Pixel-Level and Feature-Level Domain Adaptation for Heterogeneous SAR Target Recognition
IEEE GEOSCIENCE AND REMOTE SENSING LETTERS(2022)
摘要
The performance of synthetic aperture radar (SAR) target recognition has been substantially enhanced by deep learning technology. It is still difficult to get strong recognition performance due to the distribution differences across heterogeneous SAR images. To enhance target recognition performance in heterogeneous SAR situations, a pixel-level and feature-level domain adaptation (PFDA) approach is proposed in this letter to deal with this problem. The pixel-level translation module in the first step generates images with high visual similarity with other distributed images from one distributed image. The second step involves introducing feature alignment into the network to lower the probability distribution divergence of heterologous SAR images in the feature space and enhance recognition performance. We evaluated our method on the synthetic and measured paired labeled experiment (SAMPLE) dataset and a self-built airplane dataset to ensure its effectiveness. Compared to existing domain adaption approaches, experimental results reveal that our strategy greatly improves recognition performance and model stability for heterogeneous SAR target recognition.
更多查看译文
关键词
Feature extraction,Target recognition,Radar polarimetry,Synthetic aperture radar,Training,Adaptation models,Image recognition,Heterogeneous Synthetic aperture radar (SAR) image,SAR,target recognition,unsupervised domain adaptation
AI 理解论文
溯源树
样例
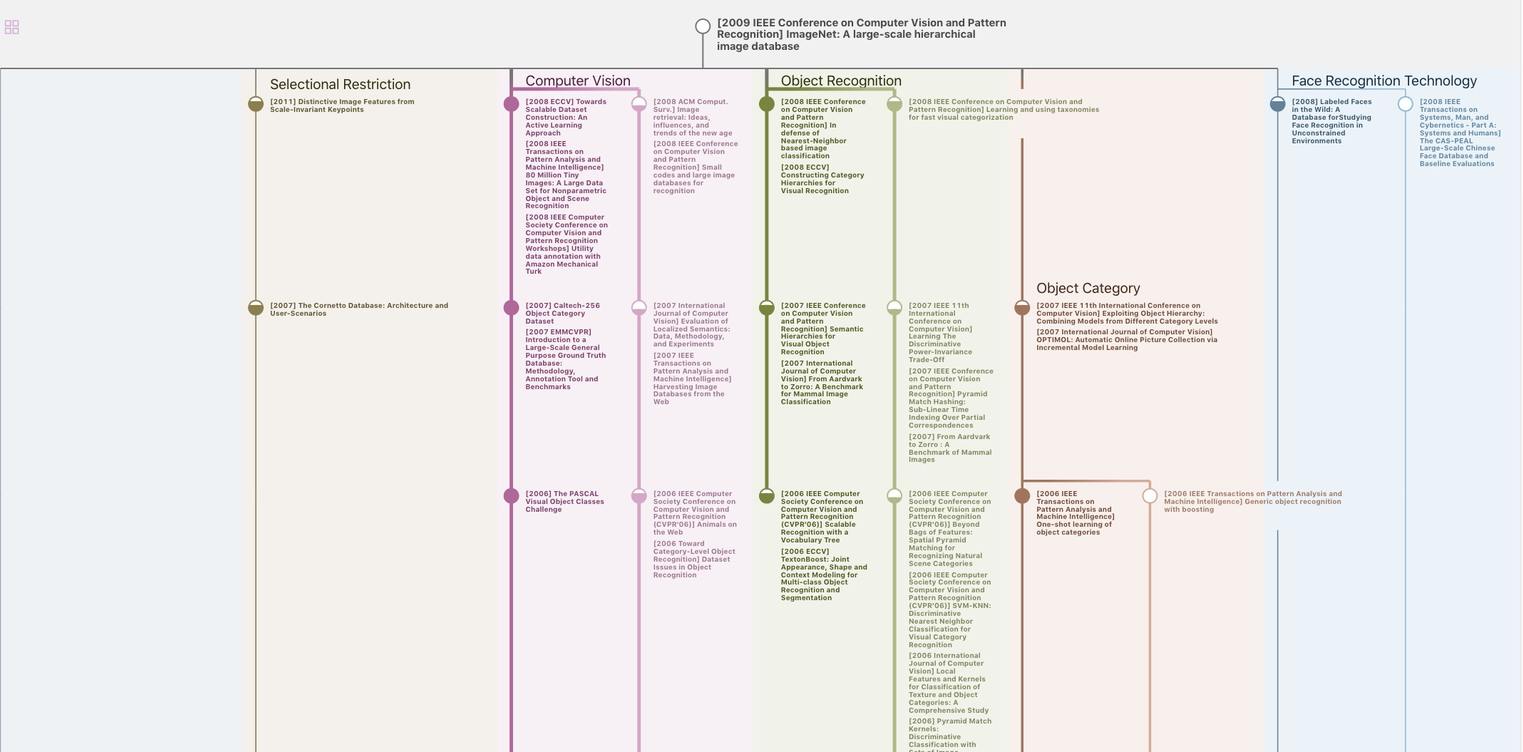
生成溯源树,研究论文发展脉络
Chat Paper
正在生成论文摘要