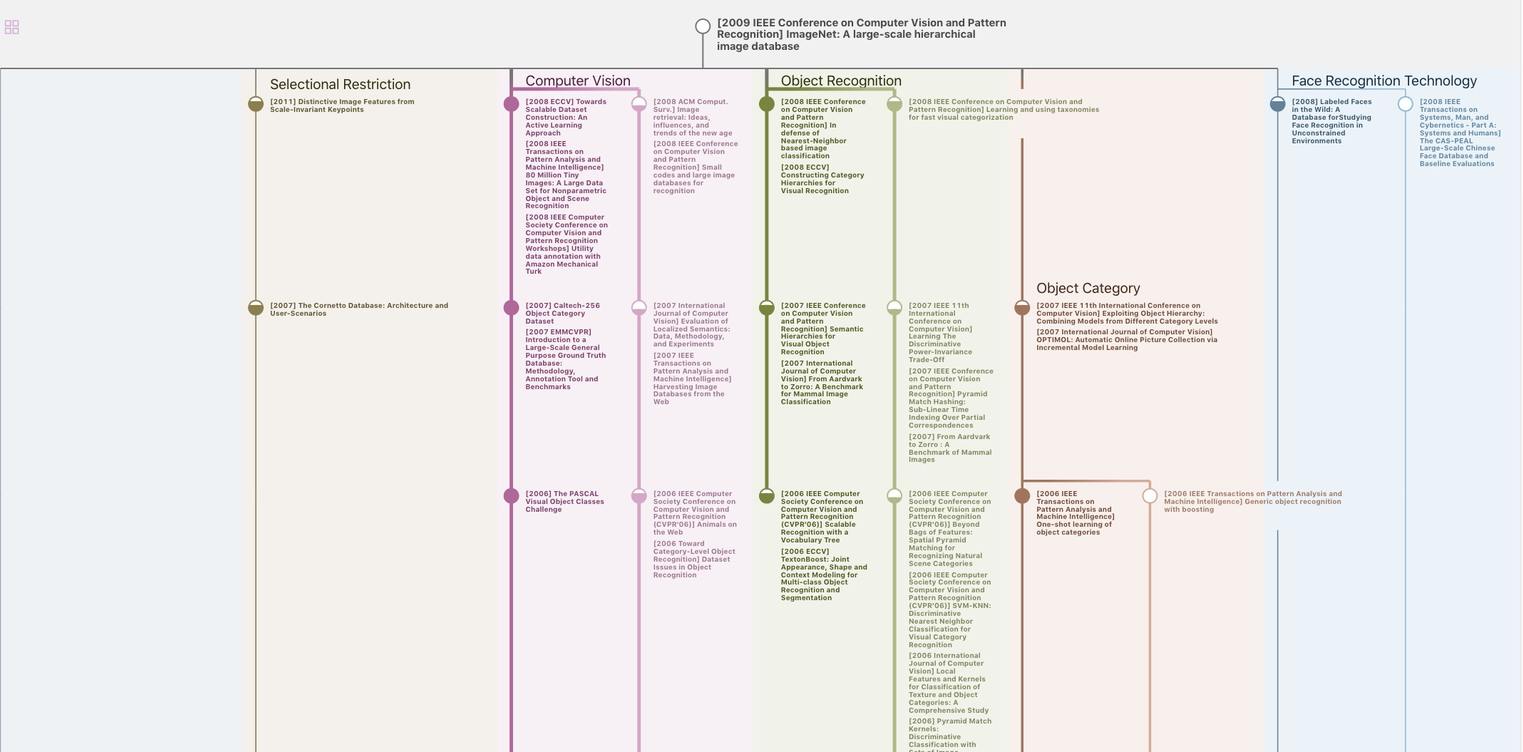
生成溯源树,研究论文发展脉络
Chat Paper
正在生成论文摘要