A Control-Theoretic and Online Learning Approach to Self-Tuning Queue Management
2022 IEEE/ACM 30th International Symposium on Quality of Service (IWQoS)(2022)
摘要
There is a growing trend that network applications not only require higher throughput, but also impose stricter delay requirements. The current Internet congestion control, which is driven by active queue management (AQM) algorithms interacting with the Transmission Control Protocol (TCP), has been playing an important role in supporting network applications. However, it still exhibits many open issues. Most of AQM algorithms only deploy a single-queue structure that cannot differentiate flows and easily leads to unfairness. Moreover, the parameter settings of AQM are often static, making them difficult to adapt to the dynamic network environments. In this paper, we propose a general framework for designing "self-tuning" queue management (SQM), which is adaptive to the changing environments and provides fair congestion control among flows. We first present a general architecture of SQM with fair queueing and propose a general fluid model to analyze it. To adapt to the stochastic environments, we formulate a stochastic network utility maximization (SNUM) problem, and utilize online convex optimization (OCO) and control theory to develop a distributed SQM algorithm which can self-tune different queue weights and control parameters. Numerical and packet-level simulation results show that our SQM algorithm significantly improves queueing delay and fairness among flows.
更多查看译文
关键词
online learning approach,self-tuning queue management,network applications,stricter delay requirements,current Internet congestion control,active queue management algorithms,Transmission Control Protocol,AQM algorithms,single-queue structure,dynamic network environments,fair congestion control,general architecture,fair queueing,general fluid model,stochastic environments,stochastic network utility maximization problem,control theory,distributed SQM algorithm,queue weights,control parameters,control-theoretic approach
AI 理解论文
溯源树
样例
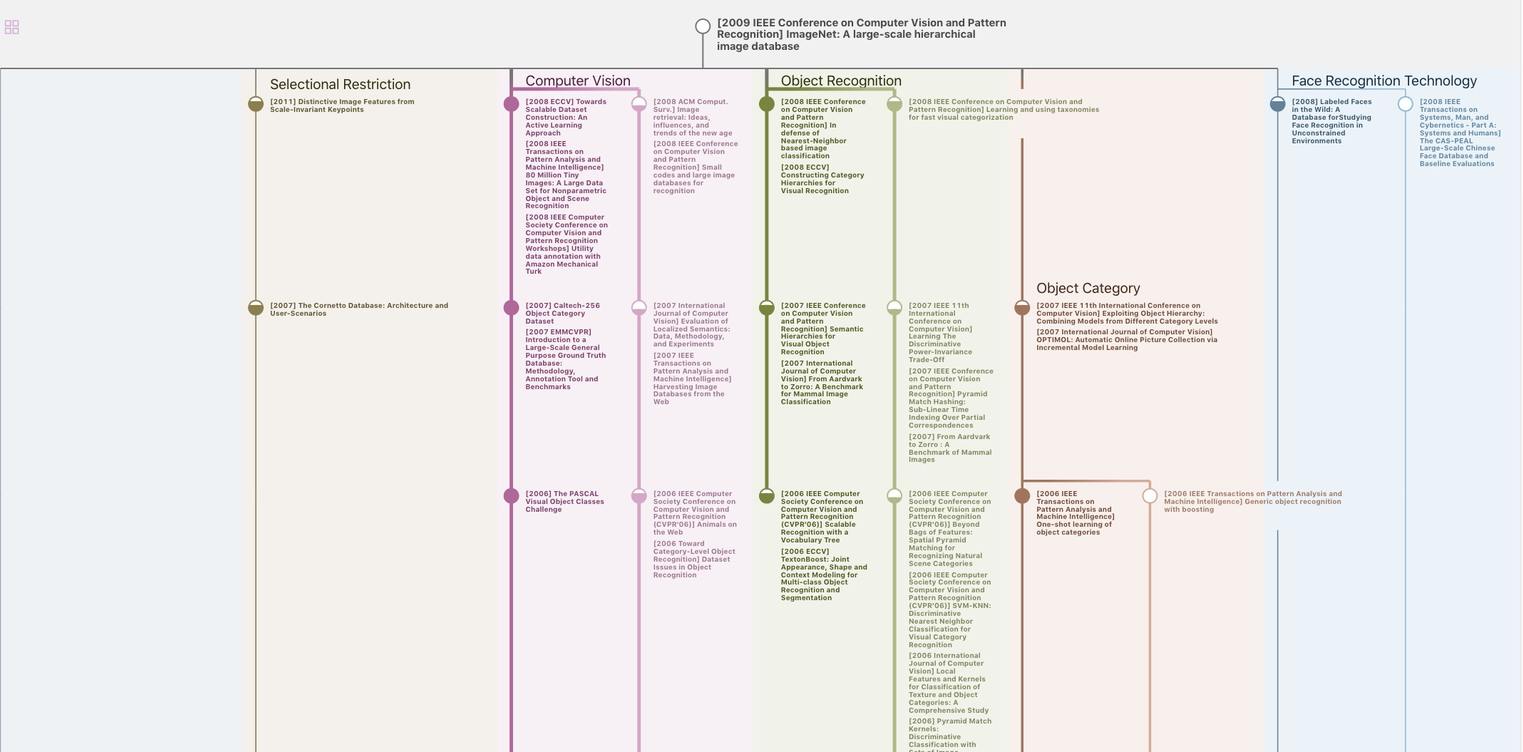
生成溯源树,研究论文发展脉络
Chat Paper
正在生成论文摘要