PRM: An Efficient Partial Recovery Method to Accelerate Training Data Reconstruction for Distributed Deep Learning Applications in Cloud Storage Systems
2022 IEEE/ACM 30th International Symposium on Quality of Service (IWQoS)(2022)
摘要
Distributed deep learning is a typical machine learning method running in distributed environment such as cloud computing systems. The corresponding training, validation and test datasets are very large in general (e.g., several TBs), which need to be stored across multiple data nodes. Due to the high disk failure ratio in cloud storage systems, one of the critical issues for distributed deep learning is how to efficiently tolerate disk failures in the training procedures. These failures can lead to a large amount of data loss, which decreases the training accuracy and slows down the training process. Although several recovery methods are proposed to accelerate the data reconstruction, the related overhead is extremely high, such as high CPU/GPU utilization, a large number of I/Os, etc.To address the above problems, we propose a novel Partial-Recovery Method (called PRM) , which is an adaptive recovery method to accelerate data reconstruction for distributed deep learning applications in cloud storage systems. The key idea of PRM is combining the advantages of erasure coding’s ability to obtain global information on the data distribution with the AI’s ability to recover partial lost data, which can sharply reduce the overhead with acceptable training accuracy. To demonstrate the effectiveness of the PRM approach, we conduct several experiments. The results show that, compared to the state-of-the-art full or approximate recovery methods, PRM decreases the average network transmission time overhead by up to 64.50%, and reduces the recovery time by up to 55.90%, respectively.
更多查看译文
关键词
Distributed Deep Learning,Cloud Storage Systems,Erasure Coding,Partial Recovery,Data Reconstruction
AI 理解论文
溯源树
样例
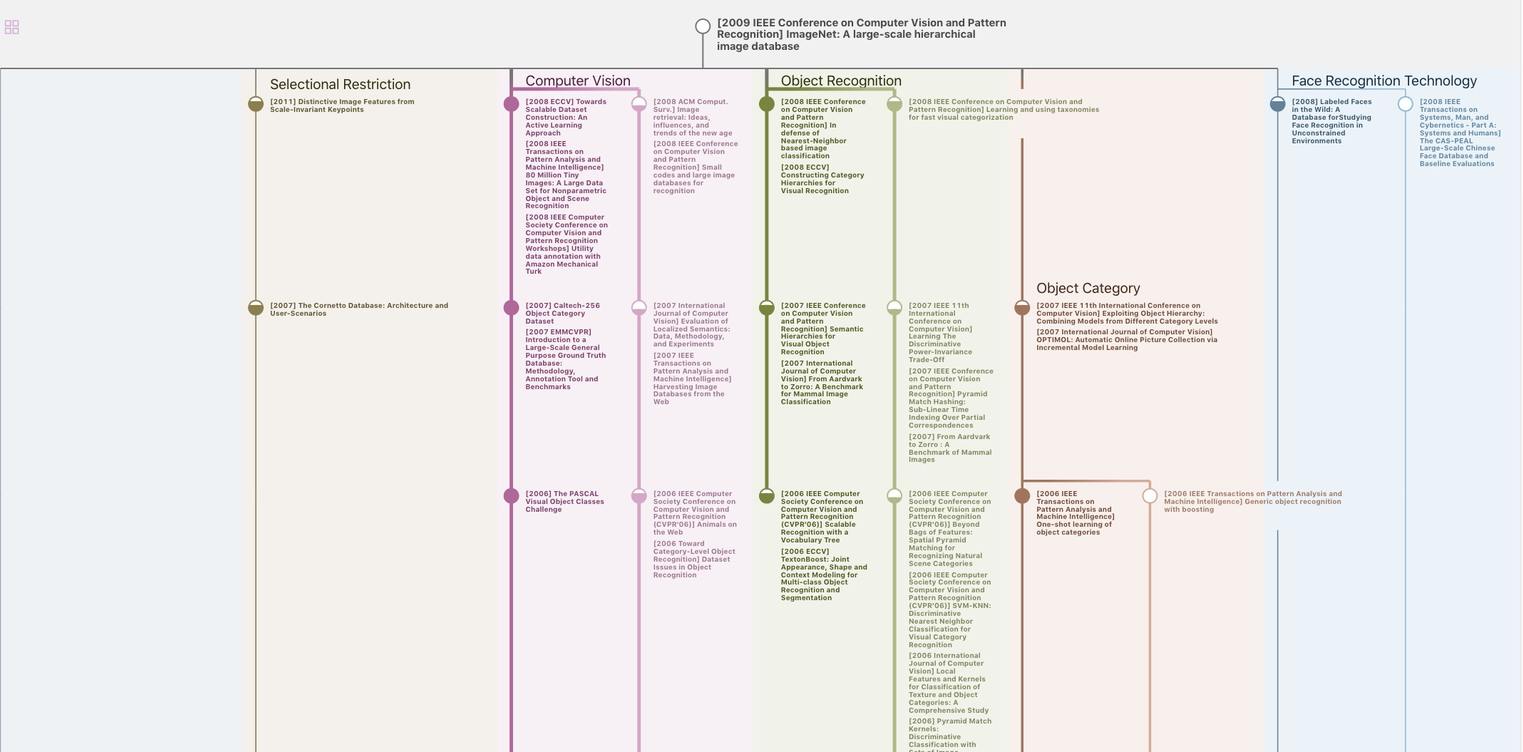
生成溯源树,研究论文发展脉络
Chat Paper
正在生成论文摘要