Accelerated Machine Learning for On-Device Hardware-Assisted Cybersecurity in Edge Platforms
2022 23rd International Symposium on Quality Electronic Design (ISQED)(2022)
摘要
Internet of Things (IoT) as an area of tremendous impact, potential, and growth has emerged with the advent of smart homes, smart cities, and smart everything. In the age of IoT, edge devices have taken on greater importance, driving the need for more intelligence and advanced services at the network edge. Since edge devices are limited in compute, storage, and network capacity, they are easy to compromise, more exposed to attackers, and more vulnerable than standard computing systems such as PCs and servers. While several software-based countermeasures have been proposed, they require high computing power and resources that edge devices do not possess. Moreover, modern threats have become more complex and severe, requiring a level of security that can only be provided at the hardware level. In this paper, we realize an efficient hardware-assisted attack detection mechanism for edge devices through effective High Level Synthesize (HLS) optimization techniques. To this end, we propose OptiEdge, a machine learning-guided hardware-assisted resource and timing estimation tool that can effectively reduce the design space exploration for edge devices’ design. This is achieved by analyzing suitability of different machine learning algorithms used for detection and how they affect hardware implementation overheads. By providing a comprehensive analysis of the accuracy, performance, and hardware efficiency of different ML algorithms, our work can assist researchers investigating this field of cybersecurity.
更多查看译文
关键词
Machine Learning,Hardware Acceleration,Hardware-Assisted Security,Malware Detection
AI 理解论文
溯源树
样例
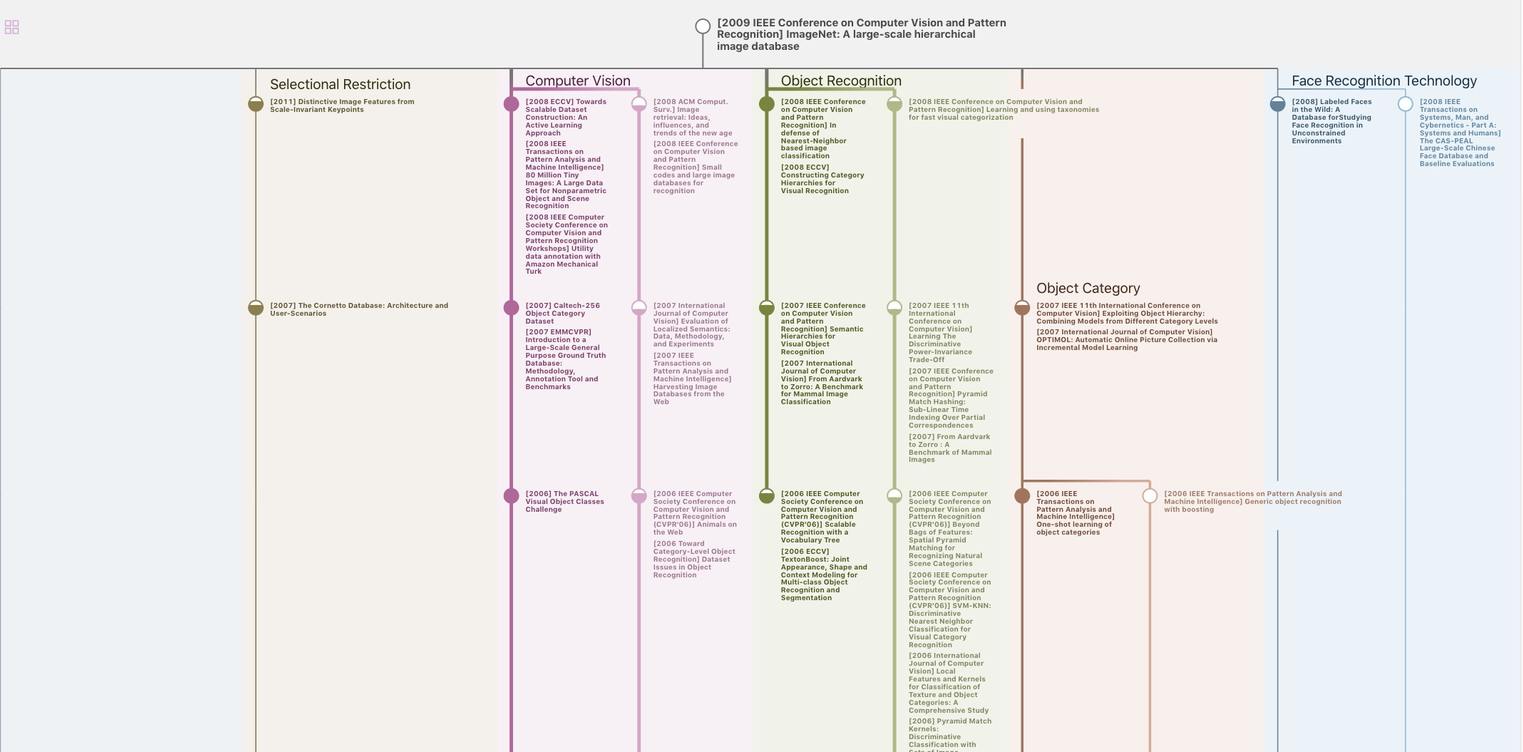
生成溯源树,研究论文发展脉络
Chat Paper
正在生成论文摘要