iSwift: Fast and Accurate Impact Identification for Large-scale CDNs
2022 IEEE/ACM 30th International Symposium on Quality of Service (IWQoS)(2022)
摘要
One key challenge to maintain a large-scale Content Delivery Network (CDN) is to minimize the service downtime when severe system problems happen (e.g., hardware failures). In this case, a critical step is to quickly and accurately identify the range of users with performance degradation, termed impact identification. Successful impact identification not only helps identify impacted users but also provides meaningful information for troubleshooting. However, current practice of impact identification usually takes network engineers several hours to manually identify impacted users, which may lead to a huge business loss. The main challenges for automatic impact identification in large CDNs include the inaccuracy of underlying anomaly detection, huge search space of impact identification and severe long-tail distribution of user traffic. In this paper we propose iSwift, a system that is specifically designed for impact identification in large-scale CDNs in order to address aforementioned challenges. We evaluate the performance of iSwift on semi-synthetic datasets and the results show that iSwift can achieve a F1-score greater than 0.85 within ten seconds, which significantly outperforms state-of-the-art solutions. Furthermore, iSwift has been deployed in a production CDN around one year as a pilot project and demonstrated its online performance confirmed by the network operators.
更多查看译文
关键词
impact identification,failure diagnosis,CDN
AI 理解论文
溯源树
样例
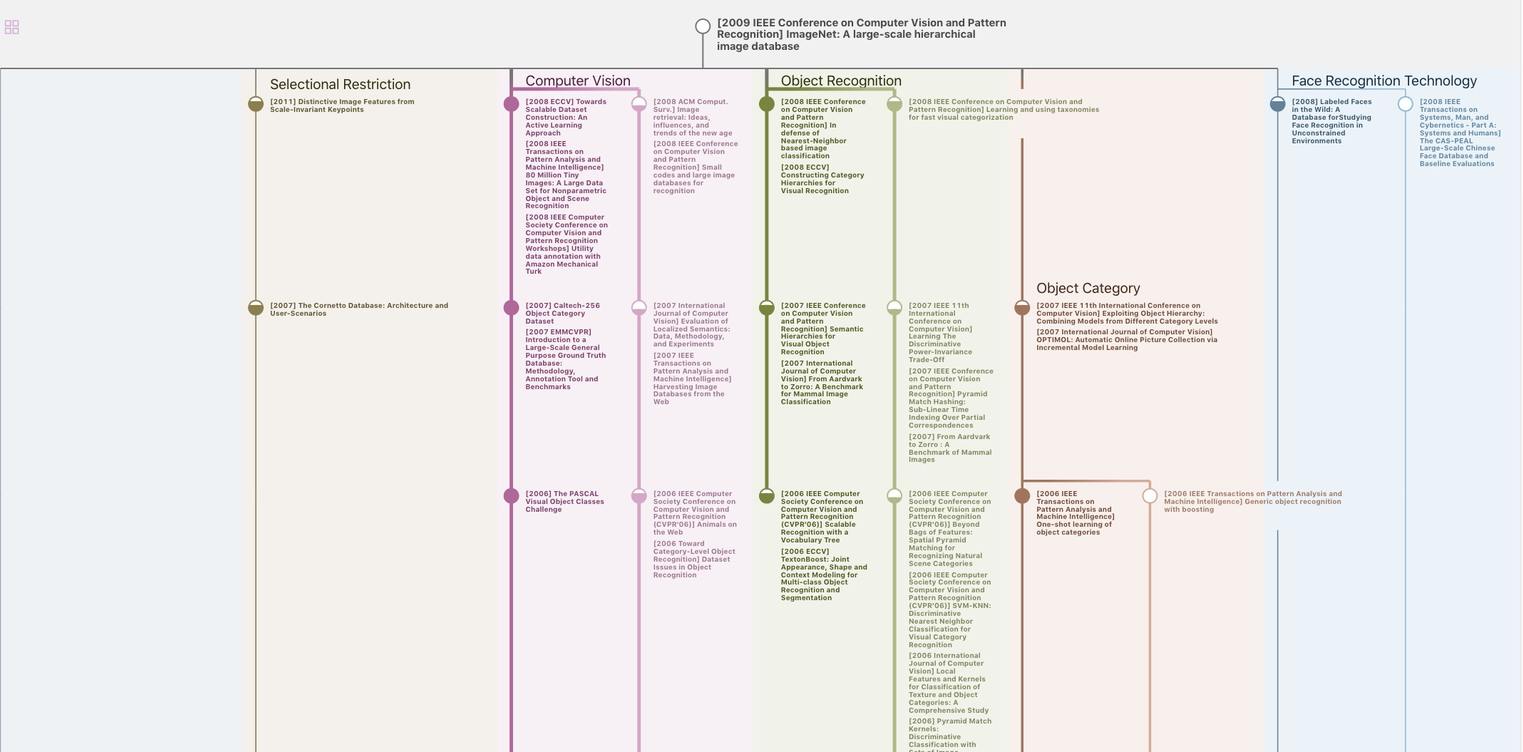
生成溯源树,研究论文发展脉络
Chat Paper
正在生成论文摘要