A Novel Multiview Sampling-Based Meta Self-Paced Learning Approach for Class-Imbalanced Intelligent Fault Diagnosis
IEEE TRANSACTIONS ON INSTRUMENTATION AND MEASUREMENT(2022)
摘要
In practical machine fault diagnosis, the obtained data samples under faulty conditions are usually far less than those under normal conditions, resulting in a class-imbalanced dataset issue. The existing solutions for class-imbalanced scenarios include data-level and model-level strategies, which are either subject to overgeneralization or time-consuming. To address it, this article proposes a novel multiview sampling-based meta self-spaced learning approach. First, the signal processing methods, such as time-domain (TD), frequency-domain (FD), and time-frequency domain (TFD), are used to extract statistical features from the original data to form diverse views. Next, the meta self-paced learning technology is applied to select high-quality samples from multiview feature data to generate a class-balanced dataset. Finally, a fault diagnosis model is trained with the obtained class-balanced dataset. The main contribution of this research has twofold: 1) the introduced multiview sampling method adaptively learns the weight in the sampling process and automatically deletes the noise samples with large loss value to improve the performance of the fault diagnosis model; and 2) the proposed meta self-spaced learning approach eliminates the error caused by setting parameters manually and ensures the quality of the extracted samples. To validate its performance, a comparative study is conducted on a public dataset and the one collected from an industrial motor test platform. Five baseline methods are compared with the proposed one based on the convolutional neural network (CNN) model. Moreover, three traditional machine learning models are to verify the sample quality generated. The experimental results achieve above 90% diagnosis accuracy, which provides a new intelligent manner for the modular service application of class-imbalance fault diagnosis.
更多查看译文
关键词
Fault diagnosis,Training,Adaptation models,Support vector machines,Generative adversarial networks,Feature extraction,Biological system modeling,Class-imbalanced data,fault diagnosis,meta self-paced learning,multiview sampling,self-paced learning
AI 理解论文
溯源树
样例
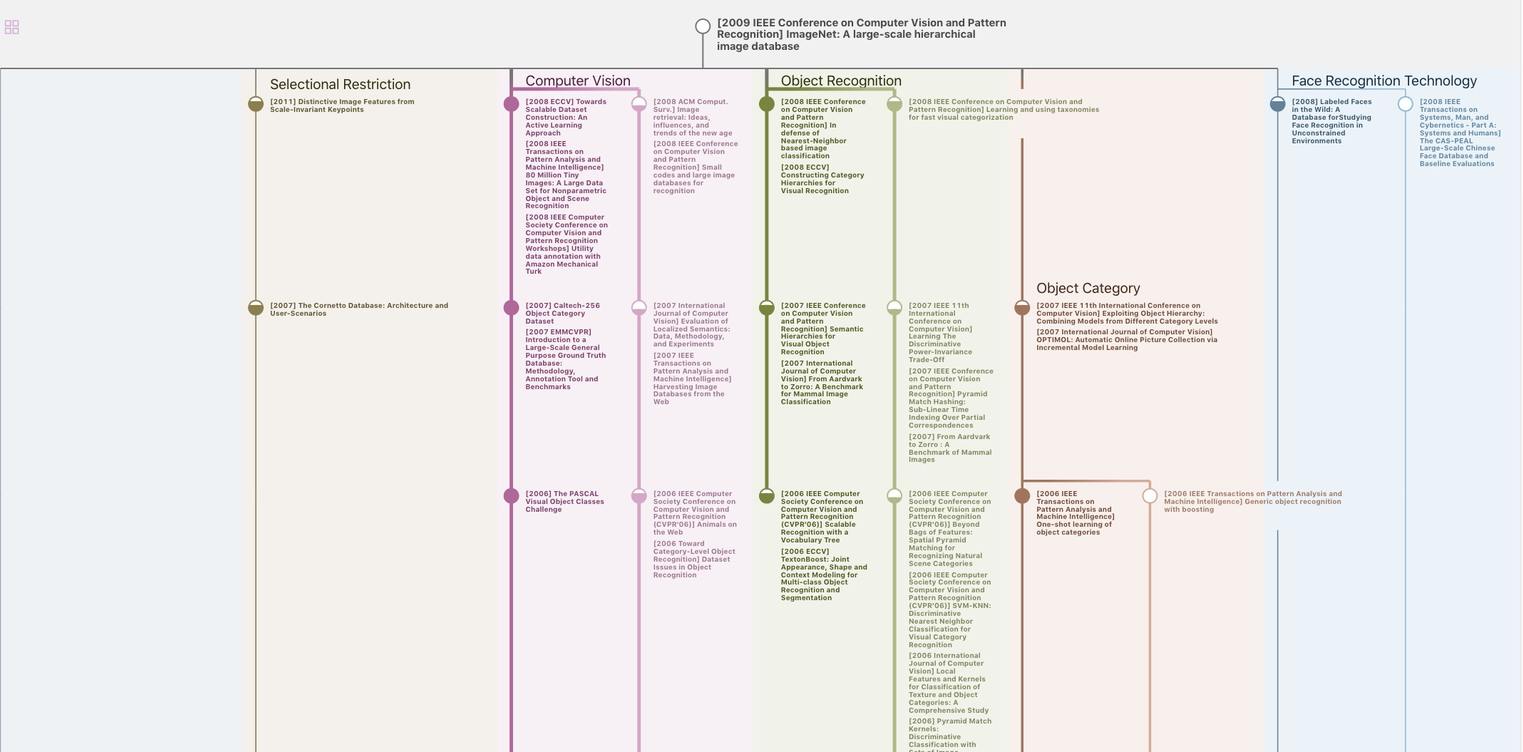
生成溯源树,研究论文发展脉络
Chat Paper
正在生成论文摘要